IDENTIFICATION OF FAST CONVERTERS AMONG MCI PATIENTS BY INTEGRATING INCOMPLETE MULTI-MODALITY IMAGE DATA USING A LONGITUDINAL TRANSFER LEARNING MODEL
Alzheimers & Dementia(2019)
摘要
Mild cognitive impairment (MCI) is a clinical stage where patients show noticeable cognitive deficit that is not severe enough to merit the designation of dementia. Identification of MCI patients who will convert to AD dementia in short term, i.e., fast converters, and enrolling them to clinical trials has important value for reducing the time and cost of the trials. To identify fast converters, one can build a machine learning classifier for individuals with MCI by integrating images of different but complementary modalities together with other clinical variables. The challenge is that multi-modality images are not universally available for all patients due to accessibility constraints (cost, insurance coverage, etc). In this study, we developed a longitudinal transfer learning (TL) model capable of training a classifier using data that contains incomplete multi-modality images collected longitudinally. 214 MCI patients from ADNI were included. 26 converted to AD within two years. We downloaded MRI, FDG-PET, and amyloid-PET images at baseline and a follow up. The patients were divided into four sub-cohorts: MRI only; MRI & FDG-PET; MRI & amyloid-PET; all three modalities available. We extracted commonly used imaging features including 3, 5, and 6 features from MRI, FDG-PET, and amyloid-PET, respectively. Clinical variables such as age, APOE 4 status, MMSE and CDR were also included. We applied longitudinal TL to integrate the incomplete multi-modality image features at baseline and the follow up and clinical variables to build a classifier for differentiating converters from non-converters. Longitudinal TL achieved 0.89 cross-validated AUC, 0.86 sensitivity, and 0.94 specificity. As a competing method, separate modeling of each sub-cohort by logistic regression achieved 0.79 AUC, 0.43 sensitivity, and 0.95 specificity. The low sensitivity was due to heavy imbalance of the training data. TL was robust to the imbalance and achieved significantly higher sensitivity (p<0.001) and AUC (p<0.001). We proposed a novel longitudinal TL model to identify fast converters of MCI patients with varying availability of imaging modalities. This capability benefited a broader patient population especially those susceptible to resource or accessibility constraints.
更多查看译文
关键词
longitudinal transfer learning model,mci patients,transfer learning,fast converters,multi-modality
AI 理解论文
溯源树
样例
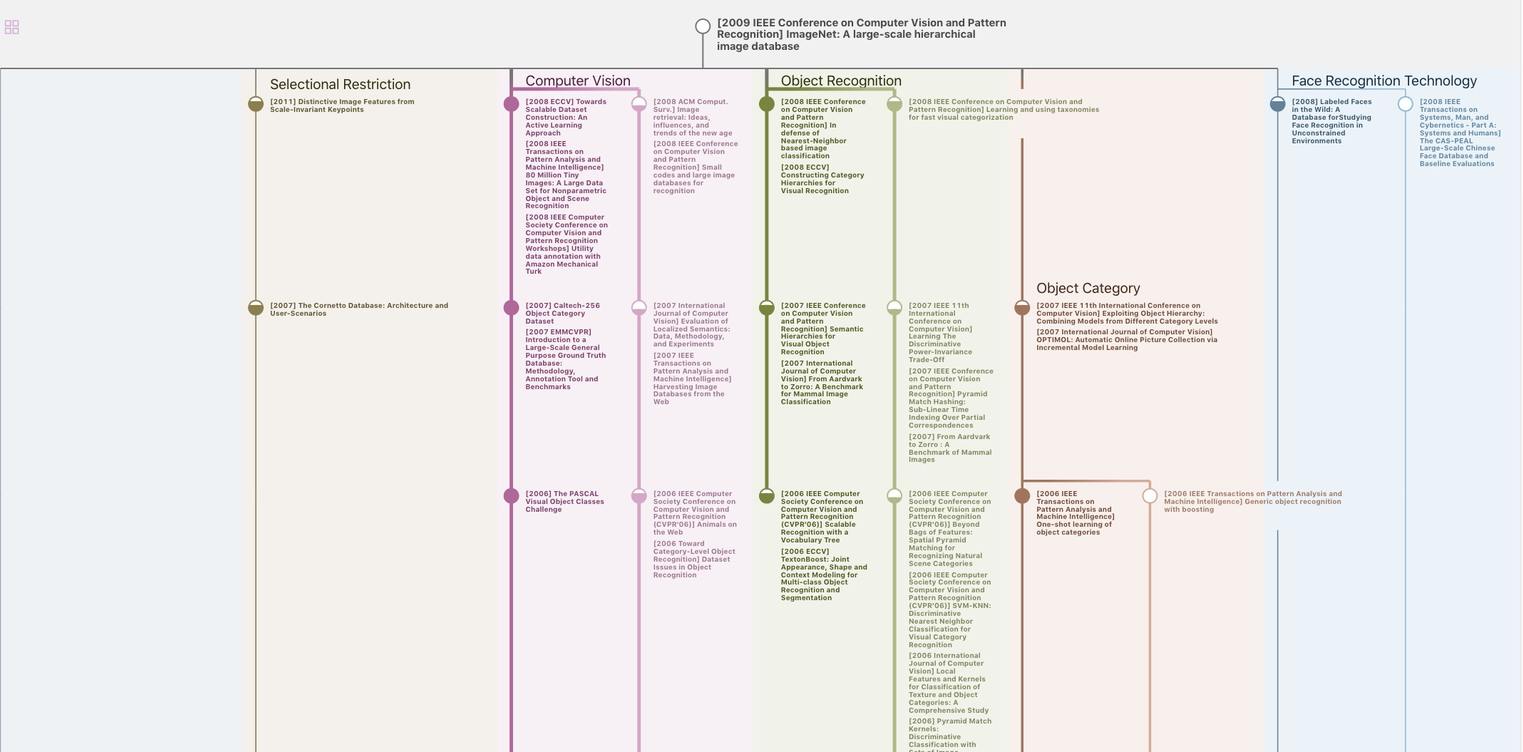
生成溯源树,研究论文发展脉络
Chat Paper
正在生成论文摘要