Detecting Pedestrian Crossing Events In Large Video Data From Traffic Monitoring Cameras
2019 IEEE INTERNATIONAL CONFERENCE ON BIG DATA (BIG DATA)(2019)
摘要
Pedestrian safety on the road is a priority for transportation system managers and operators. While there are a number of treatments and technologies to effectively improve pedestrian safety, identifying the location where these are most needed remains a challenge. Mid-block locations, where safety countermeasures are often needed the most, are typically harder to monitor. Current practice often requires manual observation of candidate locations for limited time periods, leading to an identification process that is often time consuming, lags behind traffic pattern changes over time, and lacks scalability. As a result, target locations are often selected reactively, after serious traffic incidents reveal an underlying safety issue. We propose an approach to use data collected by existing traffic monitoring cameras to automatically identify pedestrian activities on the road. We propose an algorithm to detect pedestrian crossing events based on the detection of individuals on individual video frames using a deep neural network model. Resulting pedestrian locations and movement trajectories can be visualized on a background image, which is automatically extracted at the analyzed location from the video. We demonstrate and evaluate our approach with a real-world use case. The case study considered in this work uses cameras owned by the City of Austin, Texas to study pedestrian road use before and after the deployment of a pedestrian-hybrid beacon. We explore qualitative and quantitative metrics to describe pedestrian activity and corresponding changes, which may be used to prioritize the deployment of pedestrian safety solutions, or evaluate their performance. We compared the number of crossing events detected per hour with manually reviewed results from a selected day. The result shows 67 percent overall accuracy, although we observe significant variability across times-of-day. Despite observed limitations, our work illustrates how the value of existing traffic camera networks can be augmented beyond everyday traffic monitoring, and used to collect valuable information on road usage by pedestrians.
更多查看译文
关键词
big data, traffic camera video analysis, pedestrian safety, deep learning
AI 理解论文
溯源树
样例
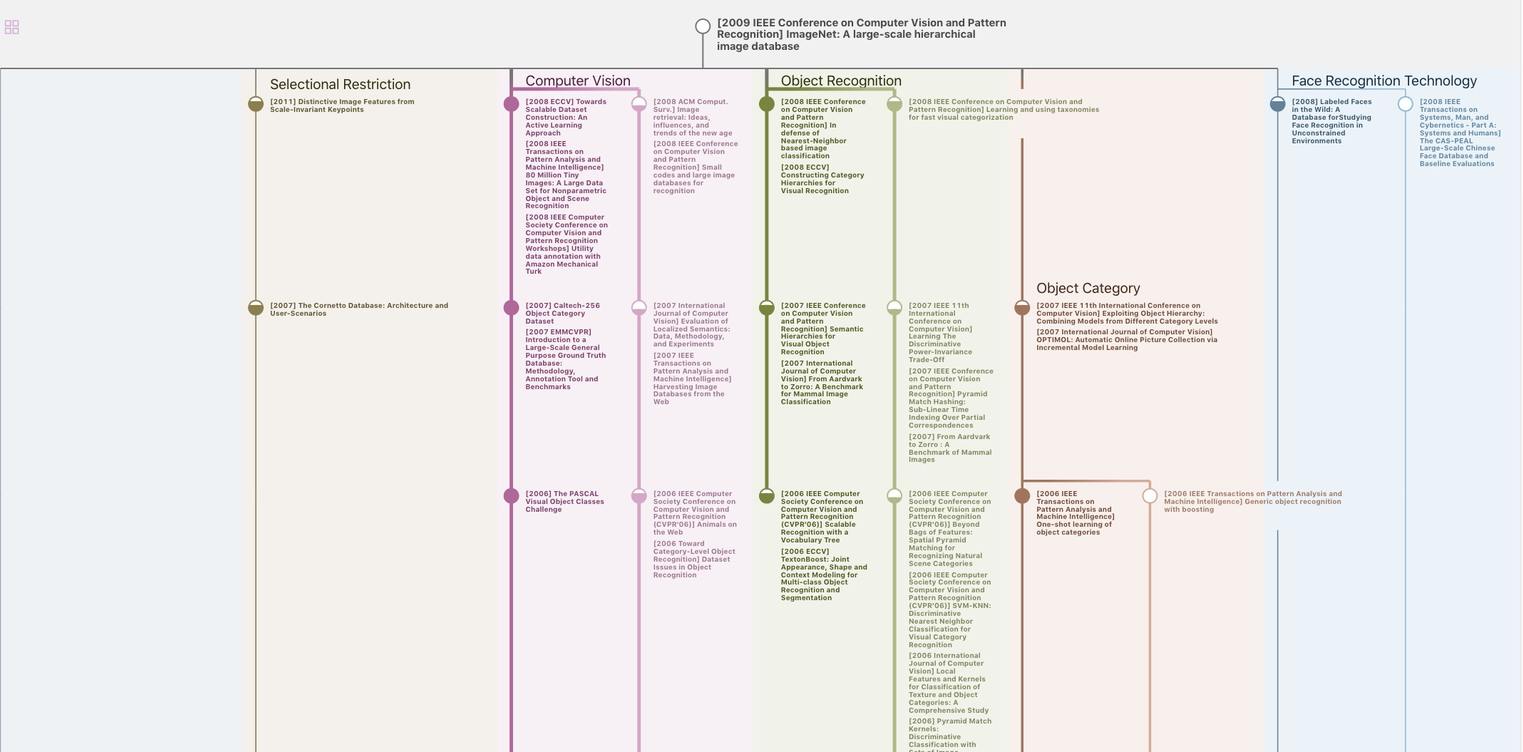
生成溯源树,研究论文发展脉络
Chat Paper
正在生成论文摘要