An energy-aware scheduling algorithm for budget-constrained scientific workflows based on multi-objective reinforcement learning
The Journal of Supercomputing(2019)
摘要
Since scientific workflow scheduling becomes a major energy contributor in clouds, much attention has been paid to reduce the energy consumed by workflows. This paper considers a multi-objective workflow scheduling problem with the budget constraint. Most existing works of budget-constrained workflow scheduling cannot always satisfy the budget constraint and guarantee the feasibility of solutions. Instead, they discuss the success rate in the experiments. Only a few works can always figure out feasible solutions. These methods work, but they are too complicated. In workflow scheduling, it has been a trend to consider more than one objective. However, the weight selection is usually ignored in these works. The inappropriate weights will reduce the quality of solutions. In this paper, we propose an energy-aware multi-objective reinforcement learning (EnMORL) algorithm. We design a much simpler method to ensure the feasibility of solutions. This method is based on the remaining cheapest budget. Reinforcement learning based on the Chebyshev scalarization function is a new framework, which is effective in solving the weight selection problem. Therefore, we design EnMORL based on it. Our goal is to minimize the makespan and energy consumption of the workflow. Finally, we compare EnMORL with two state-of-the-art multi-objective meta-heuristics in the case of four different workflows. The results show that our proposed EnMORL outperforms these existing methods.
更多查看译文
关键词
Scientific workflows,Cloud computing,Energy saving,Reinforcement learning,Multi-objective optimization,The budget constraint
AI 理解论文
溯源树
样例
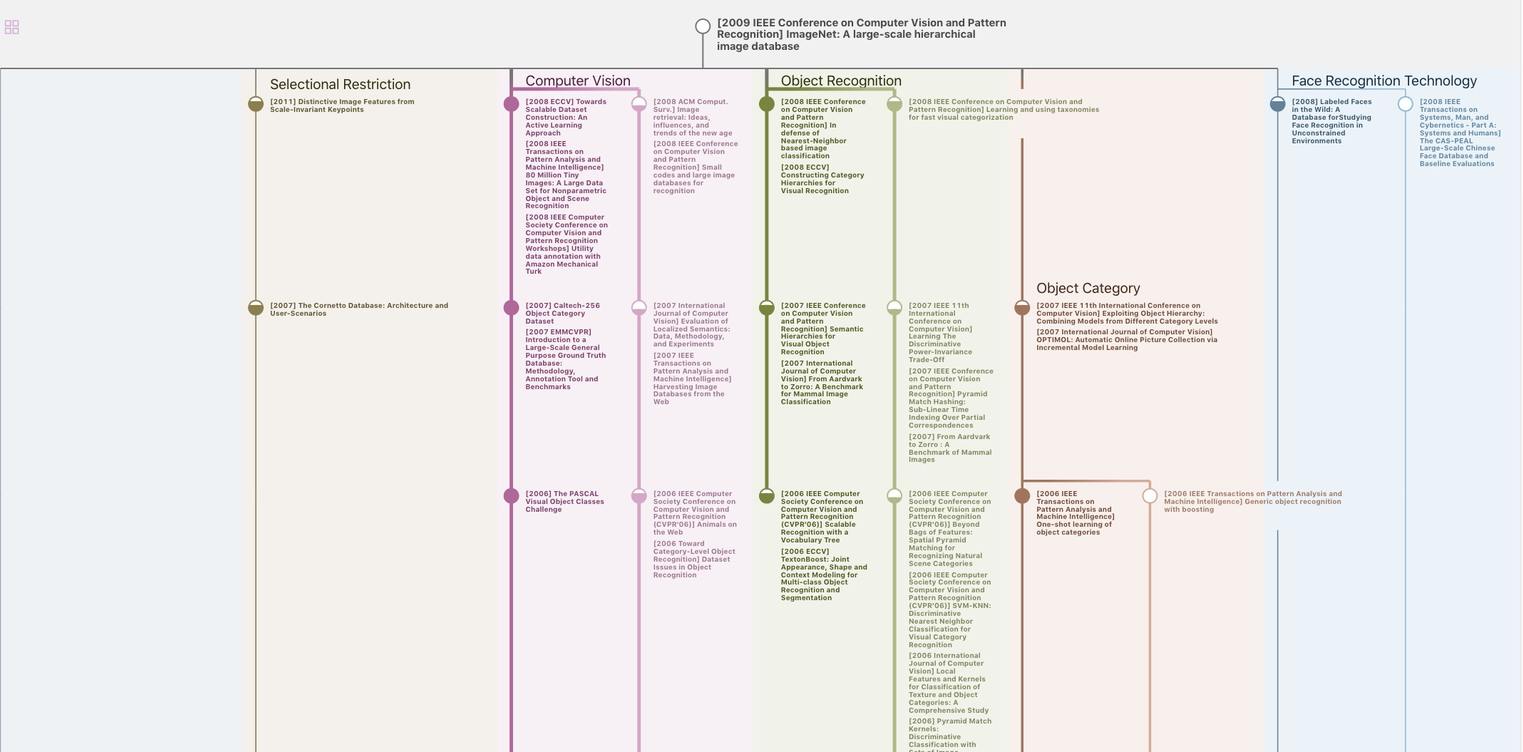
生成溯源树,研究论文发展脉络
Chat Paper
正在生成论文摘要