A hybrid deep learning framework for bacterial named entity recognition with domain features
BMC Bioinformatics(2019)
摘要
Background Microbes have been shown to play a crucial role in various ecosystems. Many human diseases have been proved to be associated with bacteria, so it is essential to extract the interaction between bacteria for medical research and application. At the same time, many bacterial interactions with certain experimental evidences have been reported in biomedical literature. Integrating this knowledge into a database or knowledge graph could accelerate the progress of biomedical research. A crucial and necessary step in interaction extraction (IE) is named entity recognition (NER). However, due to the specificity of bacterial naming, there are still challenges in bacterial named entity recognition. Results In this paper, we propose a novel method for bacterial named entity recognition, which integrates domain features into a deep learning framework combining bidirectional long short-term memory network and convolutional neural network. When domain features are not added, F1-measure of the model achieves 89.14%. After part-of-speech (POS) features and dictionary features are added, F1-measure of the model achieves 89.7%. Hence, our model achieves an advanced performance in bacterial NER with the domain features. Conclusions We propose an efficient method for bacterial named entity recognition which combines domain features and deep learning models. Compared with the previous methods, the effect of our model has been improved. At the same time, the process of complex manual extraction and feature design are significantly reduced.
更多查看译文
关键词
Named entity recognition, Biomedical text mining, Conditional random field, Deep learning
AI 理解论文
溯源树
样例
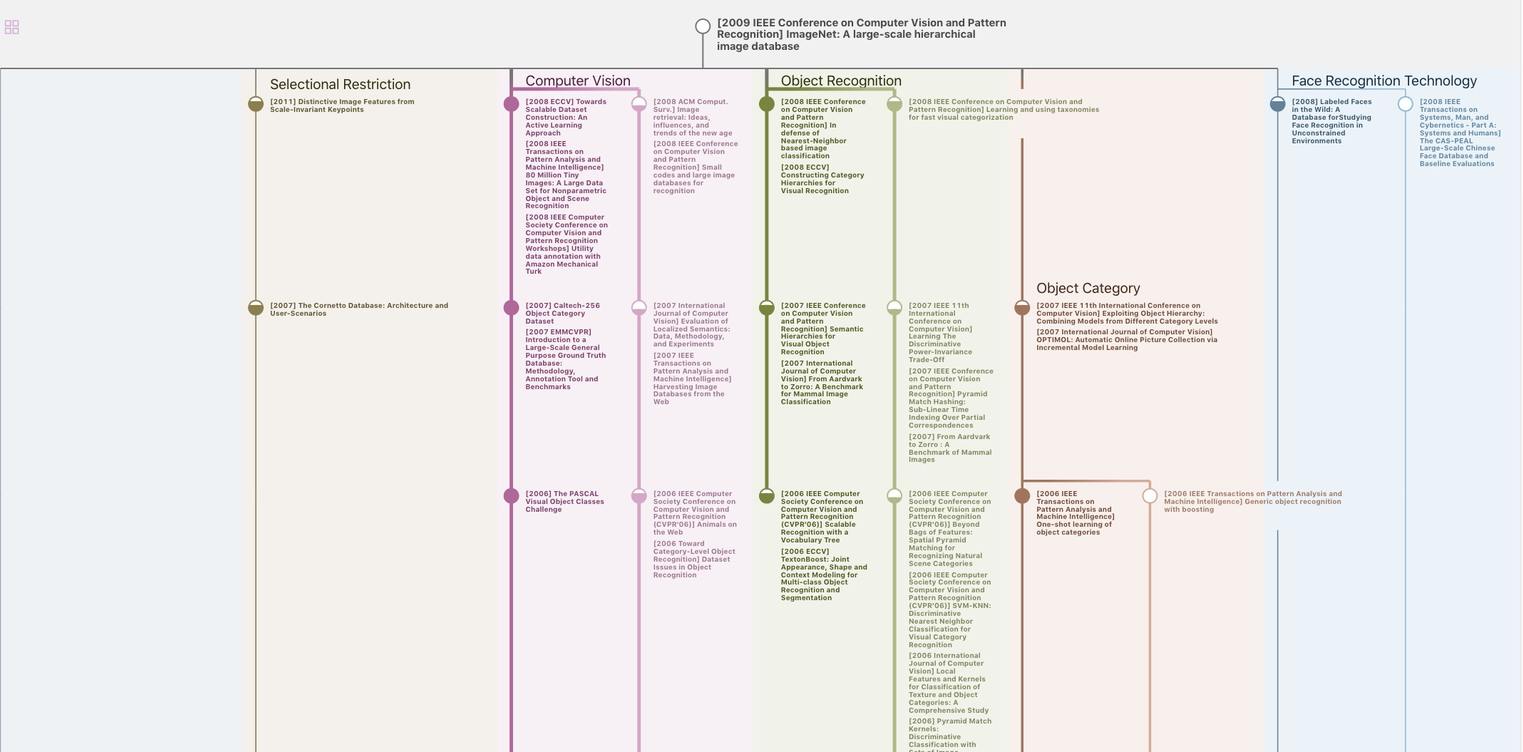
生成溯源树,研究论文发展脉络
Chat Paper
正在生成论文摘要