Differentially private synthetic mixed-type data generation for unsupervised learning
arxiv(2021)
摘要
We introduce the DP-auto-GAN framework for synthetic data generation, which combines the low dimensional representation of autoencoders with the flexibility of Generative Adversarial Networks (GANs). This framework can be used to take in raw sensitive data and privately train a model for generating synthetic data that will satisfy similar statistical properties as the original data. This learned model can generate an arbitrary amount of synthetic data, which can then be freely shared due to the post-processing guarantee of differential privacy. Our framework is applicable to unlabeled mixed-type data, that may include binary, categorical, and real-valued data. We implement this framework on both binary data (MIMIC-III) and mixed-type data (ADULT), and compare its performance with existing private algorithms on metrics in unsupervised settings. We also introduce a new quantitative metric able to detect diversity, or lack thereof, of synthetic data.
更多查看译文
关键词
Differential privacy,synthetic data generation,generative adversarial networks,mixed-type data
AI 理解论文
溯源树
样例
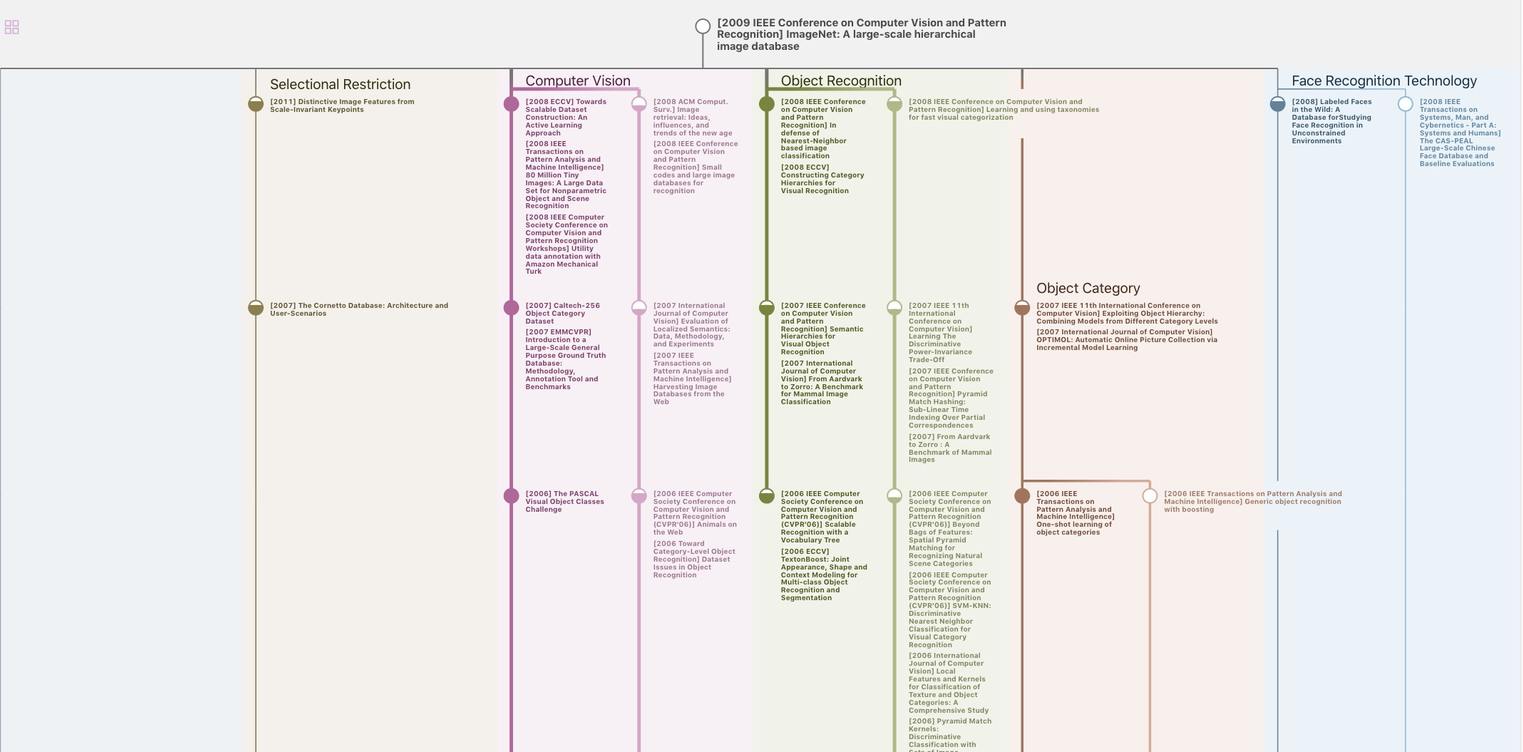
生成溯源树,研究论文发展脉络
Chat Paper
正在生成论文摘要