Learning Disentangled Representations via Mutual Information Estimation
European Conference on Computer Vision(2020)
摘要
In this paper, we investigate the problem of learning disentangled representations. Given a pair of images sharing some attributes, we aim to create a low-dimensional representation which is split into two parts: a shared representation that captures the common information between the images and an exclusive representation that contains the specific information of each image. To address this issue, we propose a model based on mutual information estimation without relying on image reconstruction or image generation. Mutual information maximization is performed to capture the attributes of data in the shared and exclusive representations while we minimize the mutual information between the shared and exclusive representation to enforce representation disentanglement. We show that these representations are useful to perform downstream tasks such as image classification and image retrieval based on the shared or exclusive component. Moreover, classification results show that our model outperforms the state-of-the-art models based on VAE/GAN approaches in representation disentanglement.
更多查看译文
关键词
Representation learning,Representation disentanglement,Mutual information maximization and minimization
AI 理解论文
溯源树
样例
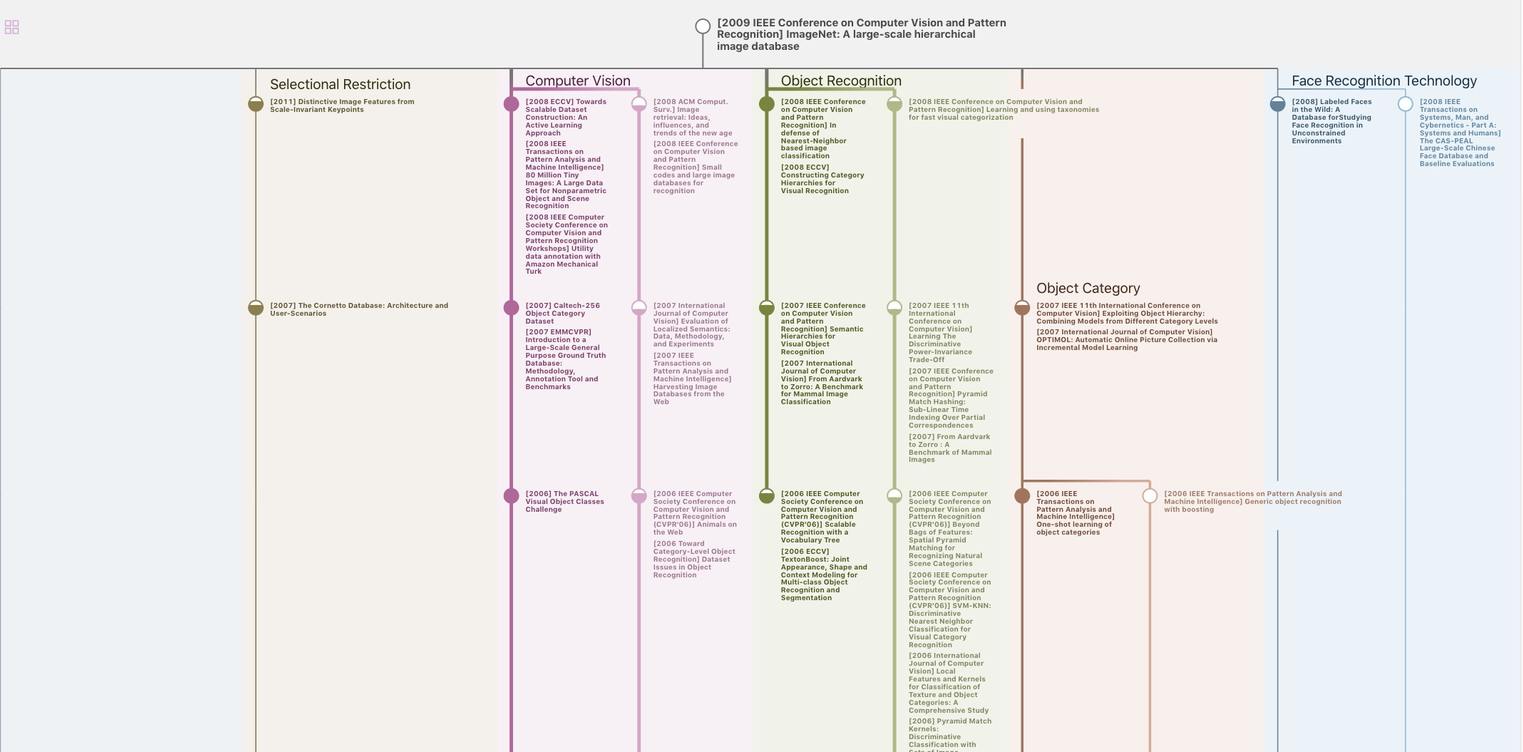
生成溯源树,研究论文发展脉络
Chat Paper
正在生成论文摘要