Accuracy of Longitudinal Assessment of Visceral Adipose Tissue by Dual-Energy X-Ray Absorptiometry in Children with Obesity.
JOURNAL OF OBESITY(2019)
摘要
Background. Increased visceral adipose tissue (VAT) is strongly associated with cardiometabolic risk factors. Accurate quantification of VAT is available through magnetic resonance imaging (MRI), which incurs a significant financial and time burden. We aimed to assess the accuracy of dual-energy X-ray absorptiometry- (DXA-) derived VAT (DXA-VAT) against a gold standard MRI protocol (MRI-VAT) in children with normal weight and obesity cross-sectionally, and over the course of a lifestyle intervention. Methodology. MRI-VAT and DXA-VAT were quantified in 61 children (30 normal weight and 31 with obesity) at baseline. Children with obesity entered a three-month exercise and/or nutrition intervention after which VAT was reassessed. MRI- and DXA-VAT cross-sectional area, volume, and mass were quantified, and associations were calculated at baseline (n = 61) and pre-post intervention (n = 28, 3 participants dropped out). Method agreement was assessed through Bland-Altman analysis, linear regression, and Passing-Bablok regression. Results. At baseline, all DXA- and MRI-VAT outcomes were strongly associated (r = 0.90, P<0.001). However, there were no significant associations between absolute or relative change in DXA- and MRI-VAT outcomes (r = 0.25-0.36, P>0.05). DXA significantly overestimated VAT CSA (cross-sectional area), volume, and mass when compared with MRI (P<0.001) at baseline. Significant proportional bias was observed for all DXA-VAT outcomes at baseline and for relative longitudinal changes in DXA-VAT. Conclusions. Although DXA-VAT outcomes were strongly associated with MRI-VAT outcomes at baseline, estimates were subject to proportional bias in children with obesity and normal weight. DXA lacks validity for detecting changes in VAT among children with obesity. This trial is registered with NCT01991106.
更多查看译文
AI 理解论文
溯源树
样例
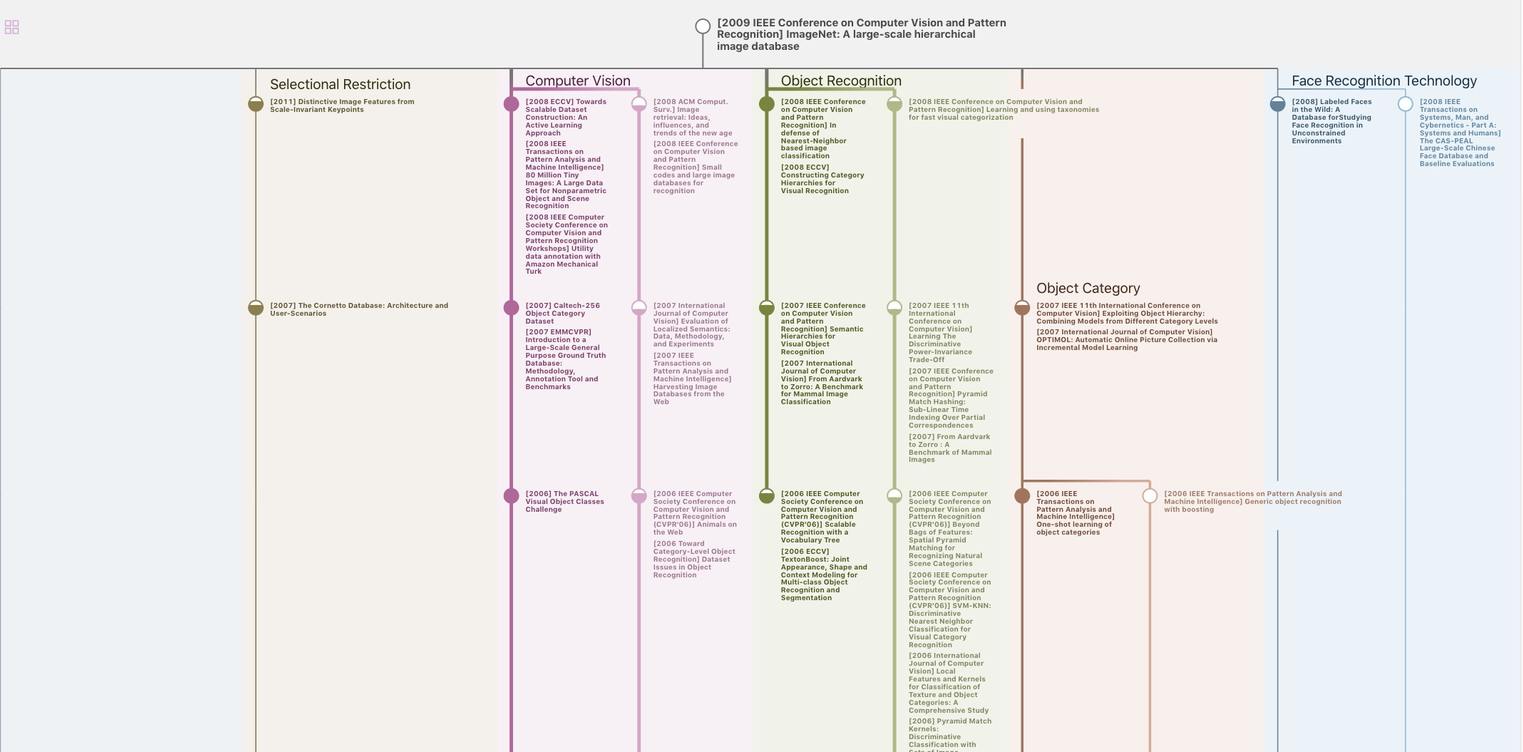
生成溯源树,研究论文发展脉络
Chat Paper
正在生成论文摘要