A Parallelized, Momentum-Incorporated Stochastic Gradient Descent Scheme For Latent Factor Analysis On High-Dimensional And Sparse Matrices From Recommender Systems
2019 IEEE INTERNATIONAL CONFERENCE ON SYSTEMS, MAN AND CYBERNETICS (SMC)(2019)
摘要
High-dimensional and sparse (HiDS) matrices are ommonly encountered in many big-data-related industrial applications like recommender systems. Latent factor (LF) analysis via stochastic gradient descent (SGD) is greatly efficient in discovering latent patterns from them. However, as a sequential algorithm, SGD suffers considerable time cost and low scalability when handling large-scale problems. To address these issues, this study proposes parallelized, momentum-incorporated stochastic gradient descent (PMSGD) scheme, which incorporates momentum effects into an SGD scheme as well as implementing its parallelization via careful data splitting. Based on a PMSGD method, we achieve a PMSGD-based LF (PLF) model to execute fast LF analysis on HiDS matrices from a recommender system. Experimental results on two HiDS matrices arising from industrial applications indicate that owing to the careful design of PMSGD, a PLF model outperforms state-of-the-art parallel LF models significantly in terms of computational efficiency.
更多查看译文
关键词
Big Data, Recommender System, Latent Factor Analysis, Stochastic Gradient Descent, Parallelization, Momentum Method
AI 理解论文
溯源树
样例
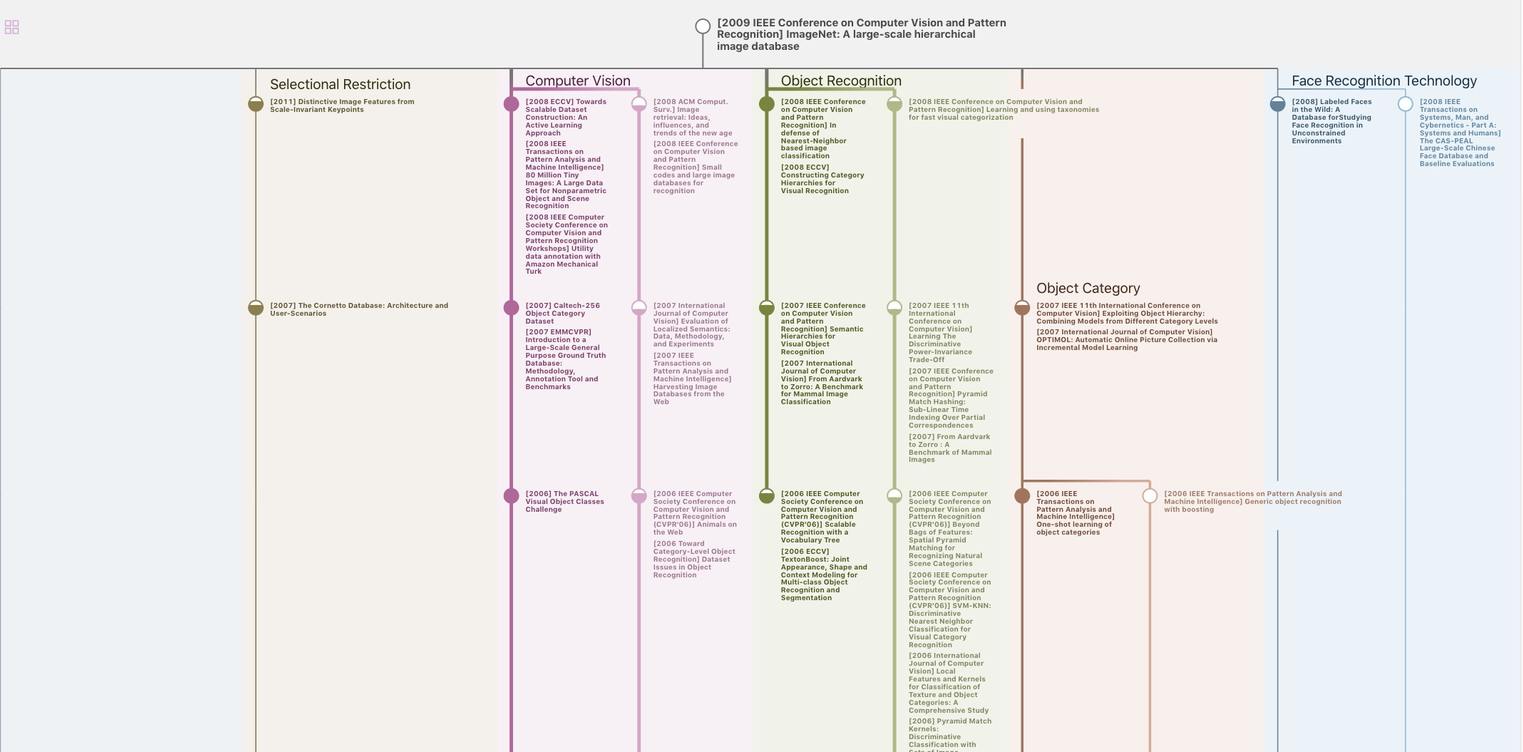
生成溯源树,研究论文发展脉络
Chat Paper
正在生成论文摘要