Deep Distributional Sequence Embeddings Based on a Wasserstein Loss
NEURAL PROCESSING LETTERS(2022)
摘要
Deep metric learning employs deep neural networks to embed instances into a metric space such that distances between instances of the same class are small and distances between instances from different classes are large. In most existing deep metric learning techniques, the embedding of an instance is given by a feature vector produced by a deep neural network and Euclidean distance or cosine similarity defines distances between these vectors. This paper studies deep distributional embeddings of sequences, where the embedding of a sequence is given by the distribution of learned deep features across the sequence. The motivation for this is to better capture statistical information about the distribution of patterns within the sequence in the embedding. When embeddings are distributions rather than vectors, measuring distances between embeddings involves comparing their respective distributions. The paper therefore proposes a distance metric based on Wasserstein distances between the distributions and a corresponding loss function for metric learning, which leads to a novel end-to-end trainable embedding model. We empirically observe that distributional embeddings outperform standard vector embeddings and that training with the proposed Wasserstein metric outperforms training with other distance functions.
更多查看译文
关键词
Metric learning, Sequence embeddings, Deep learning
AI 理解论文
溯源树
样例
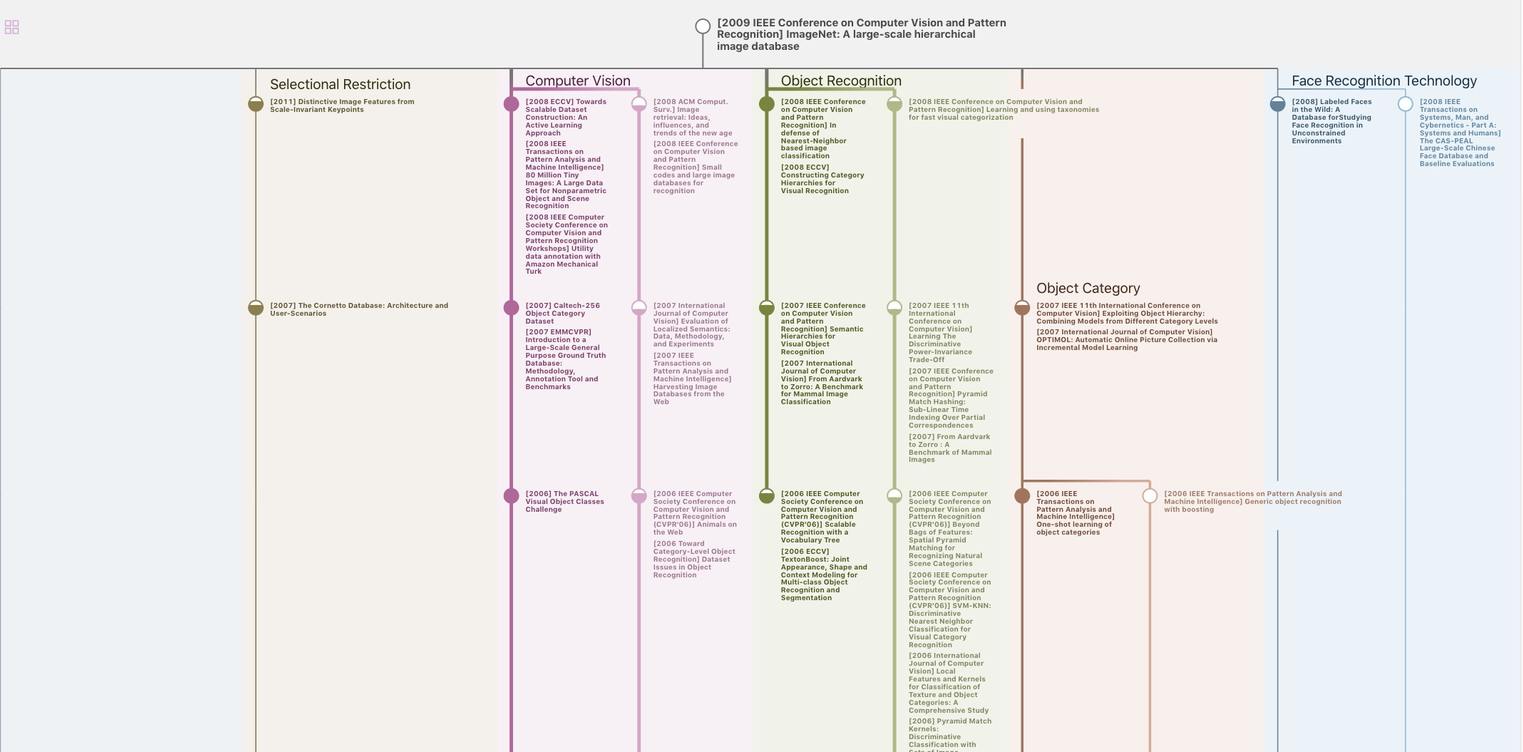
生成溯源树,研究论文发展脉络
Chat Paper
正在生成论文摘要