Low-rank graph preserving discriminative dictionary learning for image recognition.
Knowledge-Based Systems(2020)
摘要
Discriminative dictionary learning plays a key role in sparse representation-based classification. In this paper, we propose a low-rank graph preserving discriminative dictionary learning (LRGPDDL) method for sparse representation-based image recognition. Specifically, we learn a common sub-dictionary as well as several class-specific sub-dictionaries to explicitly capture the common information shared by all the classes and the class-specific information belonging to the corresponding class. We also impose a low-rank constraint on each sub-dictionary to weaken the negative influence from noise contained in training samples. A discriminative graph preserving criterion and a discriminative reconstruction error term are used for exploiting discriminative information, which can improve the discriminative ability of the learned dictionary effectively. In addition, an incoherence term is also introduced into the proposed dictionary learning model to encourage the learned sub-dictionaries to be as independent as possible. Experimental results on several image datasets verify the effectiveness and robustness of LRGPDDL.
更多查看译文
关键词
Low-rank,Graph preserving,Dictionary learning,Sparse representation,Image recognition
AI 理解论文
溯源树
样例
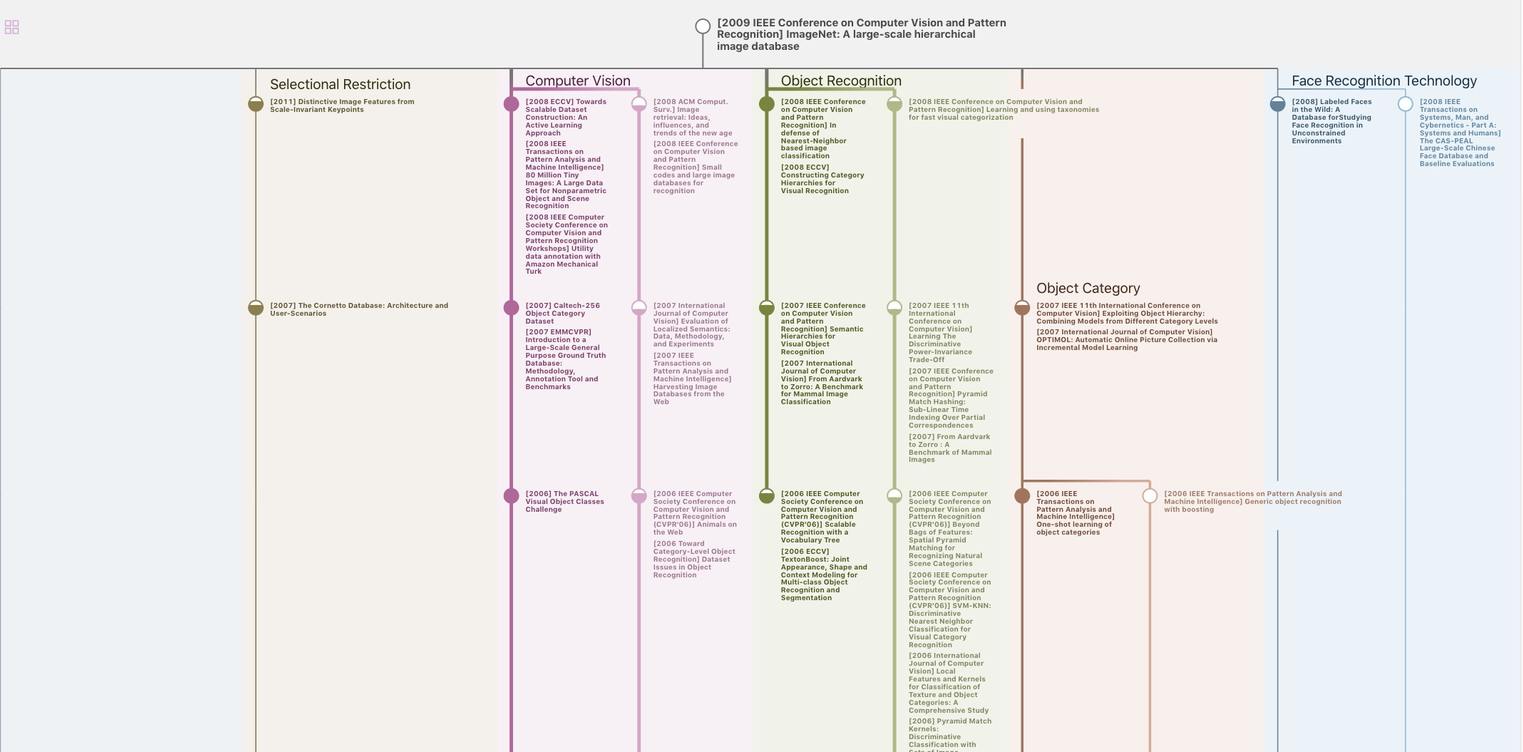
生成溯源树,研究论文发展脉络
Chat Paper
正在生成论文摘要