Dqa: Scalable, Automated And Interactive Data Quality Advisor
2019 IEEE INTERNATIONAL CONFERENCE ON BIG DATA (BIG DATA)(2019)
摘要
Fueled with growth in the fields of Internet of Things (IoT) and Big Data, data has become one of the most valuable assets in today's world. While we are leveraging this data for analyzing complex systems using machine learning and deep learning, a considerable amount of time and effort is spent on addressing data quality issues. if undetected, data quality issues can cause large deviations in the analysis, misleading data scientists. To ease the effort of identifying and addressing data quality challenges, we introduce DQA, a scalable, automated and interactive data quality advisor. In this paper, we describe the DQA framework, provide detailed description of its components and the benefits of integrating it in a data science process. We propose a programmatic approach for implementing the data quality framework which automatically generates dynamic executable graphs for performing data validations fine-tuned for a given dataset. We discuss the use of DQA to build a library of validation checks common to many applications. We provide insight into how DQA addresses many persistence and usability issues which currently make data cleaning a laborious task for data scientists. Finally, we provide a case study of how DQA is implemented in a real-world system and describe the benefits realized.
更多查看译文
关键词
Data quality, machine learning, data cleaning, scalability, automation, data science
AI 理解论文
溯源树
样例
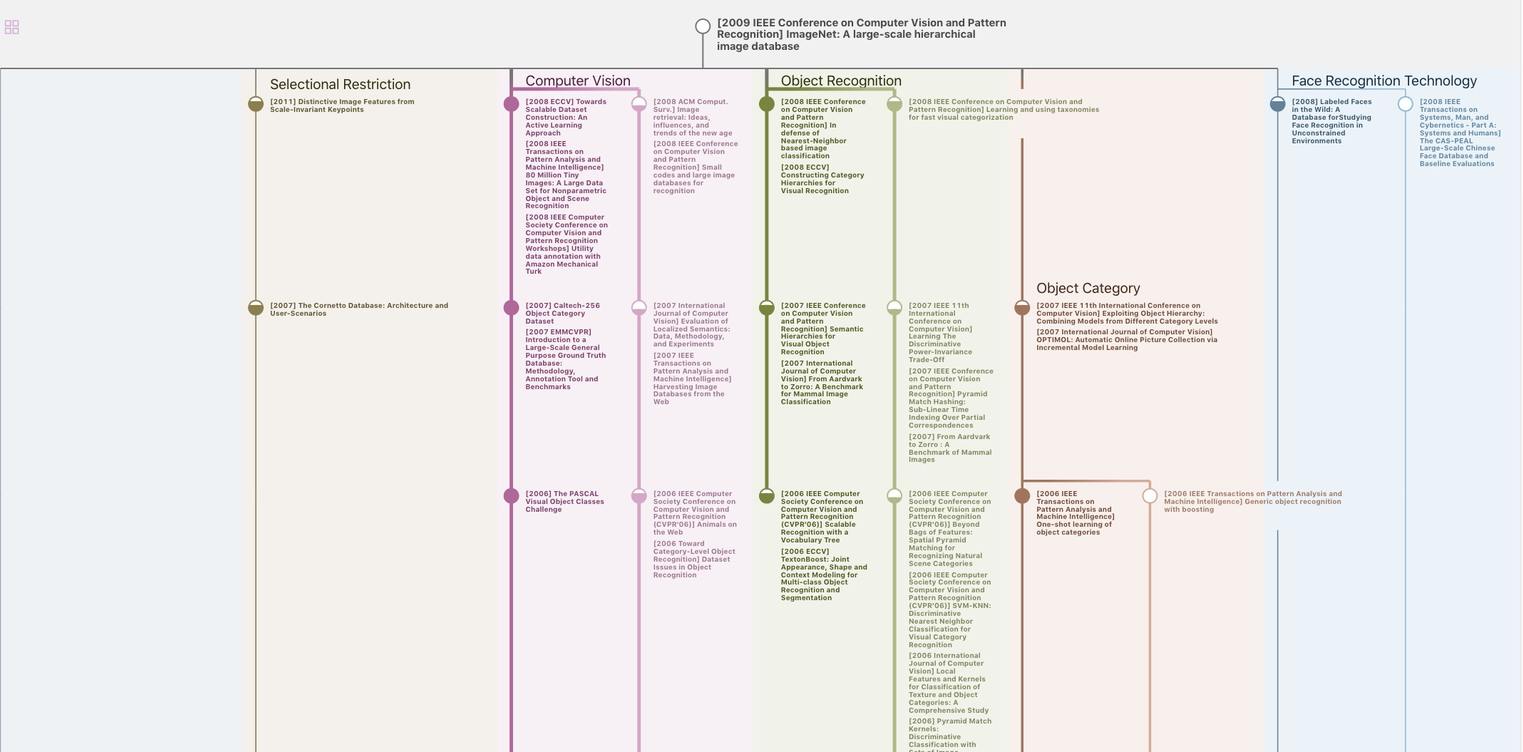
生成溯源树,研究论文发展脉络
Chat Paper
正在生成论文摘要