A Simple and Robust Attentional Encoder-Decoder Model for License Plate Recognition
PRCV (1)(2019)
摘要
Recognizing car license plates in natural scene images is an important yet challenging task in intelligent transport systems. Most of current methods perform well for license plates collected under constrained conditions [ 1 ], e.g., from a single region with specific patterns or shot in front-view with nearly horizontal position. In this work, we propose a simple yet robust approach for license plate recognition under complex conditions. It is composed of an off-the-shelf Xception module and 2-dimension attention based image to sequence learning framework. Despite its simplicity, the proposed model can recognize license plates under various scenarios, including license plates captured in dark or strong lighting conditions, from different regions, oriented, distorted, or even blurred. A CycleGAN based method is employed to generate synthetic license plate images with different province characters and under various situations (shadow, darkness, glare, etc.), which enriches the training data largely and improves the recognition capability greatly. The proposed model achieves state-of-the-art recognition performance on various datasets, which demonstrates its effectiveness and robustness.
更多查看译文
关键词
recognition,encoder-decoder
AI 理解论文
溯源树
样例
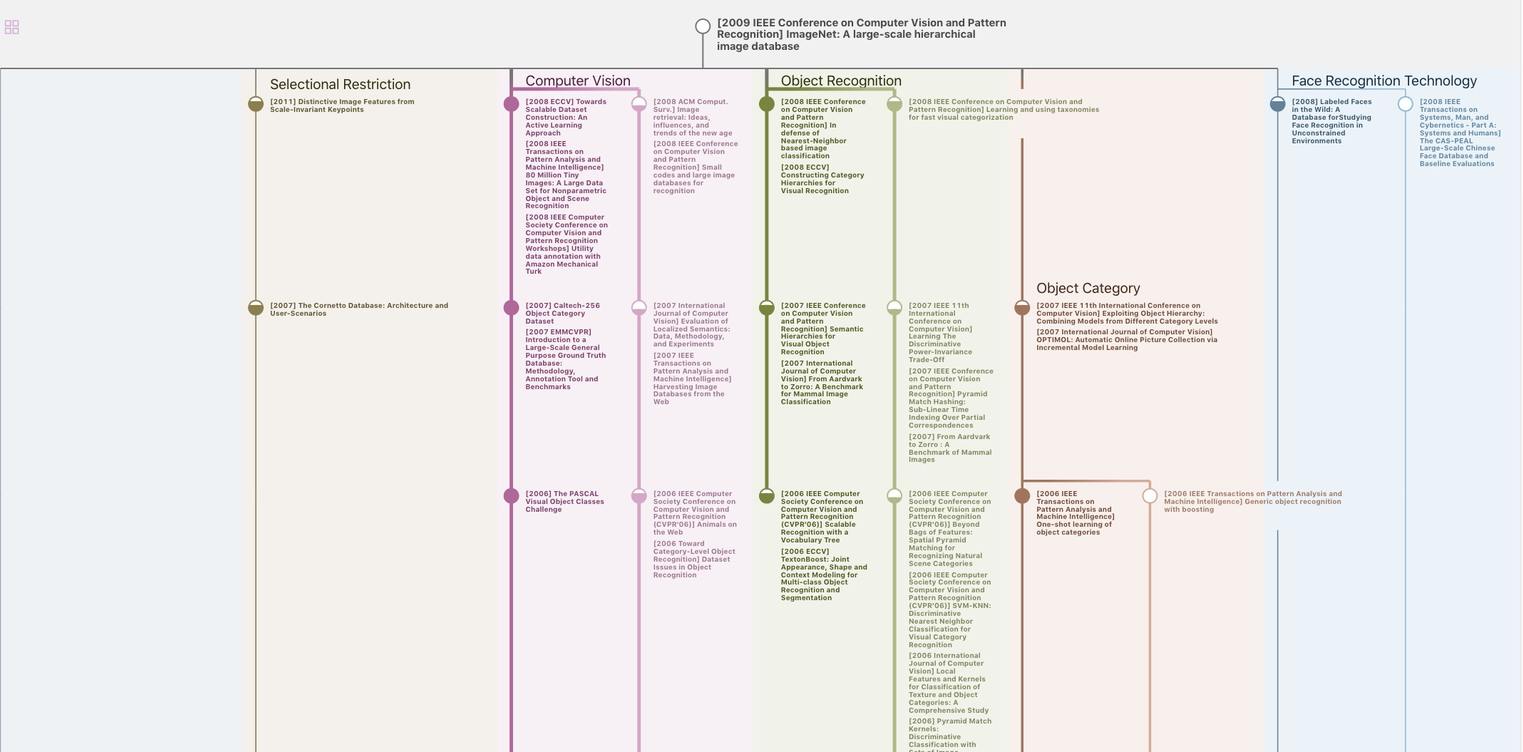
生成溯源树,研究论文发展脉络
Chat Paper
正在生成论文摘要