DeepDeform: Learning Non-Rigid RGB-D Reconstruction With Semi-Supervised Data
2020 IEEE/CVF CONFERENCE ON COMPUTER VISION AND PATTERN RECOGNITION (CVPR)(2020)
摘要
Applying data-driven approaches to non-rigid 3D reconstruction has been difficult, which we believe can be attributed to the lack of a large-scale training corpus. Unfortunately, this method fails for important cases such as highly non-rigid deformations. We first address this problem of lack of data by introducing a novel semi-supervised strategy to obtain dense inter-frame correspondences from a sparse set of annotations. This way, we obtain a large dataset of 400 scenes, over 390,000 RGB-D frames, and 5,533 densely aligned frame pairs; in addition, we provide a test set along with several metrics for evaluation. Based on this corpus, we introduce a data-driven non-rigid feature matching approach, which we integrate into an optimization-based reconstruction pipeline. Here, we propose a new neural network that operates on RGB-D frames, while maintaining robustness under large non-rigid deformations and producing accurate predictions. Our approach significantly outperforms existing non-rigid reconstruction methods that do not use learned data terms, as well as learning-based approaches that only use self-supervision.
更多查看译文
关键词
data-driven nonrigid feature matching,inter-frame correspondences,DeepDeform,optimization-based reconstruction pipeline,nonrigid 3D reconstruction,semisupervised data,nonrigid RGB-D reconstruction
AI 理解论文
溯源树
样例
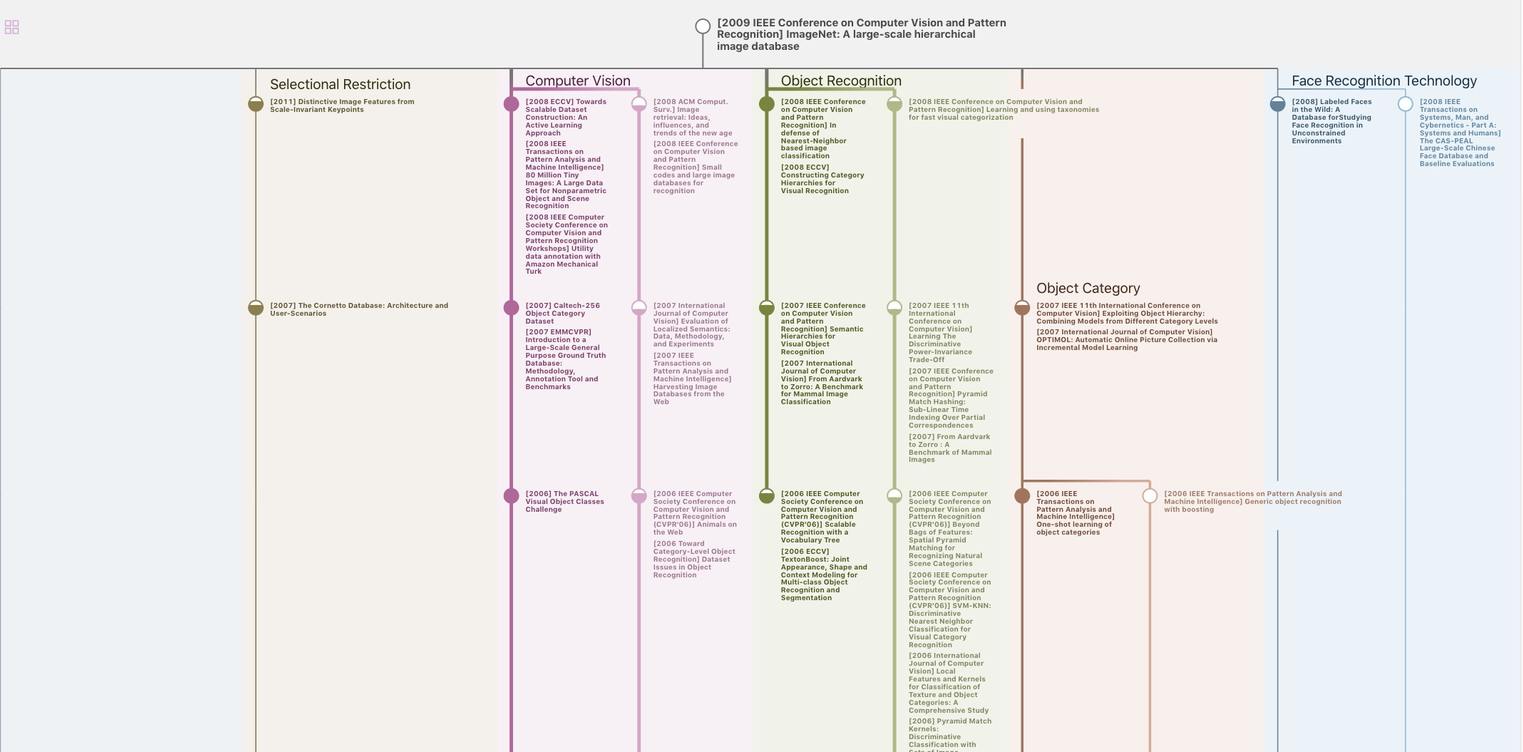
生成溯源树,研究论文发展脉络
Chat Paper
正在生成论文摘要