Learning To Run A Power Network Challenge For Training Topology Controllers
ELECTRIC POWER SYSTEMS RESEARCH(2020)
摘要
For power grid operations, a large body of research focuses on using generation redispatching, load shedding or demand side management flexibilities. However, a less costly and potentially more flexible option would be grid topology reconfiguration, as already partially exploited by Coreso (European RSC) and RTE (French TSO) operations. Beyond previous work on branch switching, bus reconfigurations are a broader class of actions and could provide some substantial benefits to route electricity and optimize the grid capacity to keep it within safety margins. Because of its non-linear and combinatorial nature, no existing optimal power flow solver can yet tackle this problem. We here propose a new framework to learn topology controllers through imitation and reinforcement learning. We present the design and the results of the first "Learning to Run a Power Network" challenge released with this framework. We finally develop a method providing performance upper-bounds (oracle), which highlights remaining unsolved challenges and suggests future directions of improvement.
更多查看译文
关键词
Artificial intelligence, Control, Power flow, Reinforcement learning, Competition
AI 理解论文
溯源树
样例
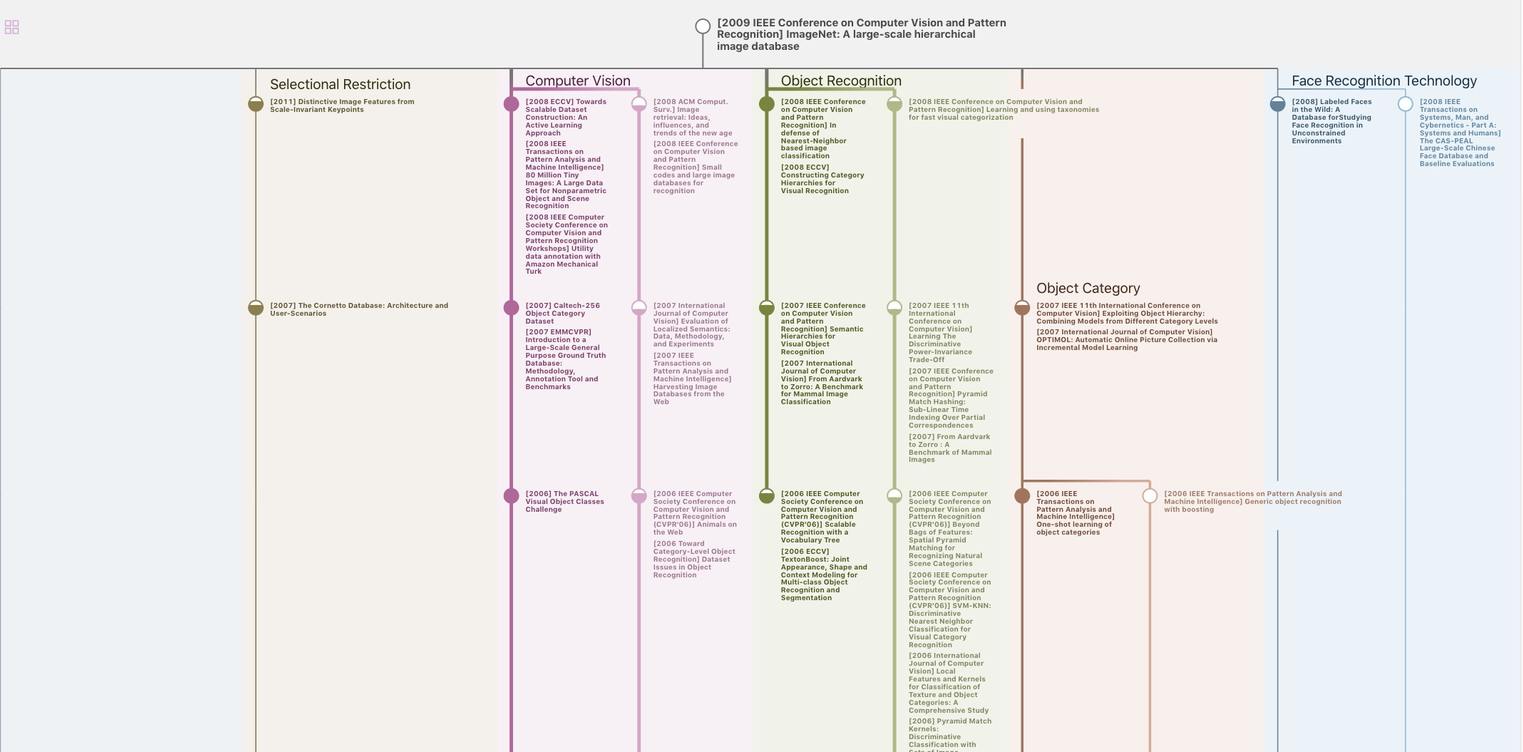
生成溯源树,研究论文发展脉络
Chat Paper
正在生成论文摘要