Effective Use of Low-level Features for Image Super-Resolution
PROCEEDINGS OF THE 38TH CHINESE CONTROL CONFERENCE (CCC)(2019)
摘要
In this paper we present an improved method based on Super-Resolution Convolutional Neural Network(SRCNN) to explore the application of lightweight CNN structure in single-image super-resolution. We keep the three layer depth unchanged and add a skip connection from input to the output and add twice skip connections across the second convolutional layer. This method can better transfer low-level features to higher layers to improve the reconstruction. In addition, we also study the effect of dataset augmentation to results. The simulation shows that the proposed method is easy to converge during training and has better image reconstruction quality.
更多查看译文
关键词
super-resolution, convolutional neural networks, skip connections
AI 理解论文
溯源树
样例
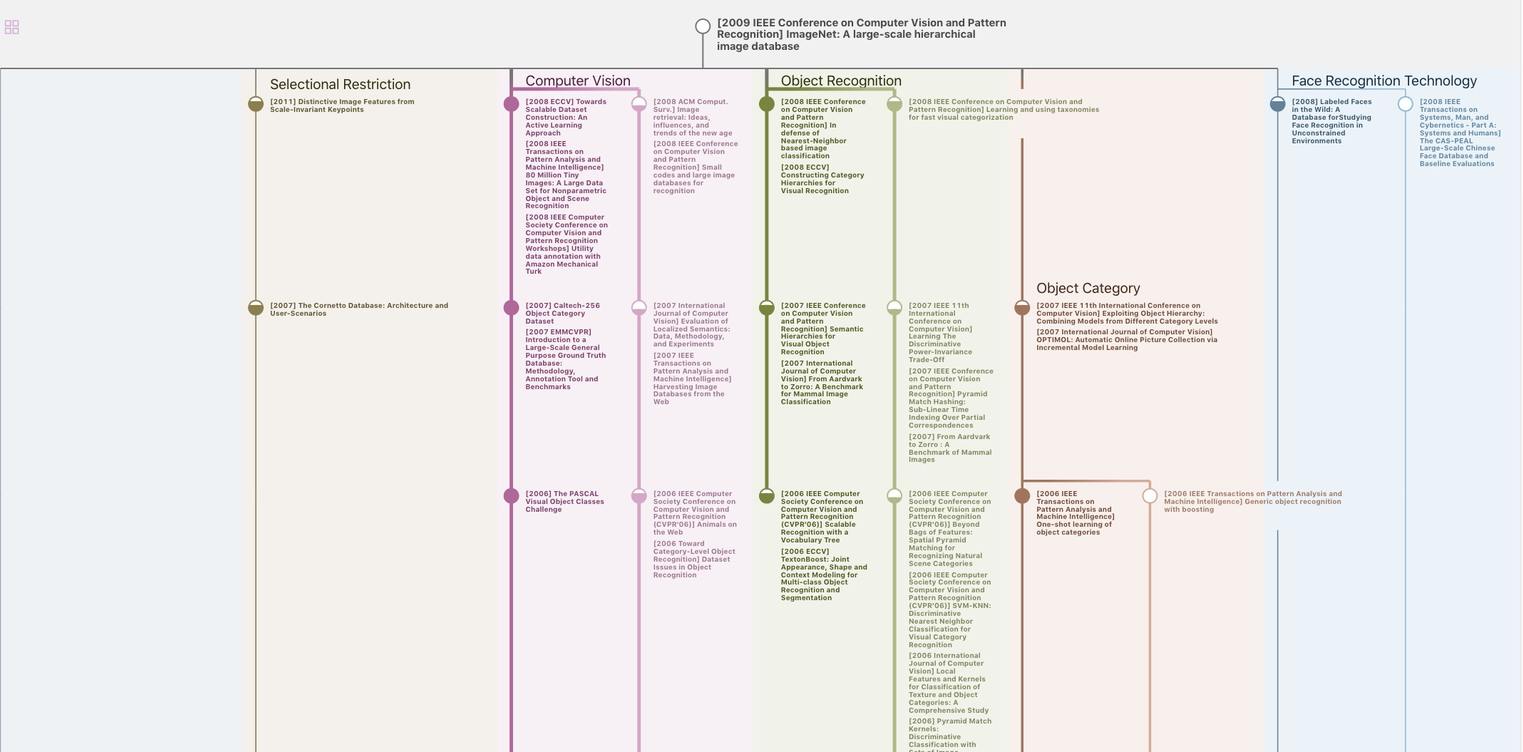
生成溯源树,研究论文发展脉络
Chat Paper
正在生成论文摘要