A Comparison of the Efficiency of Using a Deep CNN Approach with Other Common Regression Methods for the Prediction of EGFR Expression in Glioblastoma Patients
Journal of Digital Imaging(2019)
摘要
To estimate epithermal growth factor receptor (EGFR) expression level in glioblastoma (GBM) patients using radiogenomic analysis of magnetic resonance images (MRI). A comparative study using a deep convolutional neural network (CNN)–based regression, deep neural network, least absolute shrinkage and selection operator (LASSO) regression, elastic net regression, and linear regression with no regularization was carried out to estimate EGFR expression of 166 GBM patients. Except for the deep CNN case, overfitting was prevented by using feature selection, and loss values for each method were compared. The loss values in the training phase for deep CNN, deep neural network, Elastic net, LASSO, and the linear regression with no regularization were 2.90, 8.69, 7.13, 14.63, and 21.76, respectively, while in the test phase, the loss values were 5.94, 10.28, 13.61, 17.32, and 24.19 respectively. These results illustrate that the efficiency of the deep CNN approach is better than that of the other methods, including Lasso regression, which is a regression method known for its advantage in high-dimension cases. A comparison between deep CNN, deep neural network, and three other common regression methods was carried out, and the efficiency of the CNN deep learning approach, in comparison with other regression models, was demonstrated.
更多查看译文
关键词
Radiogenomic, Glioblastoma, Deep learning, Regression
AI 理解论文
溯源树
样例
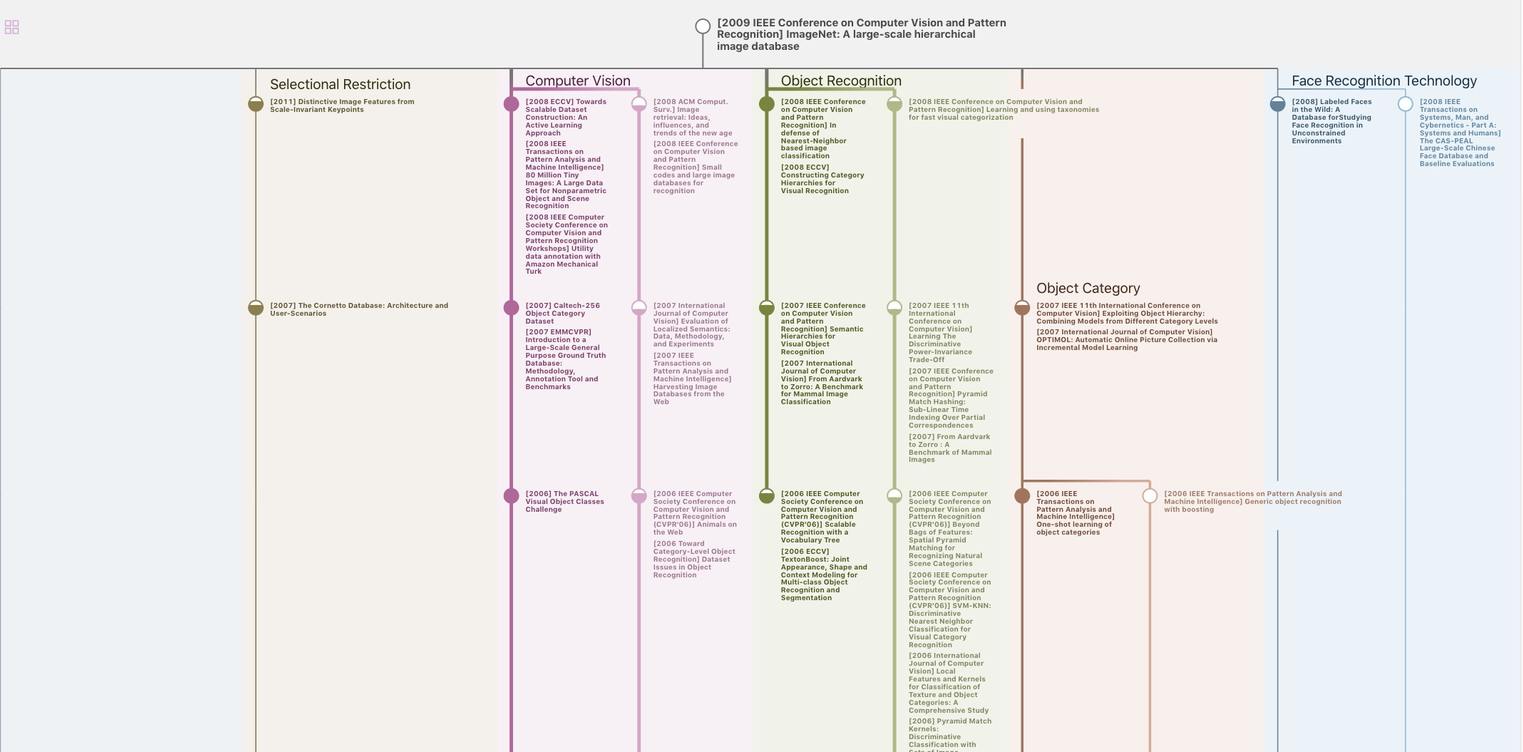
生成溯源树,研究论文发展脉络
Chat Paper
正在生成论文摘要