A predictive analytics framework as a countermeasure for attrition of students
INTERACTIVE LEARNING ENVIRONMENTS(2022)
摘要
Attrition is one of the main concerns in distance learning due to the impact on the incomes and institutions reputation. Timely identification of students at risk has high practical value in effective students' retention services. Big Data mining and machine learning methods are applied to manipulate, analyze, and predict students' failure, supporting self-directed learning. Despite the extensive application of data mining to education, the imbalance problem in minority classes of students' attrition is often overlooked in conventional models. This document proposes a large data frame using the Hadoop ecosystem and the application of machine learning techniques to different datasets of an academic year at the Hellenic Open University. Datasets were divided into 35 weeks; 32 classifiers were created, compared and statistically analyzed to address the minority classes' imbalance of student's failure. The algorithms MetaCost-SMO and C4.5 provide the most accurate performance for each target class. Early predictions of timeframes determine a remarkable performance, while the importance of written assignments and specific quizzes is noticeable. The models' performance in any week is exploited by developing a prediction tool for student attrition, contributing to timely and personalized intervention.
更多查看译文
关键词
Educational data mining, big data, machine learning, distance learning, learning analytics
AI 理解论文
溯源树
样例
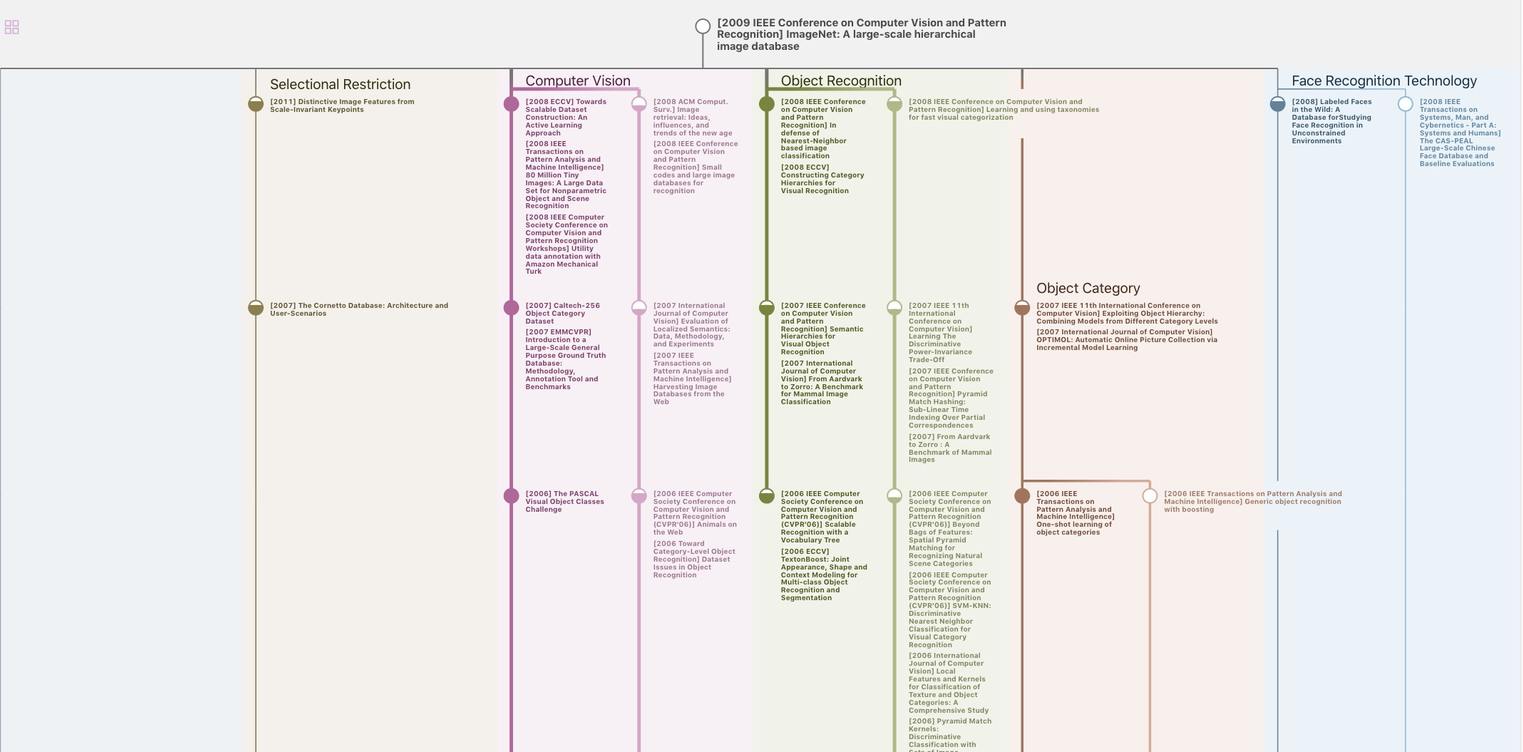
生成溯源树,研究论文发展脉络
Chat Paper
正在生成论文摘要