On Distance and Kernel Measures of Conditional Dependence
JOURNAL OF MACHINE LEARNING RESEARCH(2023)
摘要
Measuring conditional dependence is one of the important tasks in statistical inference and is fundamental in causal discovery, feature selection, dimensionality reduction, Bayesian network learning, and others. In this work, we explore the connection between conditional dependence measures induced by distances on a metric space and reproducing kernels associated with a reproducing kernel Hilbert space (RKHS). For certain distance and kernel pairs, we show the distance-based conditional dependence measures to be equivalent to that of kernel-based measures. On the other hand, we also show that some popular kernel conditional dependence measures based on the Hilbert-Schmidt norm of a certain cross-conditional covariance operator, do not have a simple distance representation, except in some limiting cases.
更多查看译文
关键词
Conditional independence test,distance covariance,energy distance,Hilbert-Schmidt independence criterion,reproducing kernel Hilbert space
AI 理解论文
溯源树
样例
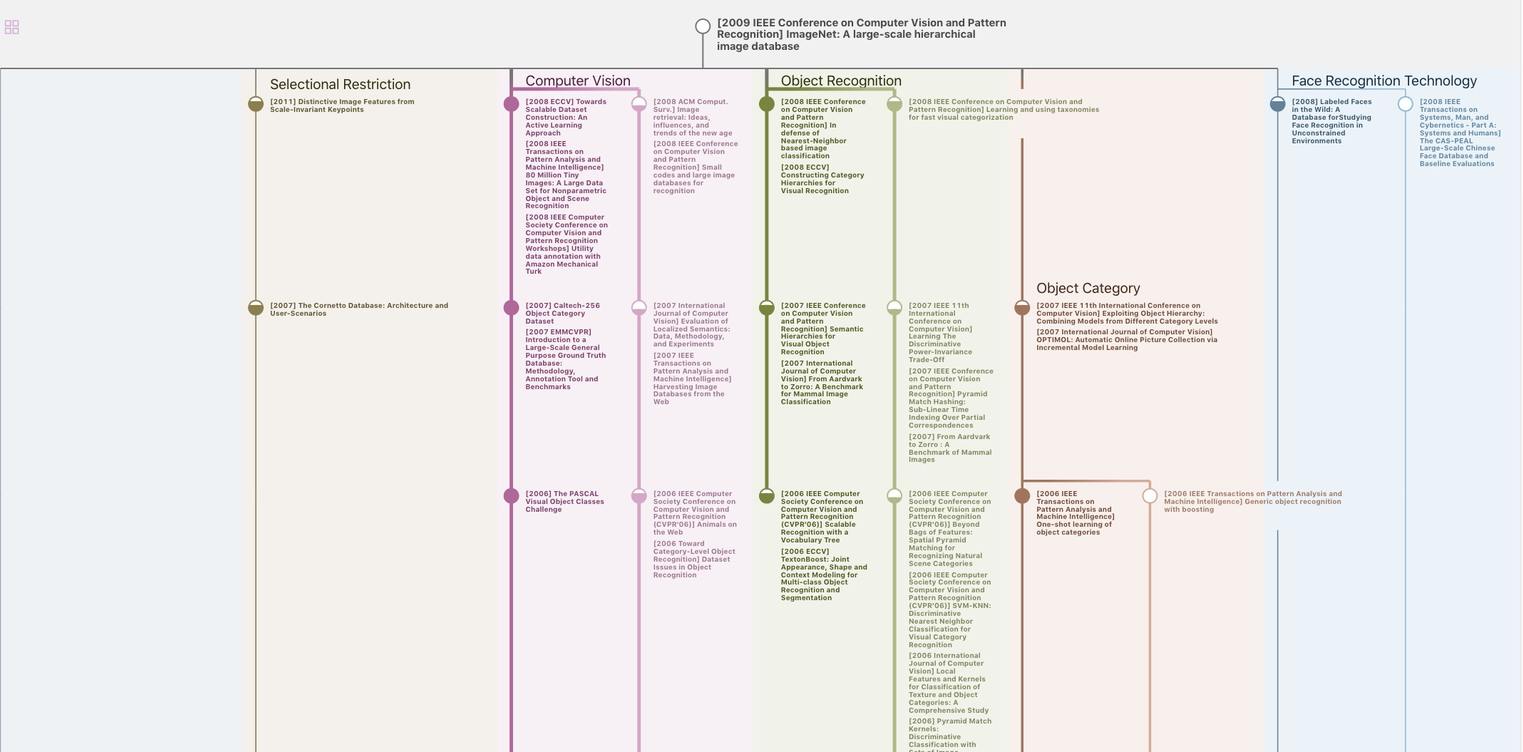
生成溯源树,研究论文发展脉络
Chat Paper
正在生成论文摘要