RecCite: A Hybrid Approach to Recommend Potential Papers
2019 IEEE INTERNATIONAL CONFERENCE ON BIG DATA (BIG DATA)(2019)
摘要
A systematic review of literature is a crucial aspect in pursuing any research problem. With the galloping growth in the number of researchers and scientific publications available online in digital libraries, there is information overload which makes the search of relevant papers cumbersome. An efficient academic paper recommendation process could be a promising maneuver for this purpose. The state-of-the-art methods in this domain primarily employ link-based approaches on citation network and do not consider the semantics (context) of papers being recommended, although the later one largely accounts for the relevance of recommendations. Also achieving online response time (scalability) is a perennial desire for any recommendation system. In this context we propose a hybrid approach “RecCite” that blends the popularity of papers with semantic similarity to acquire relevance. Further, the approach follows a top-down methodology for filtering papers from a more generic and larger network to a community pertaining to a SIG (Special Interest Group) that inherently addresses the scalability aspect. The proposed approach has been systematically evaluated with state-of-the-art baseline method using the publicly available Arnet-Miner data set. Satisfyingly, “RecCite” outperforms the baseline method with up to 37.66%, 20.14%, and 97.24% improvement in precision, recall, and response time, respectively.
更多查看译文
关键词
Citation Analysis, Recommender Systems, Popularity Network, Content Similarity, Community Analysis
AI 理解论文
溯源树
样例
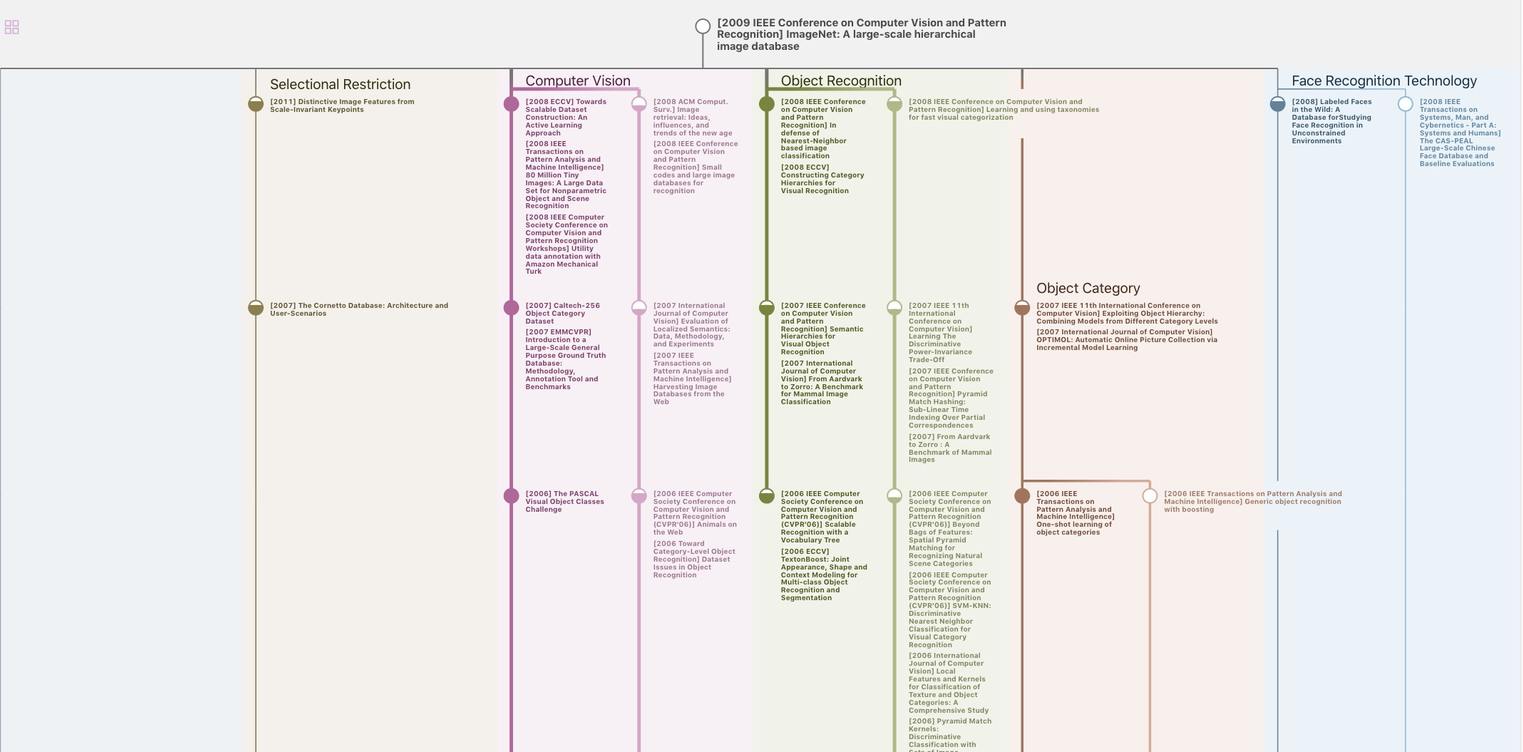
生成溯源树,研究论文发展脉络
Chat Paper
正在生成论文摘要