Bayesian Non-Linear Support Vector Machine For High-Dimensional Data With Incorporation Of Graph Information On Features
2019 IEEE INTERNATIONAL CONFERENCE ON BIG DATA (BIG DATA)(2019)
摘要
Support vector machine (SVM) is a popular classification method for analysis of high dimensional data such as genomics data. Recently a number of linear SVM methods have been developed to achieve feature selection through either frequentist regularization or Bayesian shrinkage, but the linear assumption may not be plausible for many real applications. In addition, recent work has demonstrated that incorporating known biological knowledge, such as those from functional genomics, into the statistical analysis of genomic data offers great promise of improved predictive accuracy and feature selection. Such biological knowledge can often be represented by graphs. In this article, we propose a novel knowledge-guided non-linear Bayesian SVM approach for analysis of high-dimensional data. Our model uses graph information that represents the relationship among the features to guide feature selection. To achieve knowledge-guided feature selection, we assign an Ising prior to the indicators representing inclusion/exclusion of the features in the model. An efficient MCMC algorithm is developed for posterior inference. The performance of our method is evaluated and compared with several penalized linear SVM and the standard kernel SVM method in terms of prediction and feature selection in extensive simulation studies. Also, analyses of genomic data from a cancer study show that our method yields a more accurate prediction model for patient survival and reveals biologically more meaningful results than the existing methods.
更多查看译文
关键词
Bayesian support vector machine, Gaussian process, Knowledge-guided, Pathway information, Ising prior
AI 理解论文
溯源树
样例
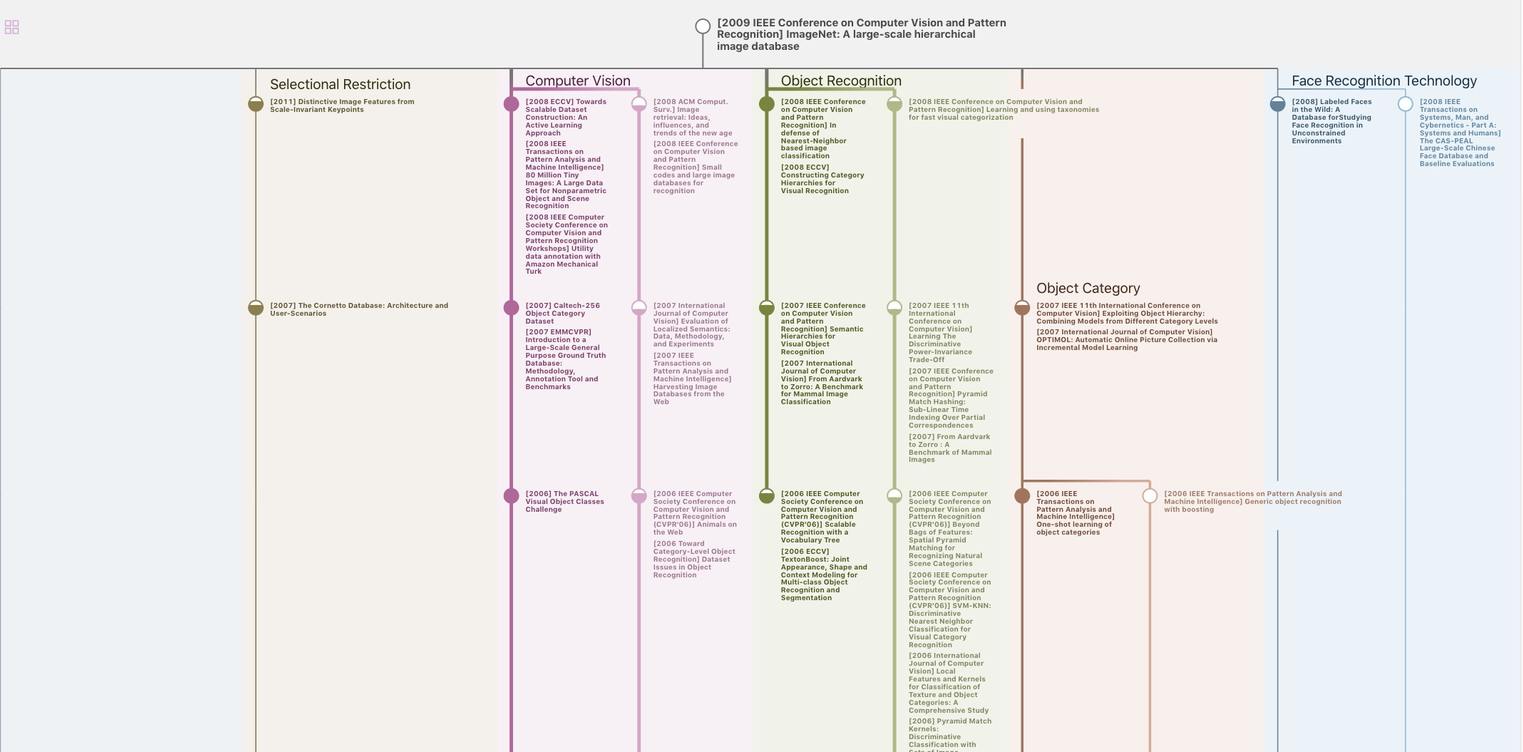
生成溯源树,研究论文发展脉络
Chat Paper
正在生成论文摘要