Swarm Optimization Algorithm For Road Bypass Extrapolation
ADVANCES IN VISUAL COMPUTING, ISVC 2019, PT I(2020)
摘要
Ant Colony Optimization (ACO) algorithms work by lever-aging a population of agents that communicate through interaction with deposited "pheromone," and have been applied in various configurations to the long-standing problem of identifying trafficable terrain from aerial imagery. While these approaches have proven successful in highlighting paved roads in urban, highly-developed sites, they tend to fail in periurban and rural locations due to the lower frequency of unnatural features. In this work, we describe a workflow that uses site-specific, near-infrared and first-return LIDAR data to predict the "accessible space" of an image-i.e., the more open regions with shallow elevation gradient that may be readily traversible by both mounted (e.g., all-terrain vehicles) and dismounted forces. Collectively, these regions are supplied as input to an ACO algorithm, modified so that the agents perform a random walk weighted by local elevation change, which allows for a more comprehensive exploration of increasingly featureless imaged terrain. Performance of this workflow is evaluated using two study sites in the continental United States: the Muscatatuck Urban Training Center in rural Indiana, and Camp Shelby in Mississippi. Comparison of results with ground-truth datasets show a high degree of success in predicting areas trafficable by a wide variety of mobile units.
更多查看译文
关键词
Image analysis, Swarm optimization, Trafficability
AI 理解论文
溯源树
样例
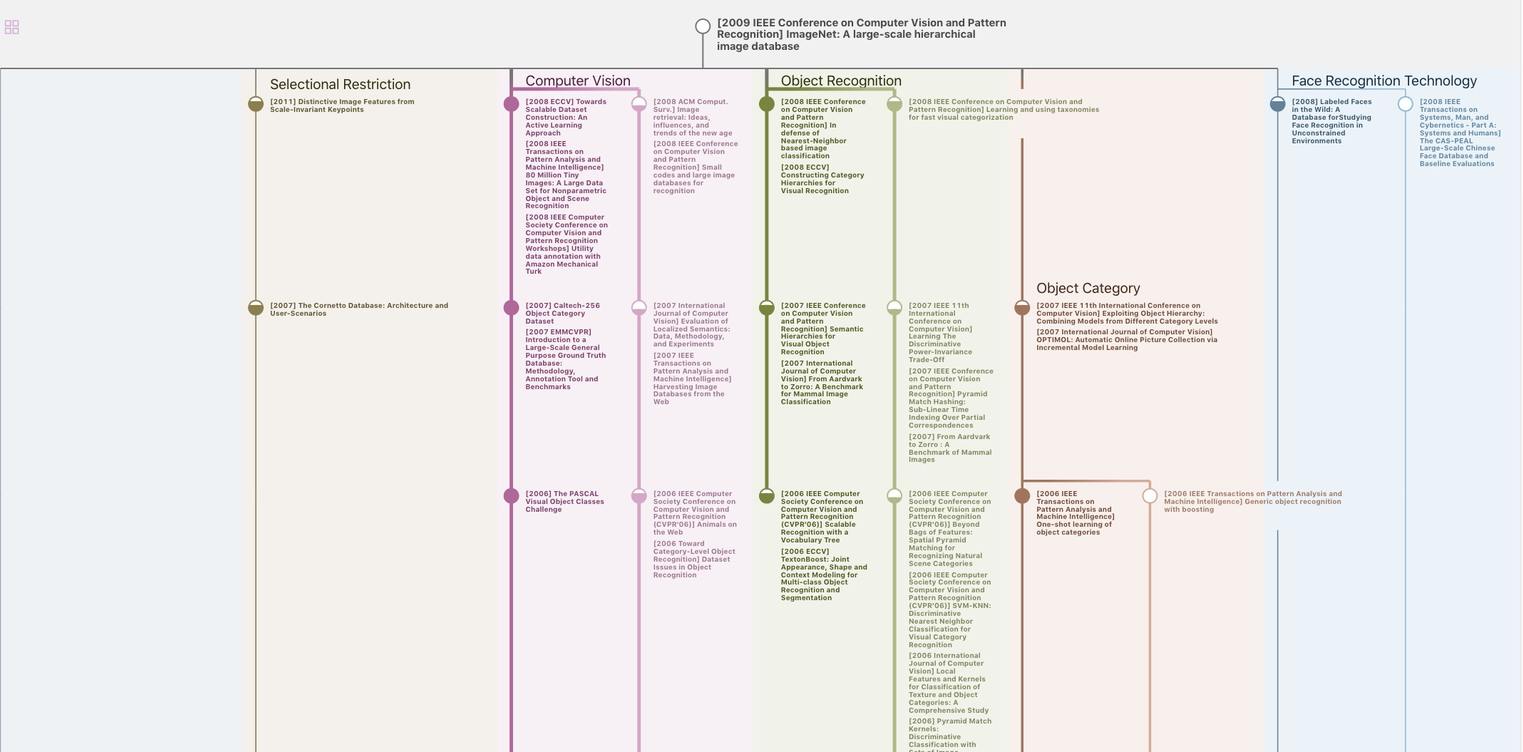
生成溯源树,研究论文发展脉络
Chat Paper
正在生成论文摘要