An Approach for Scale Suspicious Network Events Detection
2019 IEEE INTERNATIONAL CONFERENCE ON BIG DATA (BIG DATA)(2019)
摘要
Detecting the real suspicious events from a large number of low-quality alerts is a severe challenge to the security operations center teams. In this paper, we present an approach to this problem by following the sequence of machine learning steps. The highlight of our approach is the method to generate two simple but effective categories of features based on group and aggregation operations, which can scale with a large number of alerts using MapReduce framework. The two generated types of features are local features and global features. The local features cover the alert aggregation information of the same group of events, while the global features cover the network aggregation information of different groups of events. Moreover, we also introduce the model stacking mechanism to enhance the robustness of the model. The proposed approach achieves AUC scores of 0.9512 on the validating dataset and 0.9303 on the test set, which is the 2
nd
highest final score in the competition.
更多查看译文
关键词
alert correlation, network security, stacking model, map reduce, big data
AI 理解论文
溯源树
样例
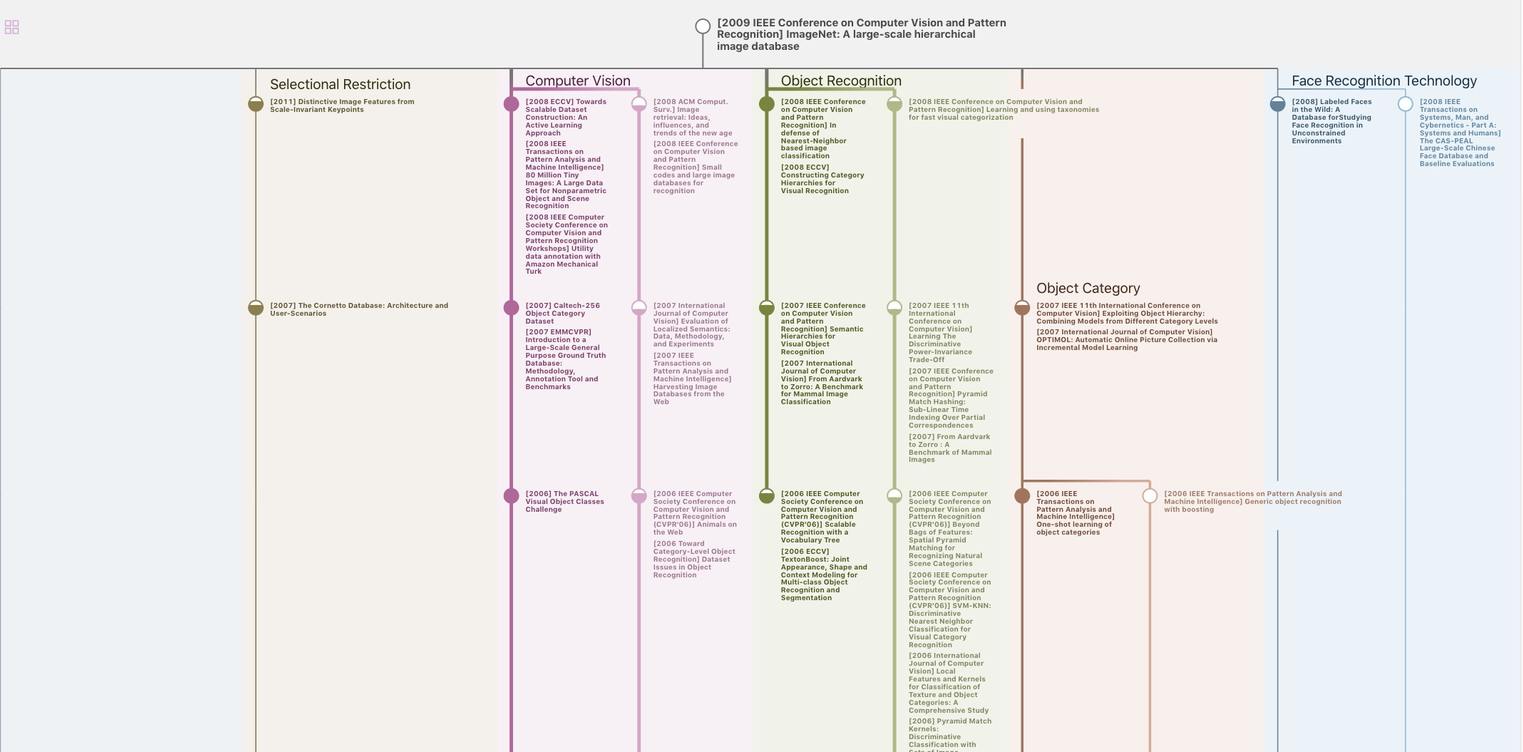
生成溯源树,研究论文发展脉络
Chat Paper
正在生成论文摘要