An Improved Deep Network For Intelligent Diagnosis Of Machinery Faults With Similar Features
IEEJ TRANSACTIONS ON ELECTRICAL AND ELECTRONIC ENGINEERING(2019)
摘要
Currently, fault diagnosis, especially single fault diagnosis, has yielded fruitful research results. However, for concurrent faults, which exist more widely in real industrial systems. Due to the challenges of complex model mechanism, single and coupled monitoring data, as well as difficulty distinguished similar features, causing the existing research findings on concurrent faults have not yet achieved satisfactory effects. Considering the problem and in order to extract features automatically while enhancing the diagnostic accuracy, an improved sparse autoencoder (SAE) network for intelligent diagnosis is proposed. Specifically, the main contents are as follows: firstly, the original time-domain signals are preprocessed to eliminate the noise disturbance. The processed signals are then adopted as the input of diagnostic network. Secondly, an initial weight constraint and an adaptive loss function are constructed to improve the diversity of SAE feature learning. Thirdly, limited-memory quasi-newton algorithm (L-BFGS) algorithm is used to solve the optimal solution and the machinery health condition identification is achieved by softmax classifier. Finally, the verification experiments based on the monitoring data obtained from different machinery are conducted. The results demonstrate the effectiveness and practicability of the proposed method. (c) 2019 Institute of Electrical Engineers of Japan. Published by John Wiley & Sons, Inc.
更多查看译文
关键词
deep learning, sparse autoencoder, concurrent faults, similar features, fault diagnosis
AI 理解论文
溯源树
样例
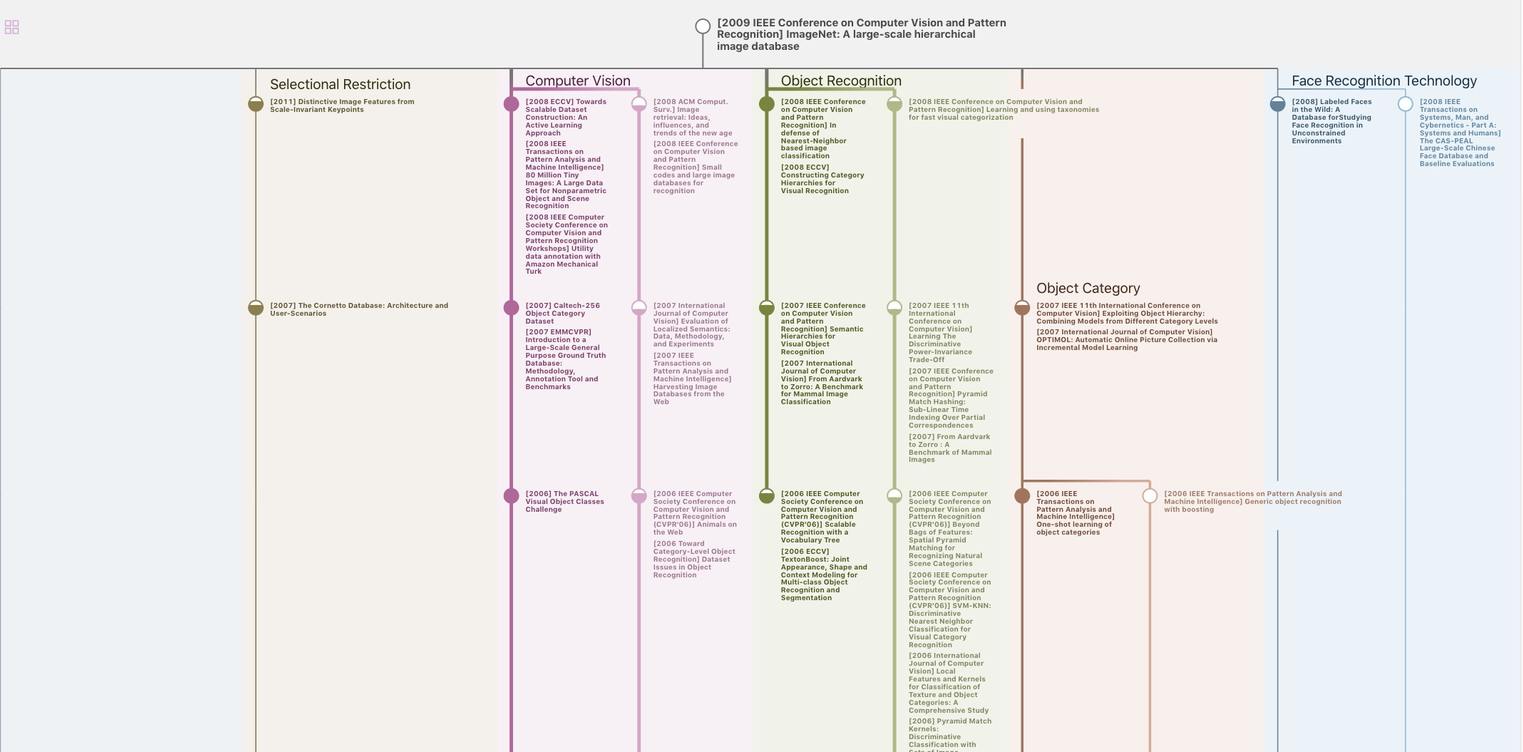
生成溯源树,研究论文发展脉络
Chat Paper
正在生成论文摘要