Fast Computation Of Persistent Homology With Data Reduction And Data Partitioning
2019 IEEE INTERNATIONAL CONFERENCE ON BIG DATA (BIG DATA)(2019)
摘要
Persistent hornology is a method of data analysis that is based in the mathematical field of topology. Unfortunately. the run-time and memory complexities associated with computing persistent homology inhibit general use for the analysis of big data. For example, the best tools currently available to compute persistent homology can process only a few thousand data points in R-3. Several studies have proposed using sampling or data reduction methods to attack this limit. While these approaches enable the computation of persistent homology on much larger data sets, the methods are approximate. Furthermore, while they largely preserve the results of large topological features, they generally miss reporting information about the small topological features that are present in the data set. While this abstraction is useful in many cases, there are data analysis needs where the smaller features are also significant (e.g., brain artery analysis). This paper explores a combination of data reduction and data partitioning to compute persistent homology on big data that enables the identification of both large and small topological features from the input data set. To reduce the approximation errors that typically accompany data reduction for persistent homology, the described method also includes a mechanism of "upscaling" the data circumscribing the large topological features that are computed from the sampled data. The designed experimental method provides significant results for improving the scale at which persistent homology can be performed.
更多查看译文
关键词
topological data analysis, persistent homology, data reduction, data partitioning, data mining, unsupervised learning
AI 理解论文
溯源树
样例
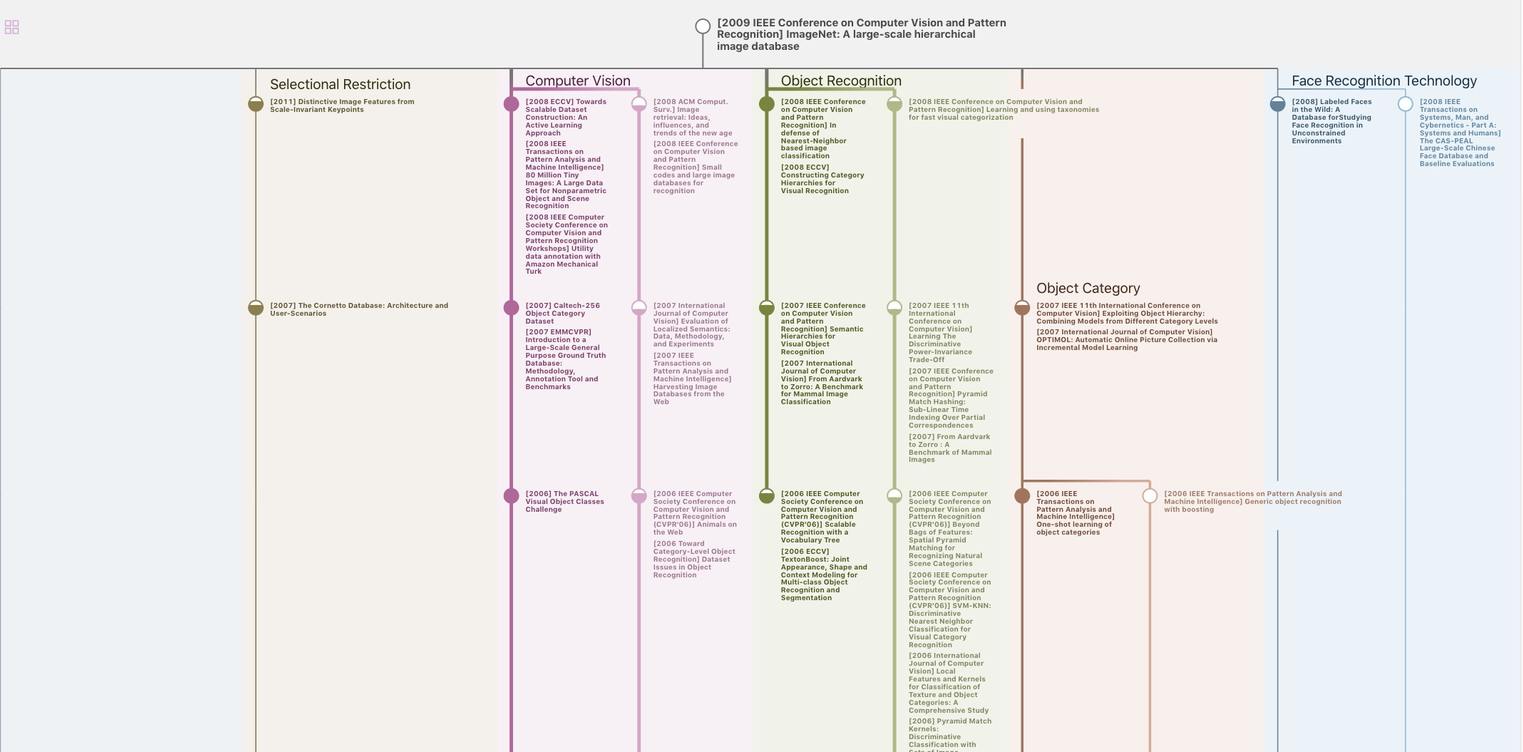
生成溯源树,研究论文发展脉络
Chat Paper
正在生成论文摘要