It GAN Do Better: GAN-Based Detection of Objects on Images With Varying Quality
IEEE TRANSACTIONS ON IMAGE PROCESSING(2021)
摘要
In this paper, we propose a novel generative framework which uses Generative Adversarial Networks (GANs) to generate features that provide robustness for object detection on reduced-quality images. The proposed GAN-based Detection of Objects (GAN-DO) framework is not restricted to any particular architecture and can be generalized to several deep neural network (DNN) based architectures. The resulting deep neural network maintains the exact architecture as the selected baseline model without adding to the model parameter complexity or inference speed. We first evaluate the effect of image quality on both object classification and object bounding box regression. We then test the models resulting from our proposed GAN-DO framework, using two state-of-the-art object detection architectures as the baseline models. We also evaluate the effect of the number of re-trained parameters in the generator of GAN-DO on the accuracy of the final trained model. Performance results provided using GAN-DO on object detection datasets establish an improved robustness to varying image quality and a higher mAP compared to the existing approaches.
更多查看译文
关键词
Object detection, Training, Image quality, Computational modeling, Task analysis, Generators, Distortion, Object detection, GAN, generator, discriminator, image quality, fine-tuning, SSD, RetinaNet
AI 理解论文
溯源树
样例
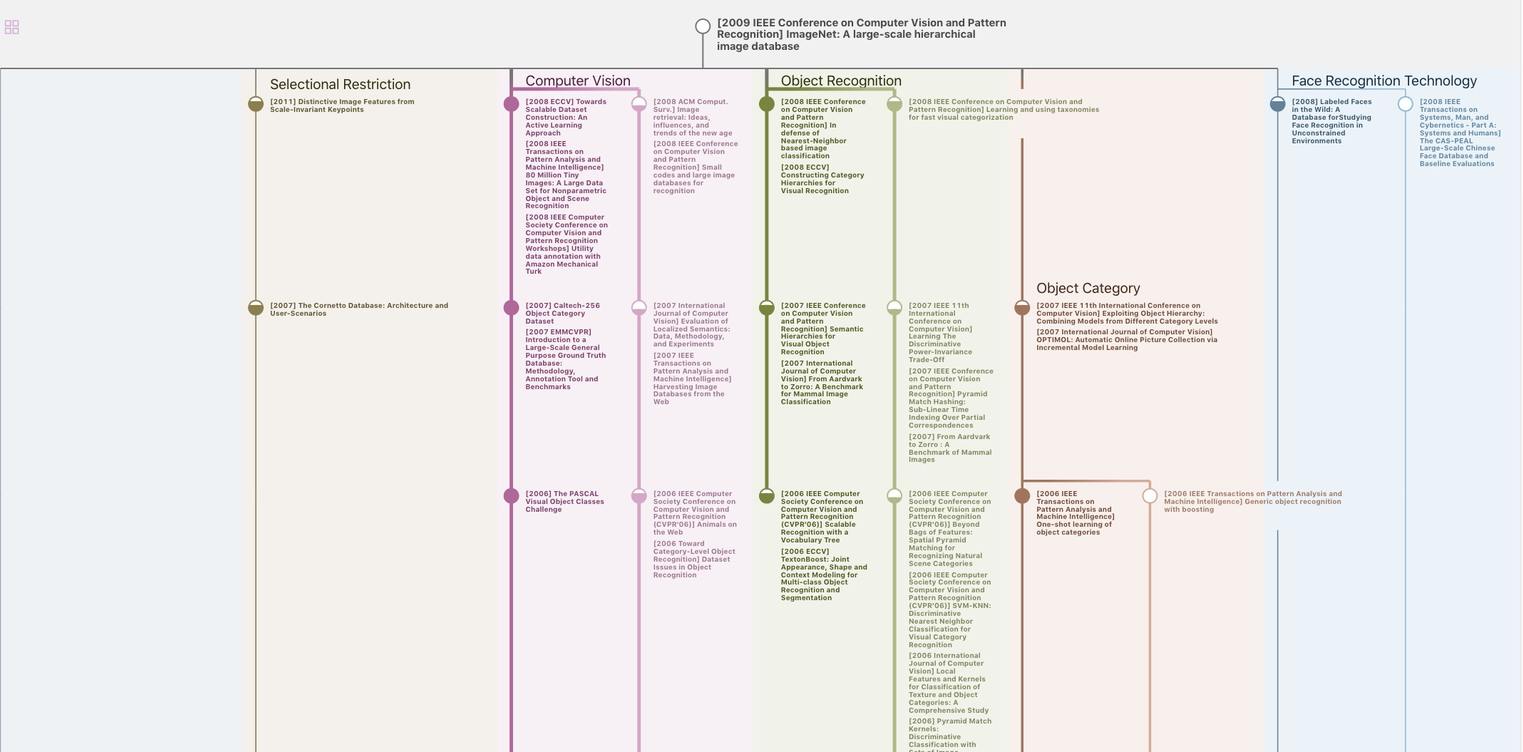
生成溯源树,研究论文发展脉络
Chat Paper
正在生成论文摘要