Incentive Design for Crowdsourced Development of Selective AI for Human and Machine Data Processing: A Case Study
2019 IEEE INTERNATIONAL CONFERENCE ON BIG DATA (BIG DATA)(2019)
摘要
The most typical approach today to data processing which does not have proven algorithms is to first request humans to provide labels to a small set of data and then develop artificial intelligences (AIs) with the data to perform all the remaining tasks. This development is sometimes crowdsourced through platforms such as Kaggle. The approach, however, is not always effective; if the AI does not meet the quality requirement, we may have to give up the development and all the data items have to be done manually. In order to avoid this all-or-nothing situation, “selective” AI programs that perform tasks which they are confident to do will be effective. This study addresses the problem of designing an incentive structure for crowdsourcing the development of such selective AI programs. This paper shows the results of our real-world experiment with a stair-step incentive structure and the behavior of a worker who developed the AI agent under the incentive. This paper also discusses the limitations of the proposed incentive design.
更多查看译文
关键词
crowdsourcing, software development, real-world experiments
AI 理解论文
溯源树
样例
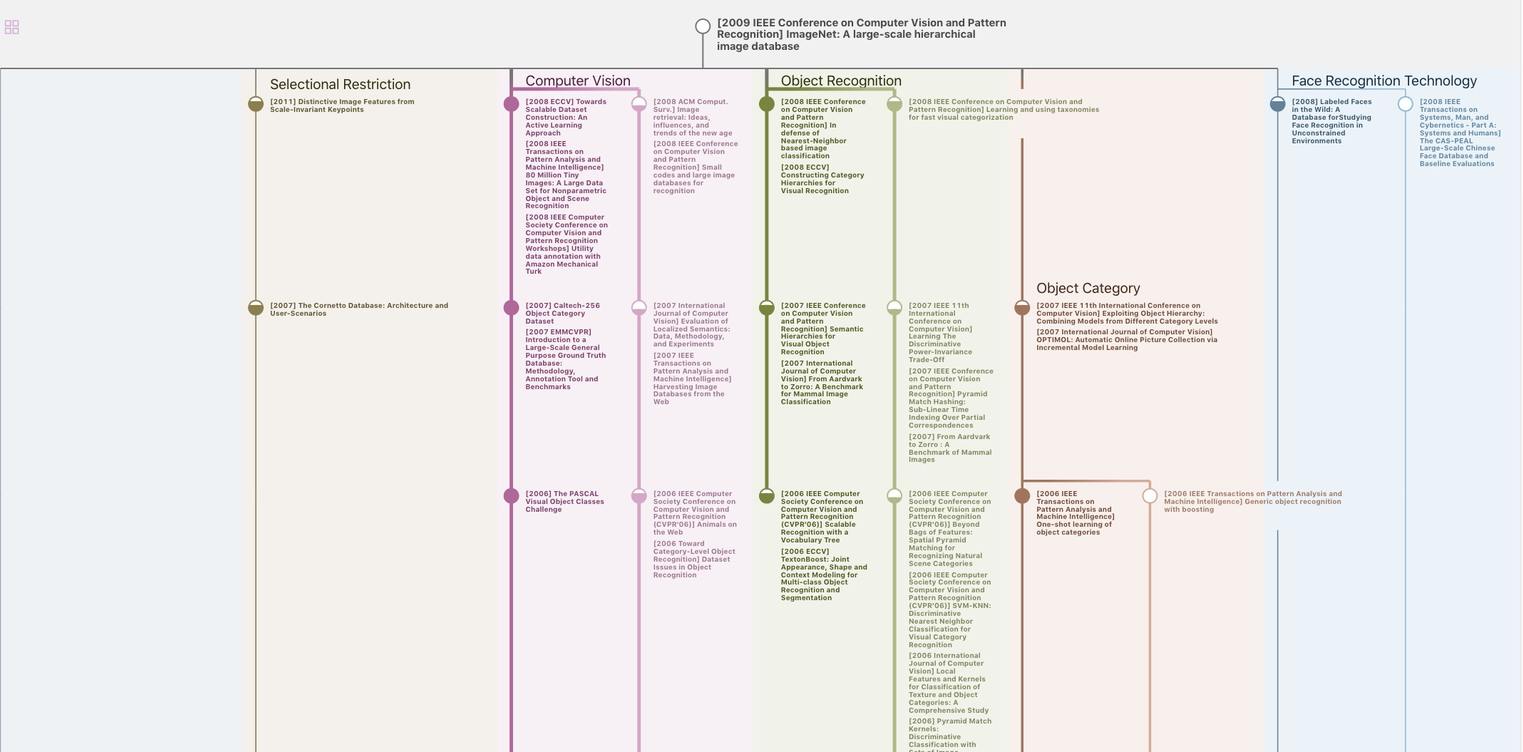
生成溯源树,研究论文发展脉络
Chat Paper
正在生成论文摘要