Affine invariant interacting Langevin dynamics for Bayesian inference
SIAM JOURNAL ON APPLIED DYNAMICAL SYSTEMS(2020)
摘要
We propose a computational method (with acronym ALDI) for sampling from a given target distribution based on first-order (overdamped) Langevin dynamics which satisfies the property of affine invariance. The central idea of ALDI is to run an ensemble of particles with their empirical covariance serving as a preconditioner for their underlying Langevin dynamics. ALDI does not require taking the inverse or square root of the empirical covariance matrix, which enables application to high-dimensional sampling problems. The theoretical properties of ALDI are studied in terms of nondegeneracy and ergodicity. Furthermore, we study its connections to diffusion on Riemannian manifolds and Wasserstein gradient flows. Bayesian inference serves as a main application area for ALDI. In case of a forward problem with additive Gaussian measurement errors, ALDI allows for a gradient-free approximation in the spirit of the ensemble Kalman filter. A computational comparison between gradient-free and gradient-based ALDI is provided for a PDE constrained Bayesian inverse problem.
更多查看译文
关键词
Langevin dynamics,interacting particle systems,Bayesian inference,gradient flow,multiplicative noise,affine invariance,gradient-free
AI 理解论文
溯源树
样例
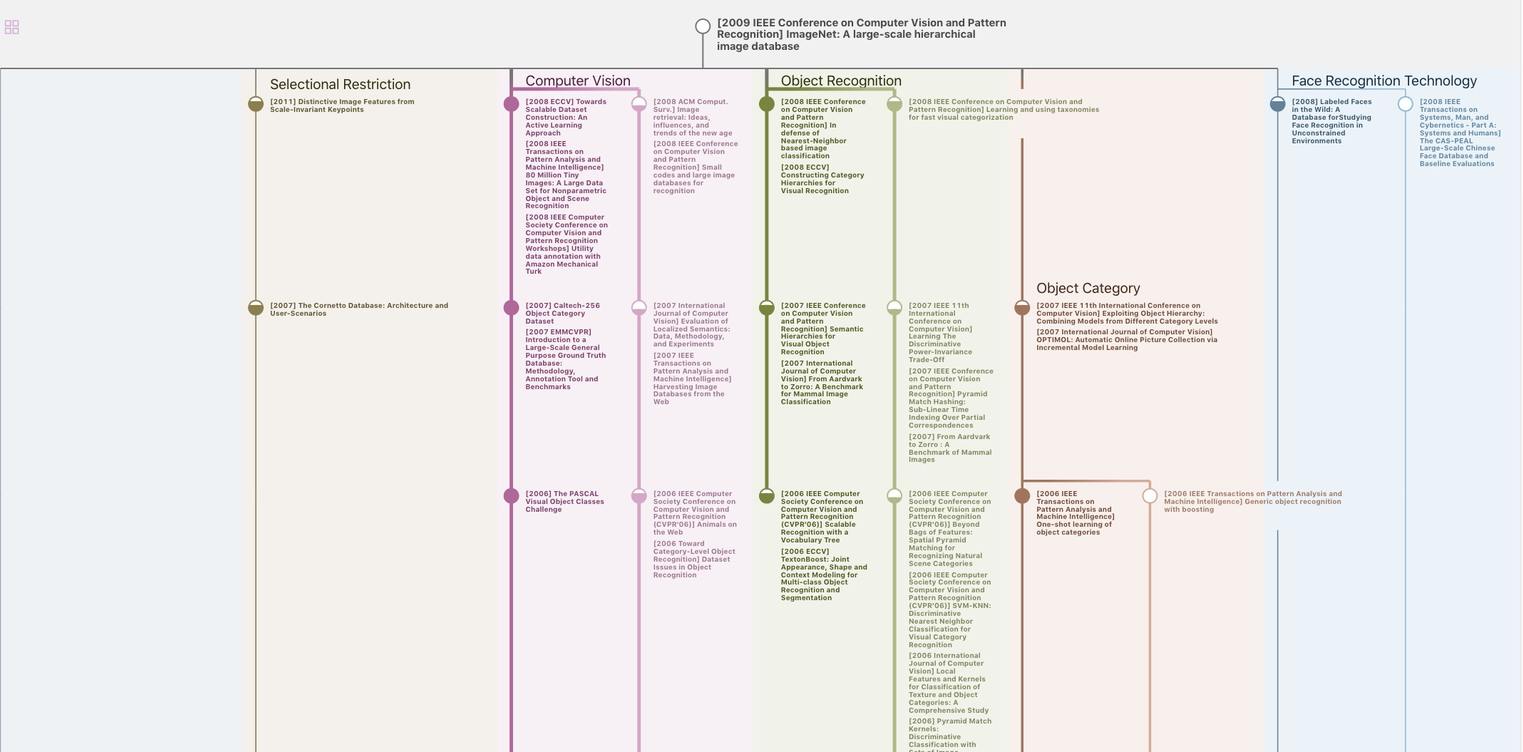
生成溯源树,研究论文发展脉络
Chat Paper
正在生成论文摘要