What Makes You Look Like You: Learning an Inherent Feature Representation for Person Re-Identification
2019 16th IEEE International Conference on Advanced Video and Signal Based Surveillance (AVSS)(2019)
摘要
In this work, we address person re-identification (ReID) by learning an inherent feature representation (inherent code) that is unique to each individual. This task is difficult because the appearance of a person may vary dramatically due to diverse factors, such as illuminations, viewpoints, and human pose changes. To tackle this issue, we propose new learning objectives to learn the inherent code for each person based on deep learning. Specifically, the proposed deep-net model is trained by jointly optimizing the multiple objectives that pulls the instances of the same person closer while pushing the instances belonging to different persons far from each other. Owing to such complementary designs, the deep-net model yields a robust code for each individual and hence better solve person ReID. Promising experimental results demonstrate the robustness and effectiveness of our proposed method.
更多查看译文
关键词
inherent feature representation,inherent code,deep learning,person ReID,deep-net model
AI 理解论文
溯源树
样例
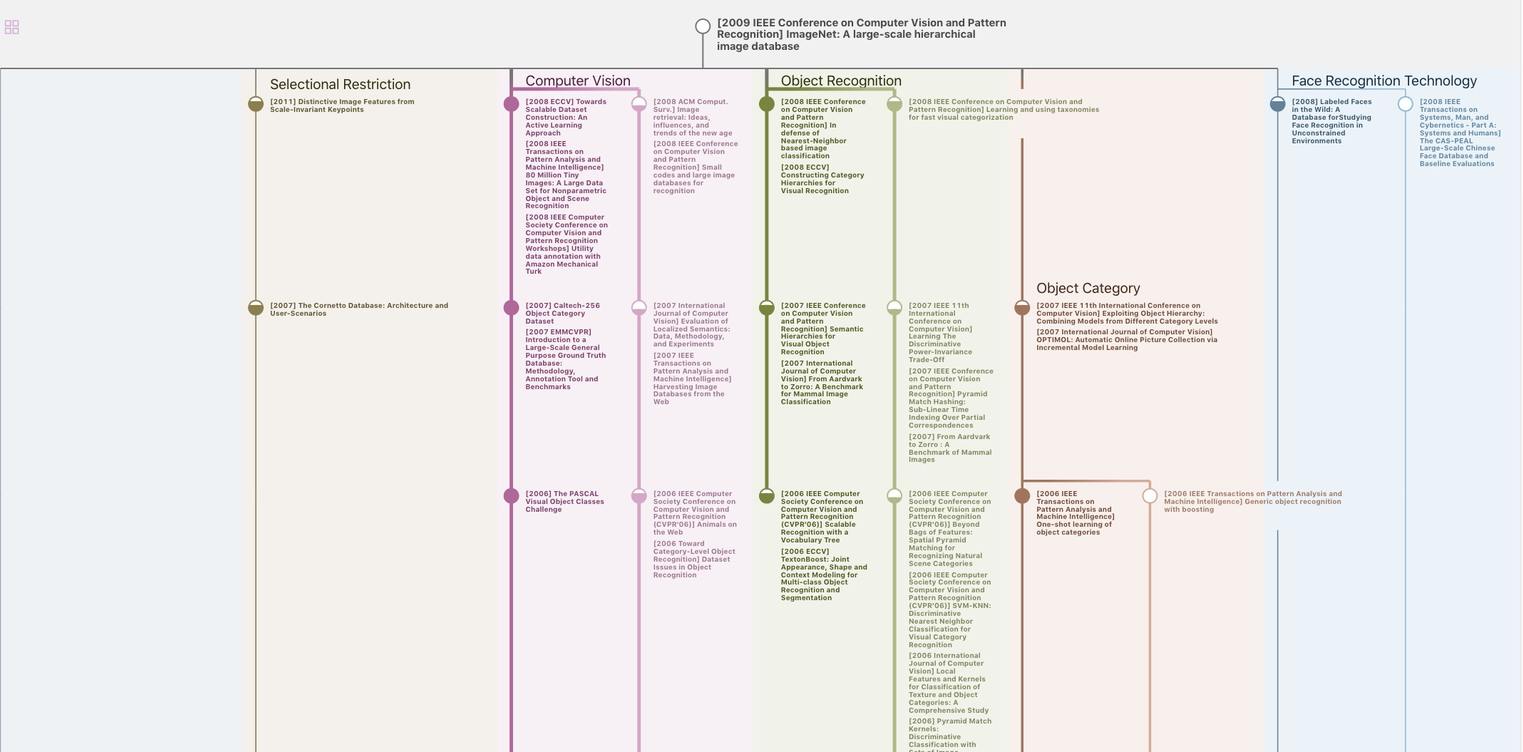
生成溯源树,研究论文发展脉络
Chat Paper
正在生成论文摘要