Energy reconstruction for a hadronic calorimeter using multivariate data analysis methods
JOURNAL OF INSTRUMENTATION(2019)
摘要
The CALICE highly granular Semi-Digital Hadronic CALorimeter (SDHCAL) technological prototype provides rich information on the shape and structure of the hadronic showers. To exploit this information and to improve on the standard energy reconstruction method where only the total number of hits is used, we propose to use two methods based on MultiVariate data Analysis (MVA) techniques: the Multi-Layer Perceptron (MLP) and the Boosted Decision Trees with Gradient Boost (BDTG). The two new methods achieve better energy linearity (Delta E/E-beam <= 2%) with respect to the classic method (Delta E/E-beam <= 5%) and improve on the relative energy resolution. For instance, the MLP method achieves 6-7% relative improvement on the whole energy range when applied on samples of simulated pi(-) events in the SDHCAL.
更多查看译文
关键词
Calorimeter methods,Calorimeters,Data Handling
AI 理解论文
溯源树
样例
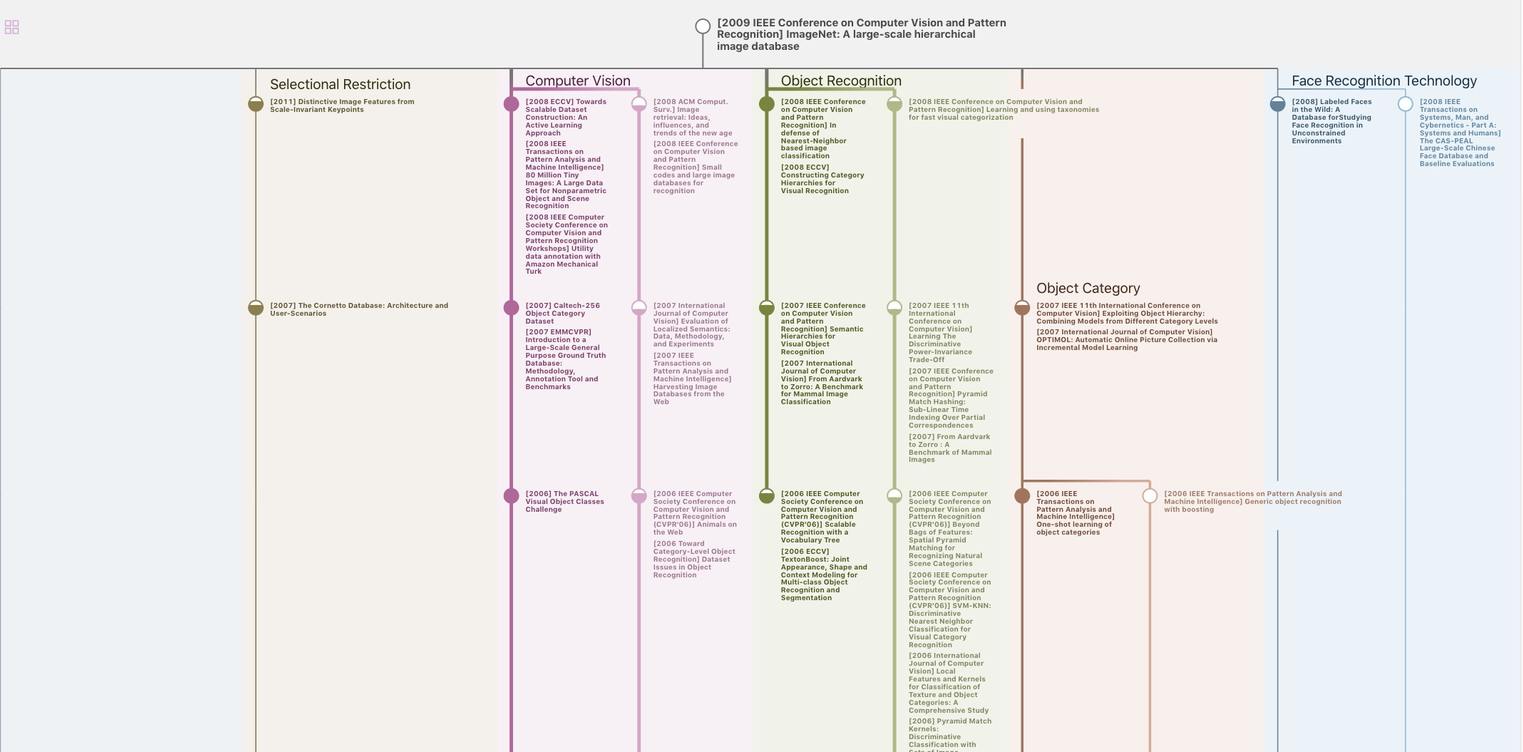
生成溯源树,研究论文发展脉络
Chat Paper
正在生成论文摘要