Disentangled Representation Learning and Residual GAN for Age-Invariant Face Verification
Pattern Recognition(2020)
摘要
•We propose a novel model called DR-RGAN to specifically address the AIFV task. DR-RGAN enjoys the strengths of both GAN model, which enhances the synthesis quality, and deep residual learning, which enables more expressive face representation learning.•Through a residual encoder-decoder structured generator, the age-invariant identity representation learning is explicitly disentangled from the age variation, so as to avoid the influence of age changes on face verification.•A multi-task discriminator is proposed to not only distinguish between real vs fake faces, but also estimate the identity and age attributes of the face. With such a design, DR-RGAN gains better face aging accuracy and stronger face representation learning ability.•Extensive quantitative and qualitative experiments on two famous large-scale datasets, CACD and LFW, clearly show that our DR-RGAN model generates pleasing aging images and achieves a high accuracy of face verification.
更多查看译文
关键词
Face recognition,Age-invariant face verification,Representation learning,Generative adversarial network
AI 理解论文
溯源树
样例
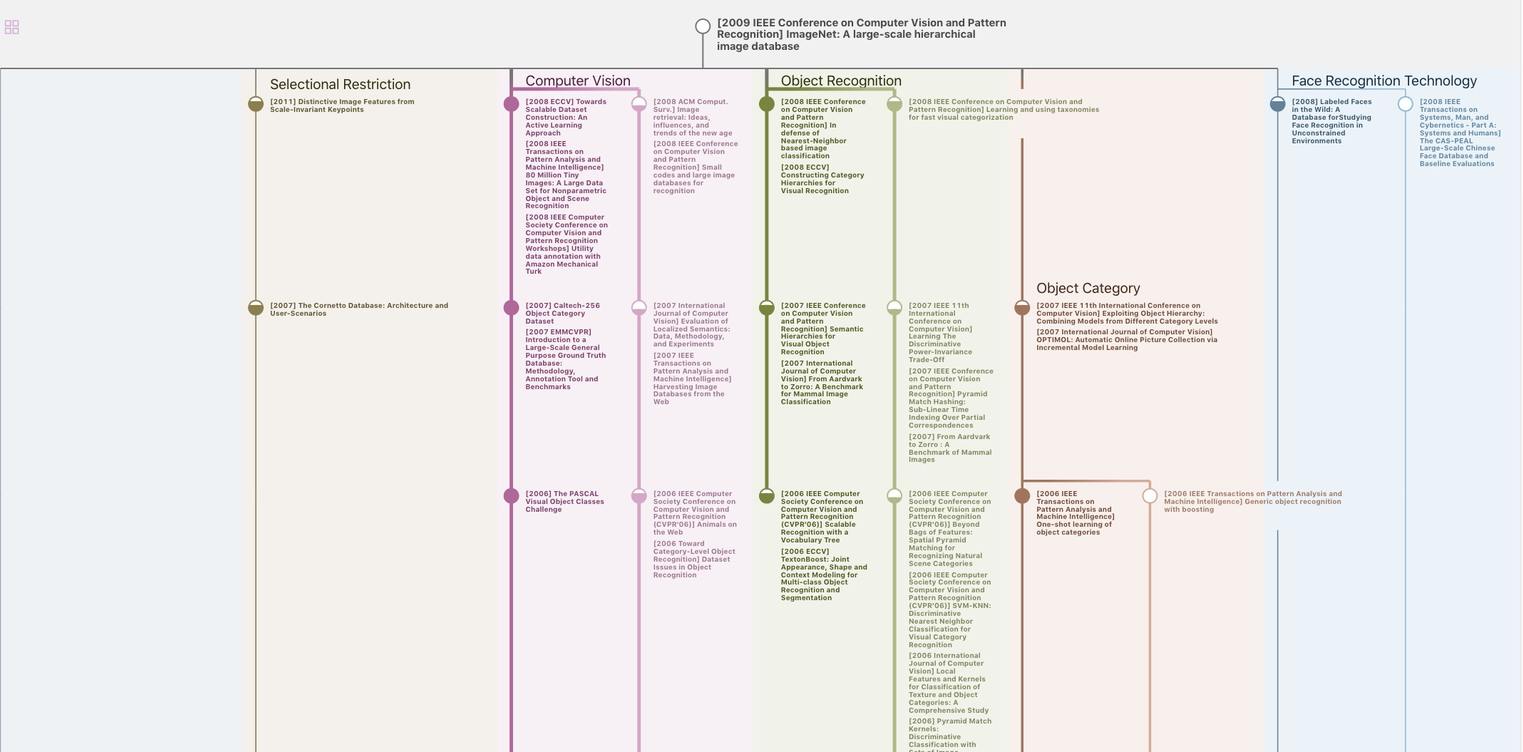
生成溯源树,研究论文发展脉络
Chat Paper
正在生成论文摘要