Nearest Neighbor Subsequence Search In Time Series Data
2019 IEEE INTERNATIONAL CONFERENCE ON BIG DATA (BIG DATA)(2019)
摘要
Continuous growth in sensor data and other temporal sequence data necessitates efficient retrieval and similarity search support On these big time series dalasels. However, finding exact similarity results, especially at the granularity of subsequences, is known to be prohibitively costly for large data sets. In this paper, we thus propose an efficient framework for solving this exact subsequence similarity match problem, called TINN (Time series Nearest Neighbor search). Exploiting the range interval diversity properties of time series datasets, TINN captures similarity at two levels of abstraction, namely, relationships among subsequences within each long time series and relationships across distinct time series in the data set. These relationships are compactly organized in an augmented relationship graph model, with the former relationships encoded in similarity vectors at TINN nodes and the later captured by augmented edge types in the TINN Graph. Query processing strategy deploy novel pruning techniques on the TINN Graph, including node skipping, vertical and horizontal pruning, to significantly reduce the number of time series as well as subsequences to be explored. Comprehensive experiments on synthetic and real world lime series data demonstrate that our T INN model consistently outperforms state-of-the-art approaches while still guaranteeing to retrieve exact matches.
更多查看译文
关键词
Time Series Data, Subsequence Mining, Nearest Neighbor Search
AI 理解论文
溯源树
样例
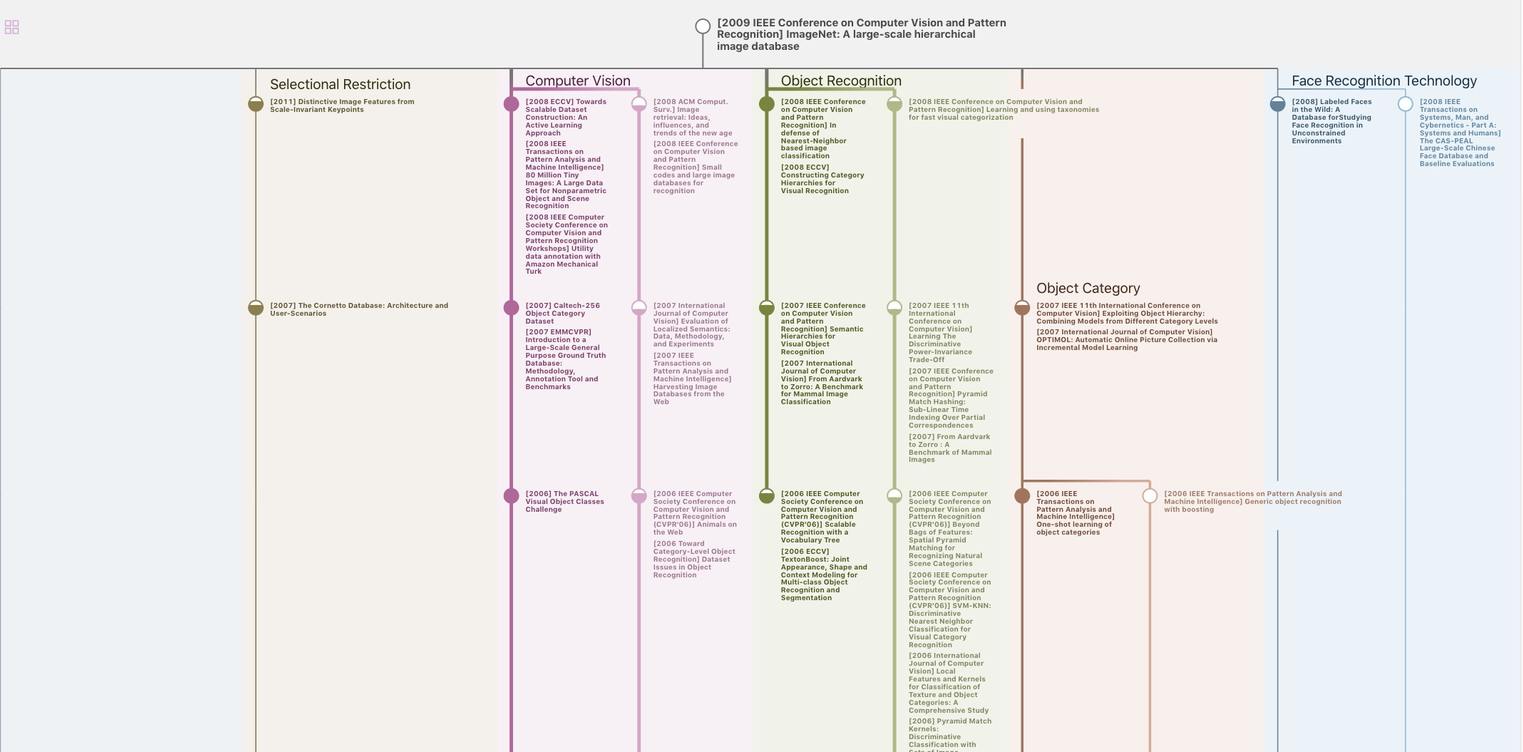
生成溯源树,研究论文发展脉络
Chat Paper
正在生成论文摘要