Joint Graph-based Depth Refinement and Normal Estimation
CVPR(2020)
摘要
Depth estimation is an essential component in understanding the 3D geometry of a scene, with numerous applications in urban and indoor settings. These scenes are characterized by a prevalence of human made structures, which in most of the cases, are either inherently piece-wise planar, or can be approximated as such. In these settings, we devise a novel depth refinement framework that aims at recovering the underlying piece-wise planarity of the inverse depth map. We formulate this task as an optimization problem involving a data fidelity term that minimizes the distance to the input inverse depth map, as well as a regularization that enforces a piece-wise planar solution. As for the regularization term, we model the inverse depth map as a weighted graph between pixels. The proposed regularization is designed to estimate a plane automatically at each pixel, without any need for an a priori estimation of the scene planes, and at the same time it encourages similar pixels to be assigned to the same plane. The resulting optimization problem is efficiently solved with ADAM algorithm. Experiments show that our method leads to a significant improvement in depth refinement, both visually and numerically, with respect to state-of-the-art algorithms on Middlebury, KITTI and ETH3D multi-view stereo datasets.
更多查看译文
关键词
ETH3D multiview datasets,KITTI,Middlebury,ADAM solver,weighted graph,inverse depth map pixels,regularization term,piece-wise planar solution,noisy input,data fidelity term,piece-wise planar scenes,inverse depth maps,underlying piece-wise planarity,depth refinement framework,inherently piece-wise planar,human made structures,indoor settings,urban settings,depth estimation,normal estimation,joint graph-based depth refinement,strongly connected pixels,scene planes
AI 理解论文
溯源树
样例
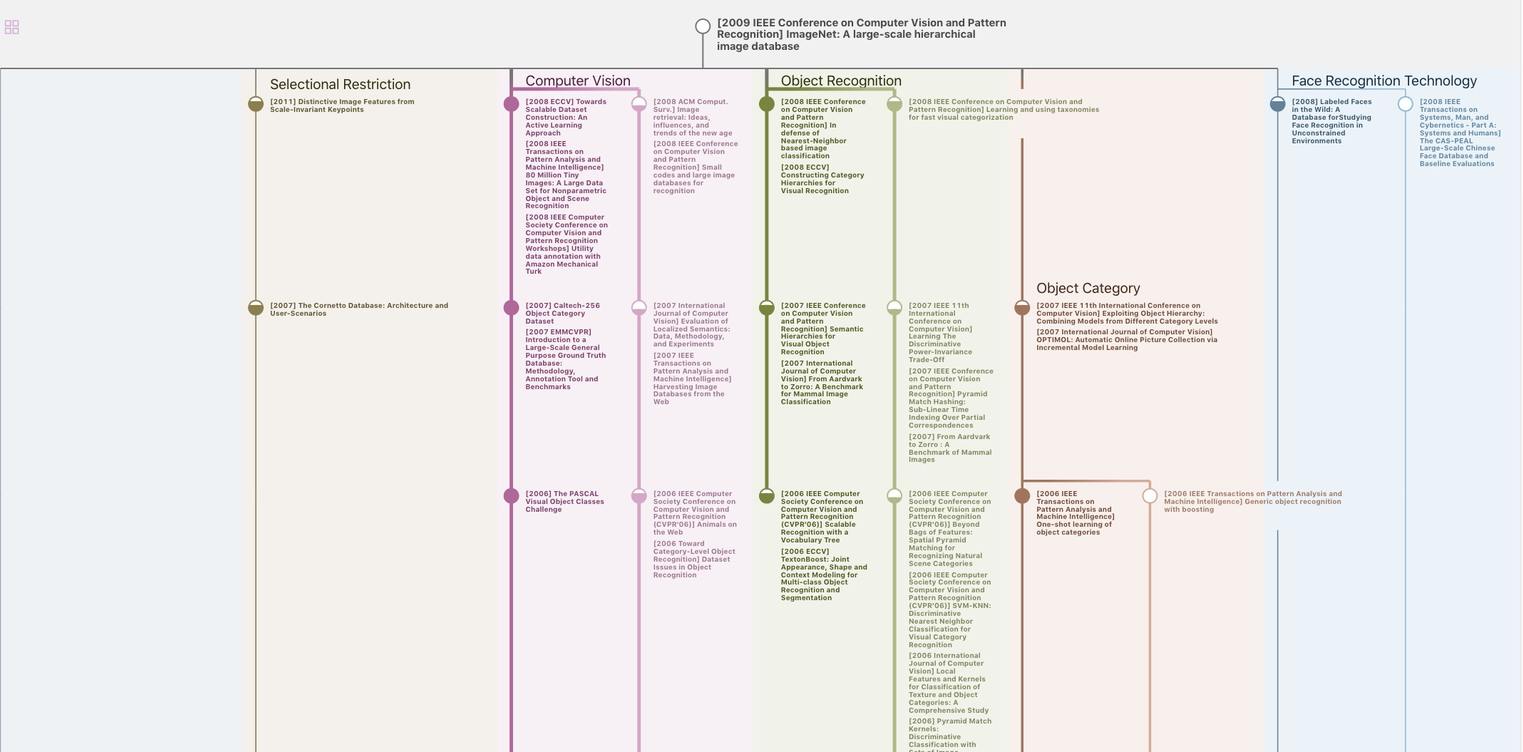
生成溯源树,研究论文发展脉络
Chat Paper
正在生成论文摘要