Patient visit forecasting in an emergency department using a deep neural network approach
KYBERNETES(2020)
摘要
Purpose This study aims to investigate the factors affecting daily demand in an emergency department (ED) and to provide a forecasting tool in a public hospital for horizons of up to seven days. Design/methodology/approach In this study, first, the important factors to influence the demand in EDs were extracted from literature then the relevant factors to the study are selected. Then, a deep neural network is applied to constructing a reliable predictor. Findings Although many statistical approaches have been proposed for tackling this issue, better forecasts are viable by using the abilities of machine learning algorithms. Results indicate that the proposed approach outperforms statistical alternatives available in the literature such as multiple linear regression, autoregressive integrated moving average, support vector regression, generalized linear models, generalized estimating equations, seasonal ARIMA and combined ARIMA and linear regression. Research limitations/implications The authors applied this study in a single ED to forecast patient visits. Applying the same method in different EDs may give a better understanding of the performance of the model to the authors. The same approach can be applied in any other demand forecasting after some minor modifications. Originality/value To the best of the knowledge, this is the first study to propose the use of long short-term memory for constructing a predictor of the number of patient visits in EDs.
更多查看译文
关键词
Patient visit forecasting,Deep neural networks,Long short-term memory,Emergency department
AI 理解论文
溯源树
样例
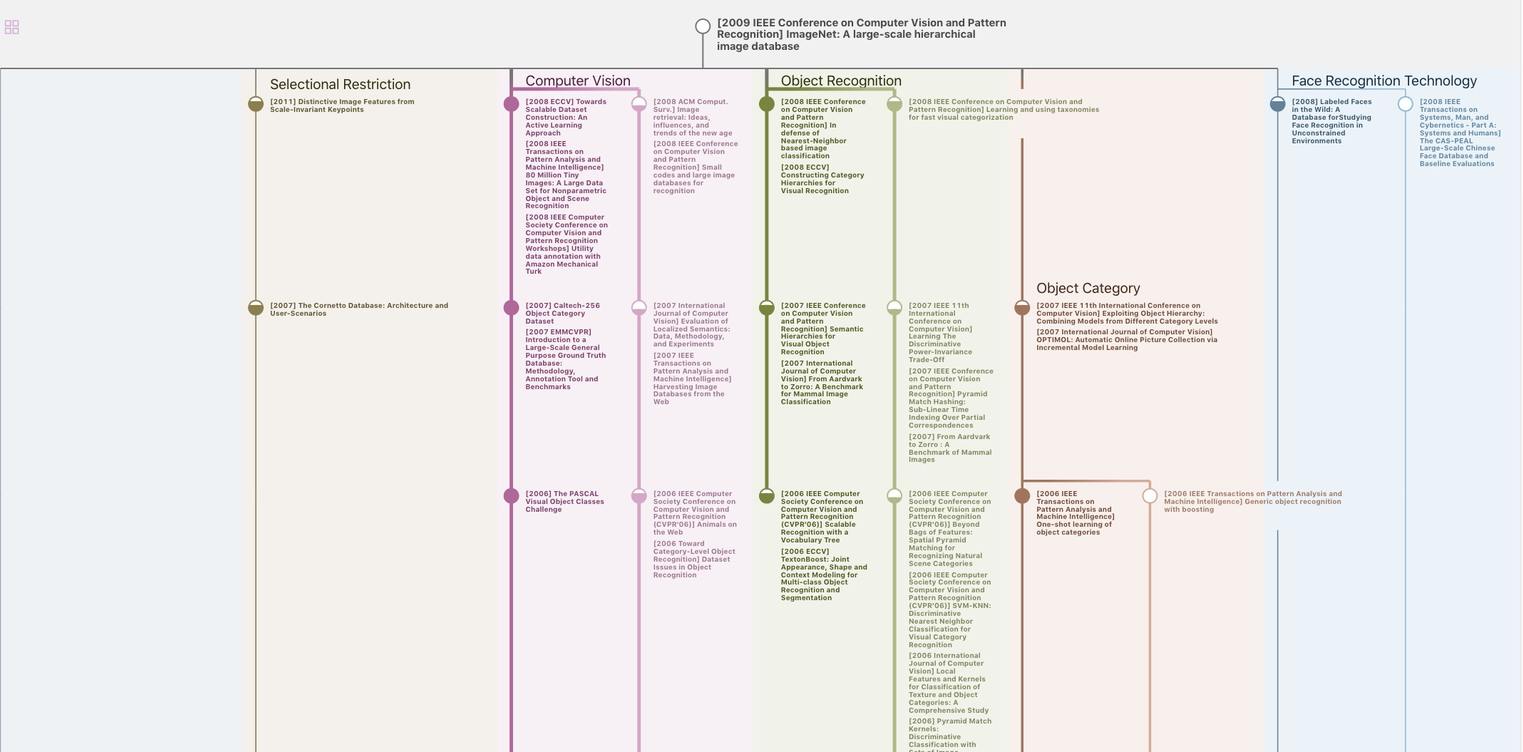
生成溯源树,研究论文发展脉络
Chat Paper
正在生成论文摘要