Driving Style Encoder: Situational Reward Adaptation for General-Purpose Planning in Automated Driving
ICRA(2020)
摘要
General-purpose planning algorithms for automated driving combine mission, behavior, and local motion planning. Such planning algorithms map features of the environment and driving kinematics into complex reward functions. To achieve this, planning experts often rely on linear reward functions. The specification and tuning of these reward functions is a tedious process and requires significant experience. Moreover, a manually designed linear reward function does not generalize across different driving situations. In this work, we propose a deep learning approach based on inverse reinforcement learning that generates situation-dependent reward functions. Our neural network provides a mapping between features and actions of sampled driving policies of a model-predictive control-based planner and predicts reward functions for upcoming planning cycles. In our evaluation, we compare the driving style of reward functions predicted by our deep network against clustered and linear reward functions. Our proposed deep learning approach outperforms clustered linear reward functions and is at par with linear reward functions with a-priori knowledge about the situation.
更多查看译文
关键词
situational reward adaptation,general-purpose planning algorithms,automated driving,planning algorithm,driving kinematics,linear reward function,driving situation,deep learning approach,situation-dependent reward functions,sampled driving policies,driving style,planning cycle
AI 理解论文
溯源树
样例
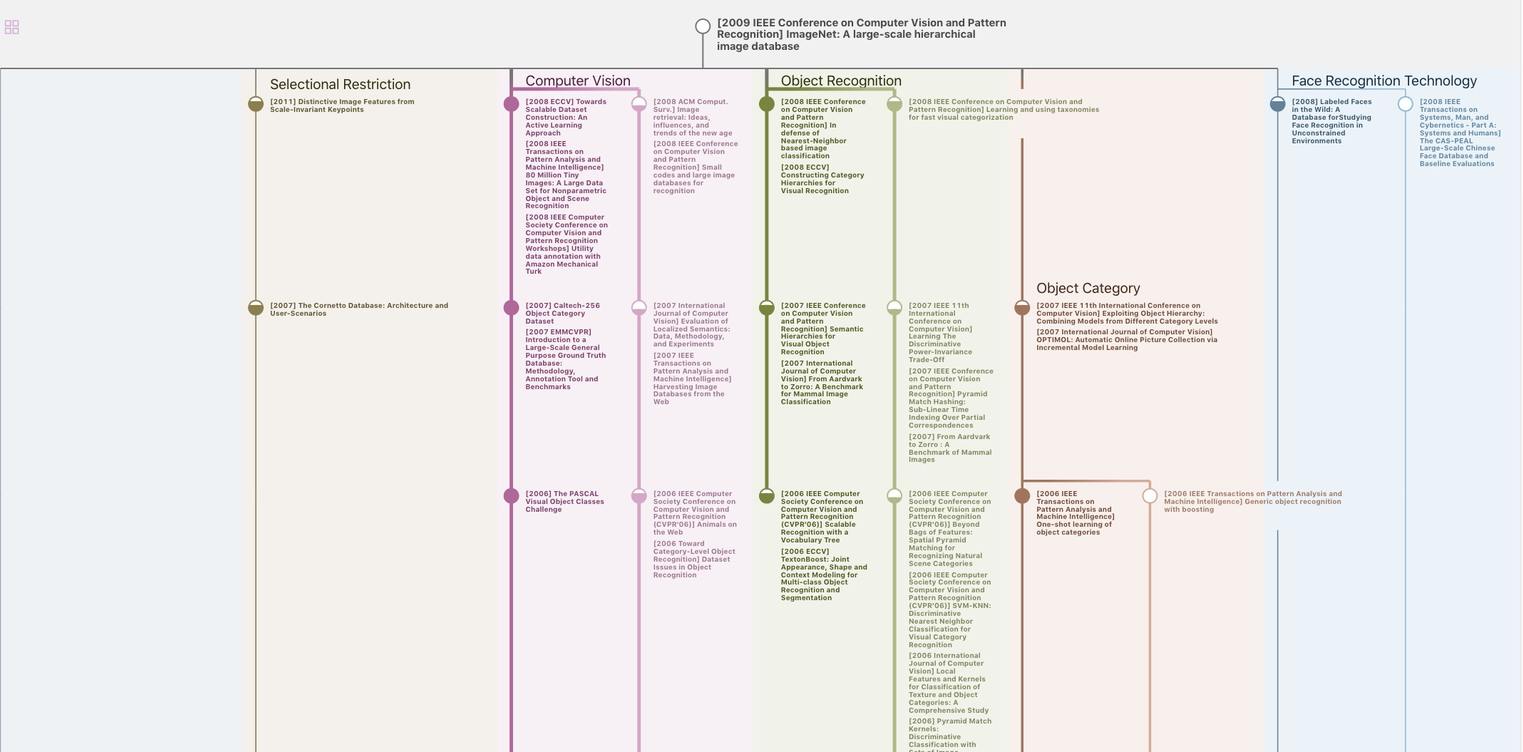
生成溯源树,研究论文发展脉络
Chat Paper
正在生成论文摘要