Adaptive Activation Functions Using Fractional Calculus
2019 IEEE/CVF INTERNATIONAL CONFERENCE ON COMPUTER VISION WORKSHOPS (ICCVW)(2019)
摘要
We introduce a generalization methodology for the automatic selection of the activation functions inside a neural network, taking advantage of concepts defined in fractional calculus. This methodology enables the neural network to define and optimize its own activation functions during the training process, by defining the fractional order of the derivative of a given primitive activation function, tuned as an additional training hyper-parameter By following this approach, the neurons inside the network can adjust their activation functions, e.g. from MLP to RBF networks, to best fit the input data, and reduce the output error. The result show the benefits of using this technique implemented on a ResNet18 topology by outperforming the accuracy of a ResNet100 trained with CIFAR10 reported in the literature.
更多查看译文
关键词
Activation functions,fractional calculus
AI 理解论文
溯源树
样例
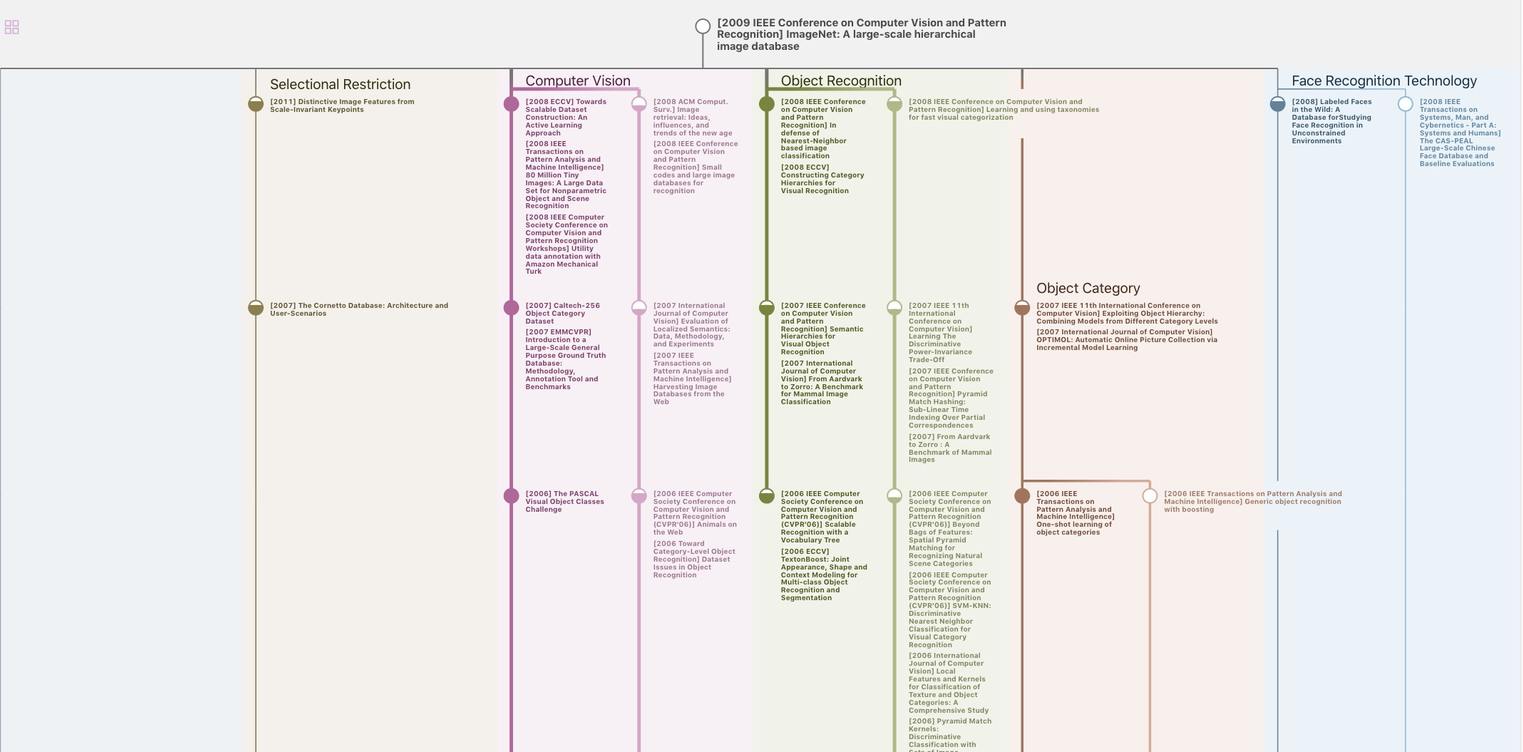
生成溯源树,研究论文发展脉络
Chat Paper
正在生成论文摘要