Meta-Path Reduction With Transition Probability Preserving In Heterogeneous Information Network
2019 IEEE INTERNATIONAL CONFERENCE ON BIG DATA (BIG DATA)(2019)
摘要
Heterogeneous Information Network (HIN) has attracted much attention due to its wide applicability in a variety of data mining tasks. A potentially large number of meta-paths can be extracted from the heterogeneous networks, providing abundant semantic knowledge. However, too many meta-paths may be redundant. Reduction on the number of meta-paths can enhance the effectiveness since some redundant meta-paths provide interferential linkage to the task. Moreover, the reduced meta-paths can reflect the characteristic of the heterogeneous network. In this paper, unlike previous supervised model, we propose a novel algorithm, SPMR (Semantic Preserving Metapath Reduction), to reduce a set of pre-defined meta-paths in an unsupervised setting. The proposed method is able to evaluate a set of meta-paths to maximally preserve the semantics of original meta-paths after reduction. Experimental results show that SPMR can select a succinct subset of meta-paths which can achieve comparable or even better performance with fewer meta-paths.
更多查看译文
关键词
heterogeneous information network, meta-path, reduction, semantic preserving, unsupervised learning
AI 理解论文
溯源树
样例
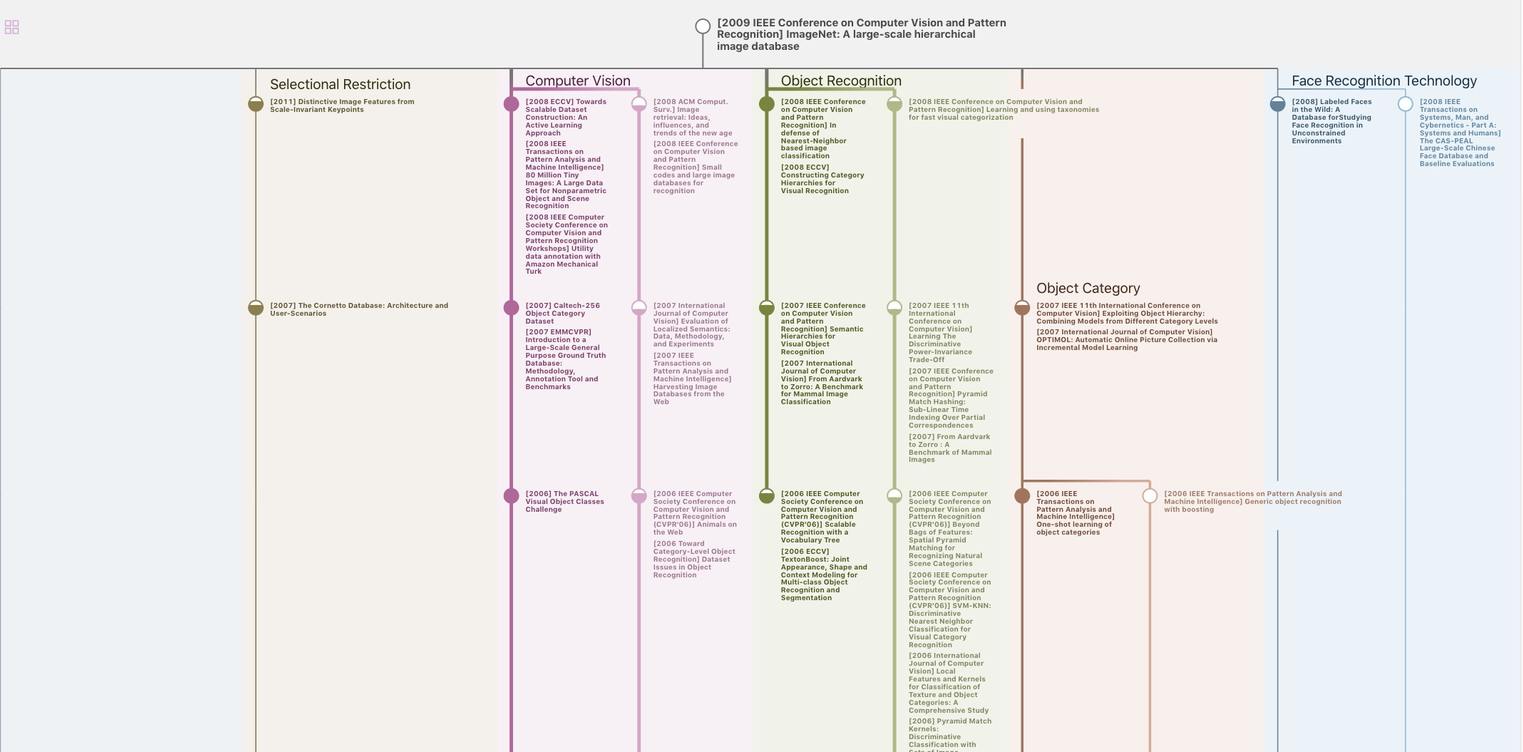
生成溯源树,研究论文发展脉络
Chat Paper
正在生成论文摘要