Multi-source data fusion for aspect-level sentiment classification.
Knowledge-Based Systems(2020)
摘要
Neural networks have achieved great success in aspect-level sentiment classification due to their ability to learn sentiment knowledge from text. Generally, the effectiveness of neural networks relies on sufficiently large training corpora. However, existing aspect-level corpora are relatively small, which greatly limits the performance of neural network-based systems. In this paper, we propose a novel approach to aspect-level sentiment classification based on multi-source data fusion, which allows our system to learn sentiment knowledge from different types of resources. Specifically, we design a unified framework to integrate data from aspect-level corpora, sentence-level corpora, and word-level sentiment lexicons. Moreover, we take advantage of BERT, a pre-trained language model based on deep bidirectional Transformers, to generate aspect-specific sentence representations for sentiment classification. We evaluate our approach using laptop and restaurant datasets from SemEval 2014. Experimental results show that our approach consistently outperforms the state-of-the-art methods on all datasets.
更多查看译文
关键词
Sentiment analysis,Neural networks,Data fusion
AI 理解论文
溯源树
样例
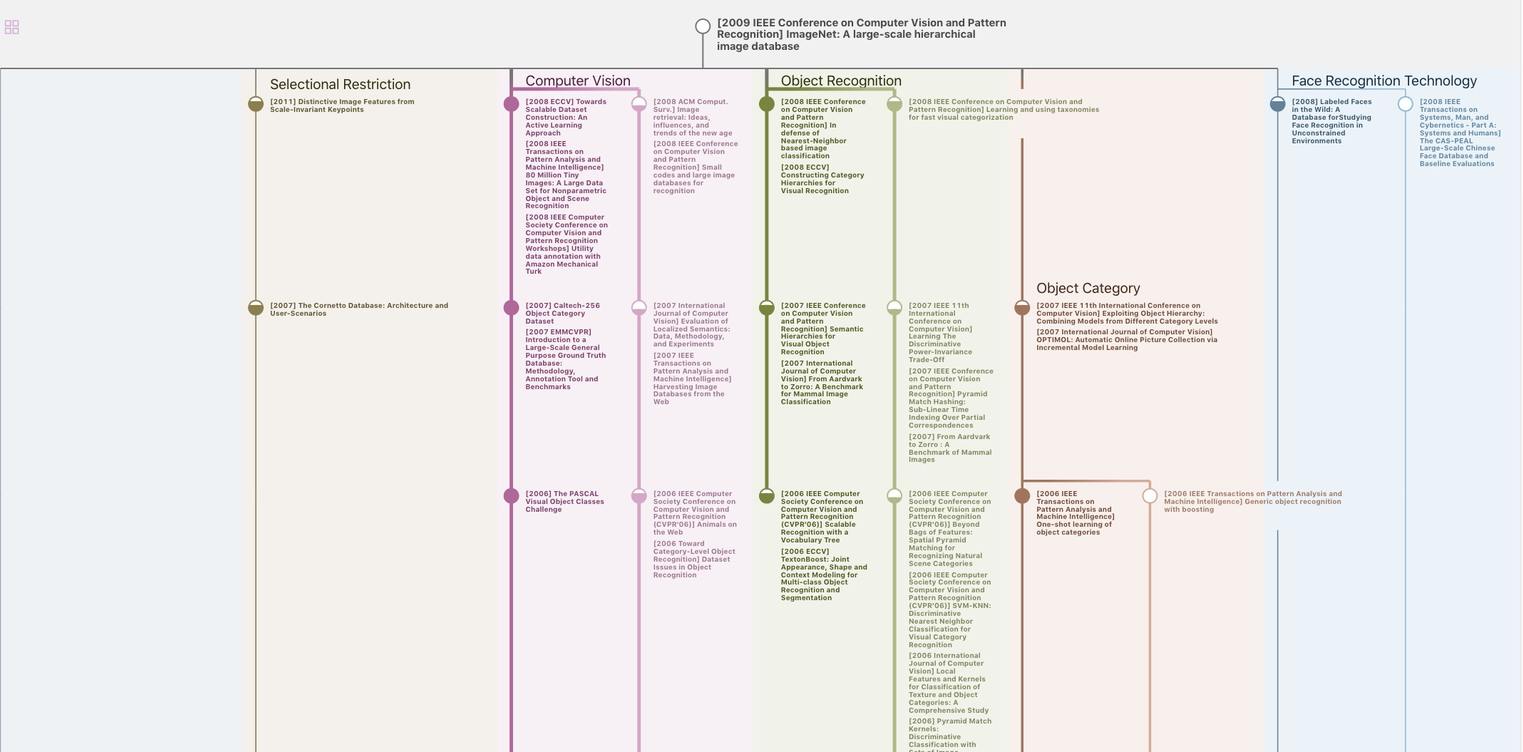
生成溯源树,研究论文发展脉络
Chat Paper
正在生成论文摘要