Regression with Uncertainty Quantification in Large Scale Complex Data.
SMC(2022)
摘要
While several methods for predicting uncertainty on deep networks have been recently proposed, they do not readily translate to large and complex datasets. In this paper we utilize a simplified form of the Mixture Density Networks (MDNs) to produce a one-shot approach to quantify uncertainty in regression problems. We show that our uncertainty bounds are on-par or better than other reported existing methods. When applied to standard regression benchmark datasets, we show an improvement in predictive log-likelihood and root-mean-square-error when compared to existing state-of-the-art methods. We also demonstrate this method's efficacy on stochastic, highly volatile time-series data where stock prices are predicted for the next time interval. The resulting uncertainty graph summarizes significant anomalies in the stock price chart. Furthermore, we apply this method to the task of age estimation from the challenging IMDb-Wiki dataset of half a million face images. We successfully predict the uncertainties associated with the prediction and empirically analyze the underlying causes of the uncertainties. This uncertainty quantification can be used to pre-process low quality datasets and further enable learning.
更多查看译文
关键词
age estimation,anomaly detection,complex datasets,complex video segments,data cleansing,deep networks,face images,future stock prices,IMDb-Wiki dataset,large scale complex data,MDN,mixture density networks,predictive log-likelihood,quantity uncertainty,regression benchmark datasets,root-mean-square-error,time-series data,uncertainty quantification
AI 理解论文
溯源树
样例
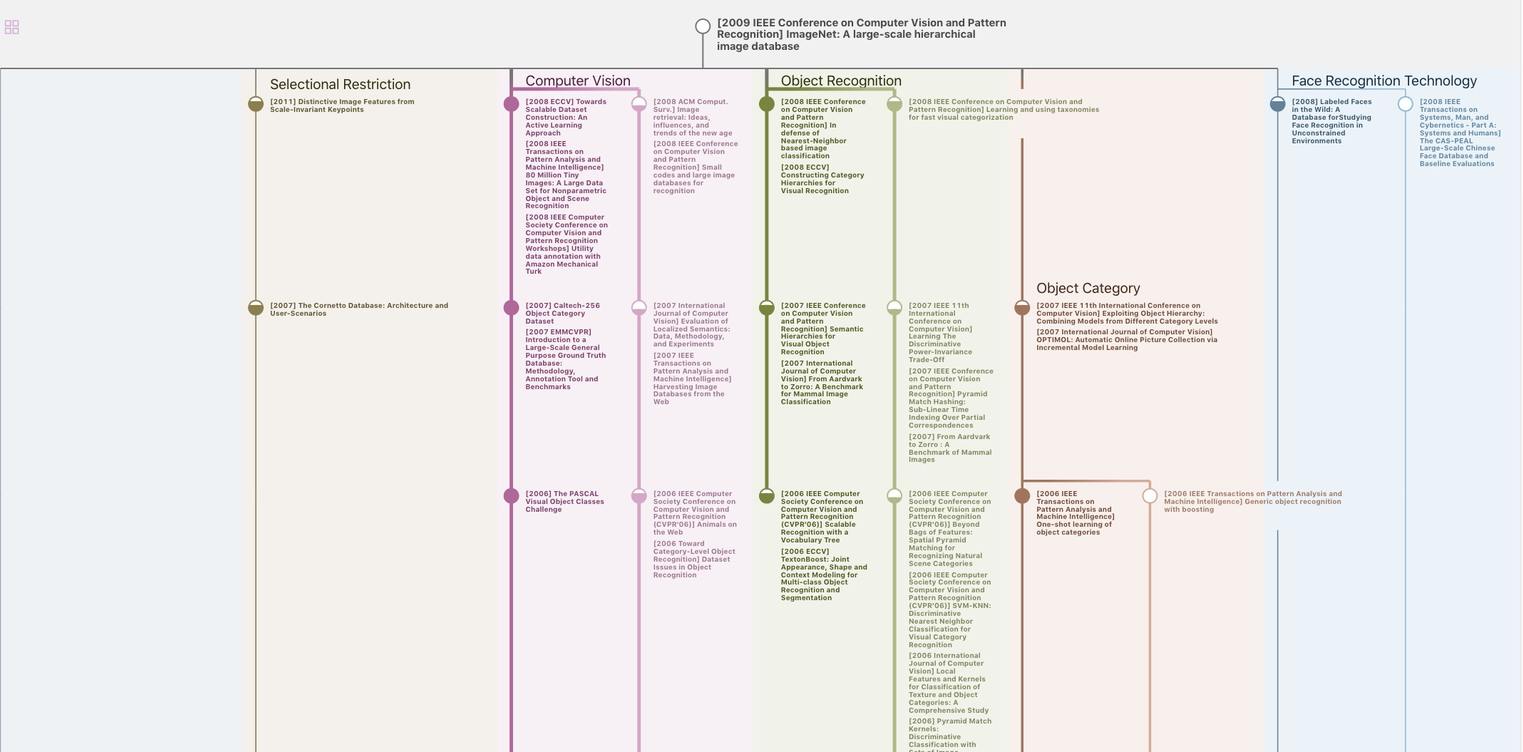
生成溯源树,研究论文发展脉络
Chat Paper
正在生成论文摘要