Opportunistic Spectrum Access In Cognitive Radio For Tactical Network
2018 2ND EUROPEAN CONFERENCE ON ELECTRICAL ENGINEERING AND COMPUTER SCIENCE (EECS 2018)(2018)
摘要
This manuscript addresses the problem of Opportunistic Spectrum Access (OSA) in Cognitive Radio Networks (CRNs). In the context of CRN, two types of users may coexist: primary users (PU), i.e. licensed user and the secondary user (SU), i.e. opportunistic user who is willing to transmit over the licensed bandwidth of PU. The main aim of a SU is to maximize his transmission rate. Hence, he must target the best channels, i.e. the must available one with the best performance. To estimate the availability of channels, one can use the Multi-Armed Bandit (MAB) learning algorithms such as (Thompson Sampling, Upper Confidence Bound, and e-greedy, etc.). Generally, these algorithms are proposed to handle the simple case of a single SU. MAB algorithms are composed of two stages: exploration and exploitation. In this study, we consider the multi-user case where a novel policy is proposed to manage the CRN network. In our study, we consider the case where the SUs have different ranks and transmission priorities. To evaluate our policy, we use the parameter regret which is the reward loss between the maximum reward getting from the best channel and the actual reward got from selecting sub -optimal channels (i.e. less vacant ones). Our simulations show that the proposed policy achieves better results than some known policies such as Random Rank or SL(K) (Selective learning of the k-th largest expected rewards) policies.
更多查看译文
关键词
Cognitive Radio, Learning Algorithms, Tactical Radio, Dynamic Spectrum Access, Opportunistic Spectrum Access, Decentralized network, Priority Access
AI 理解论文
溯源树
样例
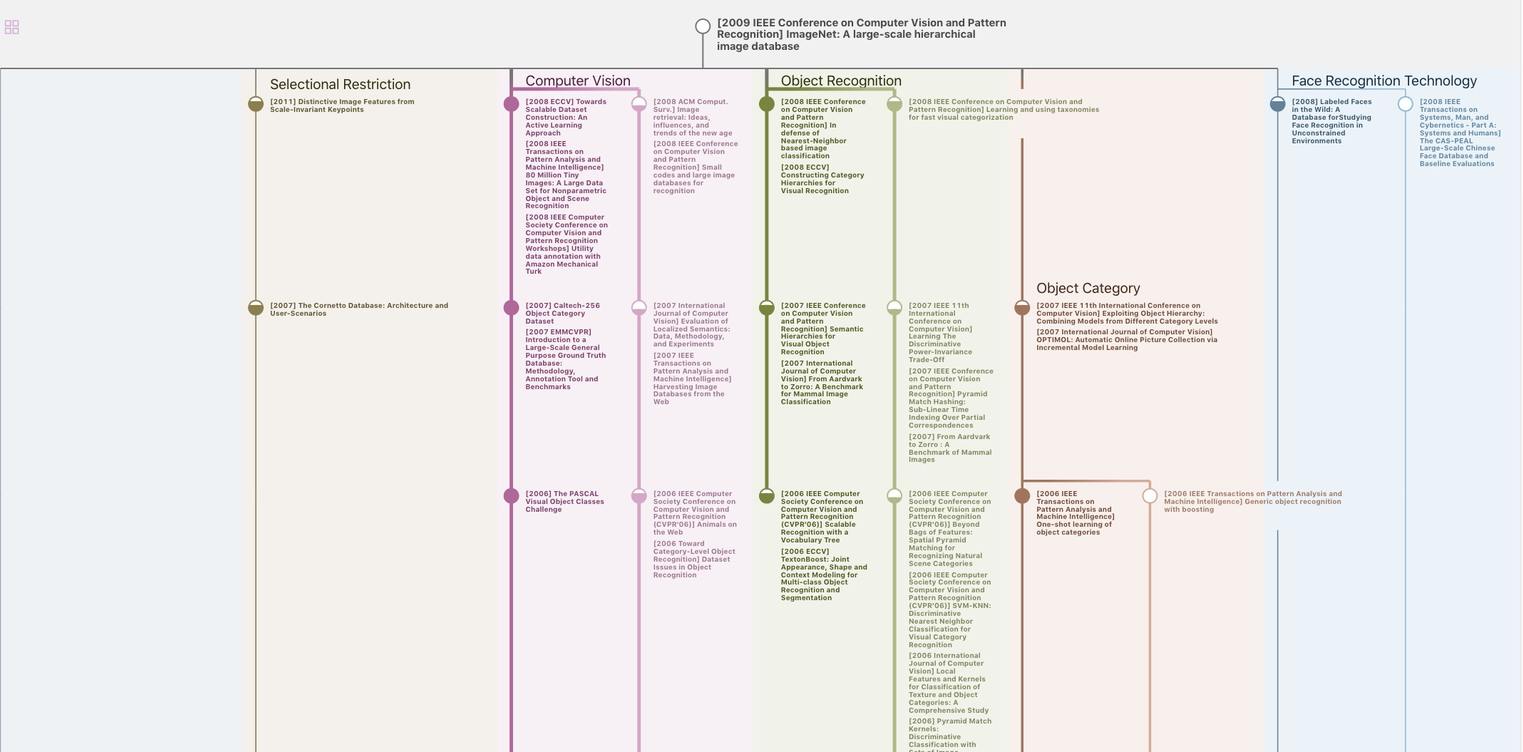
生成溯源树,研究论文发展脉络
Chat Paper
正在生成论文摘要