Polar Occupancy Map - A Compact Traffic Representation For Deep Learning Scenario Classification
2019 IEEE INTELLIGENT TRANSPORTATION SYSTEMS CONFERENCE (ITSC)(2019)
摘要
In order to accelerate development, testing and verification of automated vehicles, it is crucial to classify a wide range of driving scenarios. Scenario classification is usually done by rule-based algorithms or even manual video or signal inspection. A promising alternative is to use machine learning and let neural networks extract the relevant classification features. Since inputs to neural networks need to have a fixed size, an abstract representation of the driving scenario is necessary. In this paper, a scenario representation that captures the dynamic traffic behavior, without loss of relevant information, is presented.
更多查看译文
关键词
compact traffic representation,deep learning,automated vehicles,driving scenario,rule-based algorithms,machine learning,neural networks,abstract representation,classification feature extraction,scenario representation,dynamic traffic behavior,polar occupancy map,scenario classification
AI 理解论文
溯源树
样例
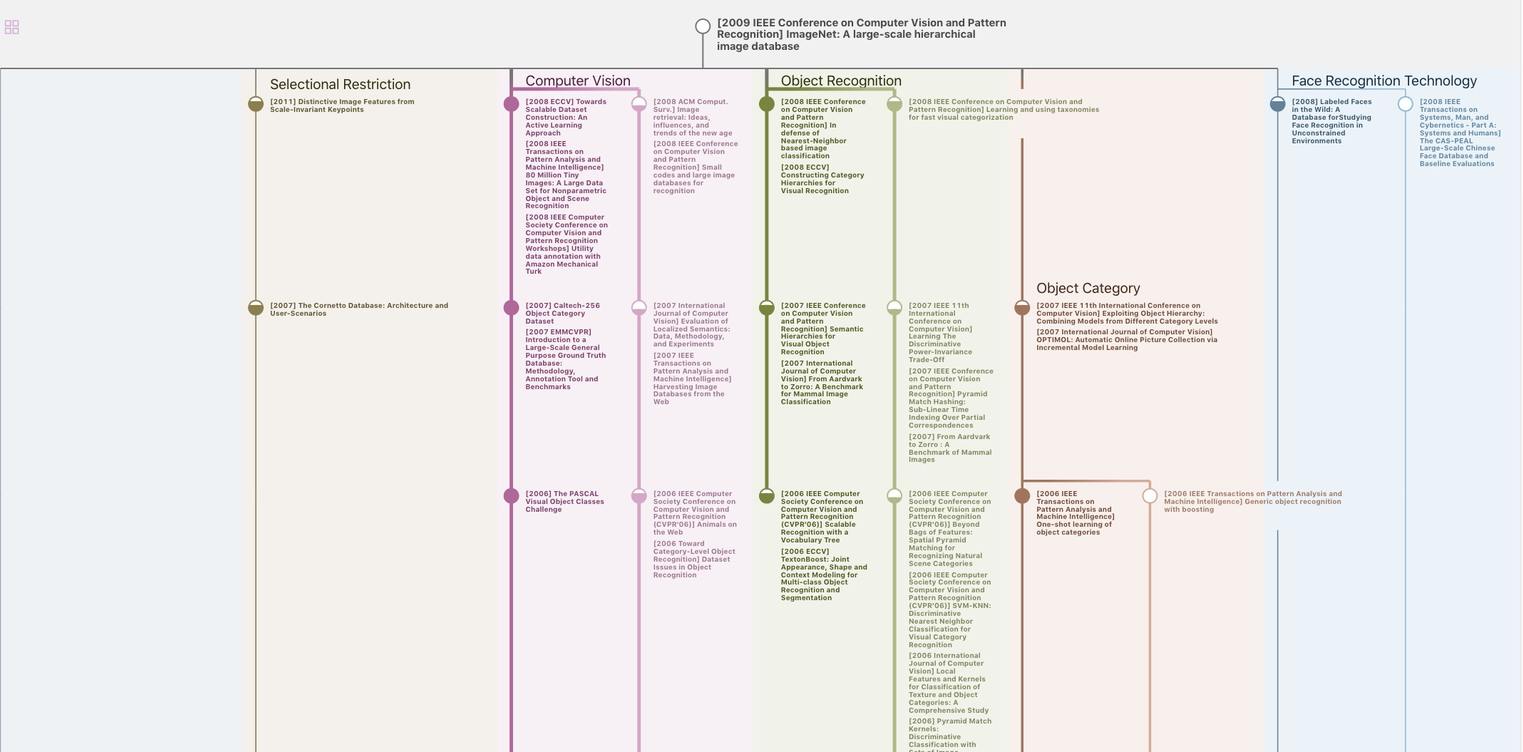
生成溯源树,研究论文发展脉络
Chat Paper
正在生成论文摘要