Examination Of Double Arbiter Pufs On Security Against Machine Learning Attacks
2019 IEEE INTERNATIONAL CONFERENCE ON BIG DATA (BIG DATA)(2019)
摘要
Security is important for the functioning of Internet-of-Things (loTs). Many loT devices are closely physically approachable by the crowd, making cryptographic key-based security protocols vulnerable to side-channel attacks. Physical Unclonable Functions (PUF) are emerging as a promising keyless solution by utilizing inherent variations of integrated circuits (ICs) to produce different responses from different devices. While physically unclonable, some PUFs were reported to be mathematically clonable by machine learning (ML) methods. XOR PUFs are a group of PUFs mathematically clonable when a large number of challenge-response pairs (CRPs) are available to attackers. Double Arbiter PUFs (DAPUFs) were developed for increased security against machine learning attacks over XOR PUFs, and studies showed that DAPUFs are highly secure against attacks using Support Vector Machine (SVM). In this paper, we investigate how secure DAPUFs arc against neural network-based attack methods and compare the DAPUFs' performance with that of XOR PUFs. The results confirm with existing studies on DAPUFs' higher security when compared with XOR PUFs, but also discovered that DAPUFs are not secure against neural network-based attacks if attackers can obtain a large number of CRPs, revealing a security vulnerability of those DAPUFs we examined in this study.
更多查看译文
关键词
Physical Unclonable Functions, Double Arbiter PUF, Machine Learning, Internet Of Things
AI 理解论文
溯源树
样例
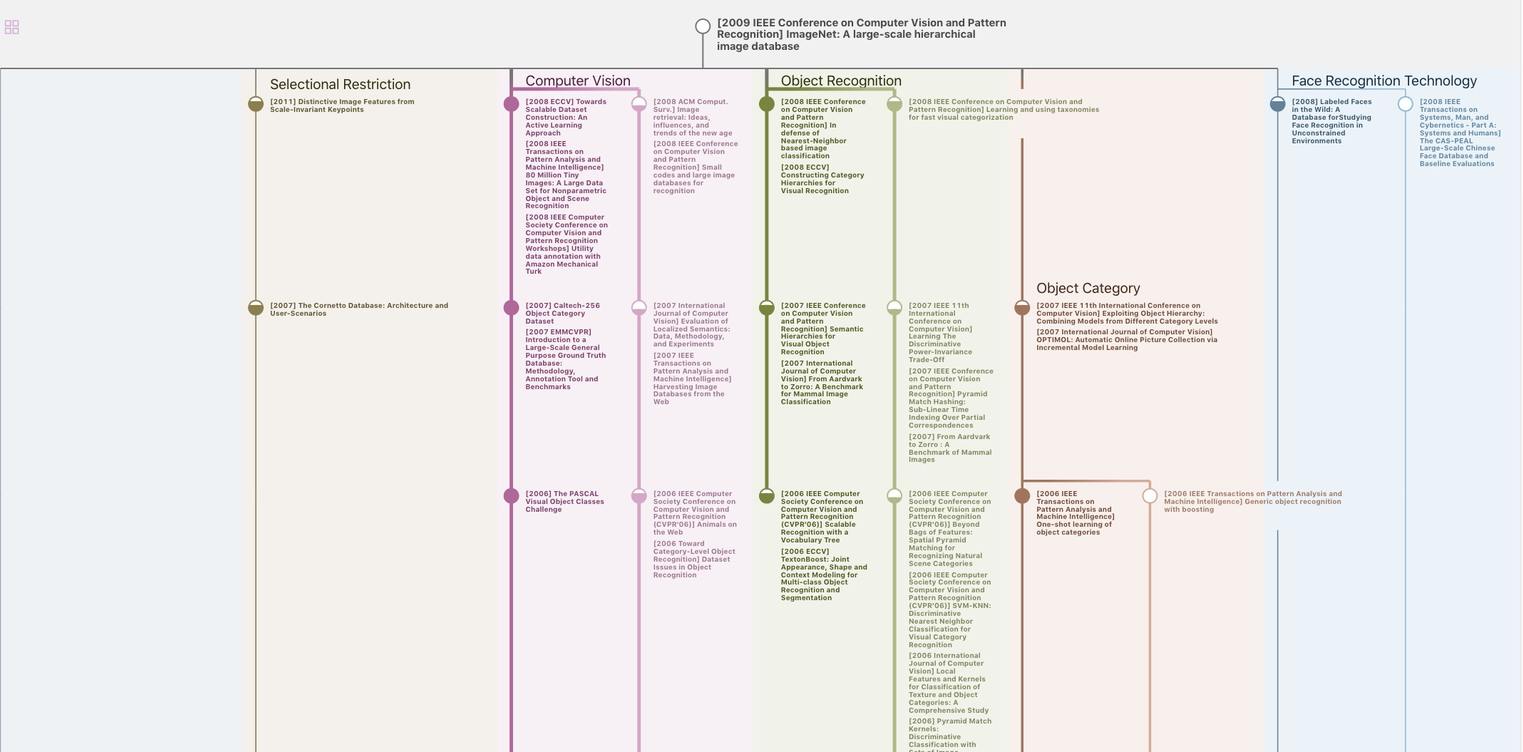
生成溯源树,研究论文发展脉络
Chat Paper
正在生成论文摘要