A novel approach for learning label correlation with application to feature selection of multi-label data
Information Sciences(2020)
摘要
Generally, every label has its own indispensable features, and it is reasonable to assume higher repetitiveness of indispensable features represents higher correlations among labels. Inspired by discernibility matrix and granular computing, this paper draws conclusions as follows:•Essential elements for any label are defined and characterized to reflect the internalconnection between features and label.•Label correlation and relevance judgement matrix for label set are described considering overlap of essential element sets.•Labels with strong correlation are assigned to a relevant label group.•The compact subset of indispensable features for any relevant label group is effectively captured from original feature set.•A novel multi-label learning method, called CLSF-MK, is proposed to increase effectiveness and practicability of prediction.
更多查看译文
关键词
Multi-label learning,Global label correlation,Local label correlation,Feature selection
AI 理解论文
溯源树
样例
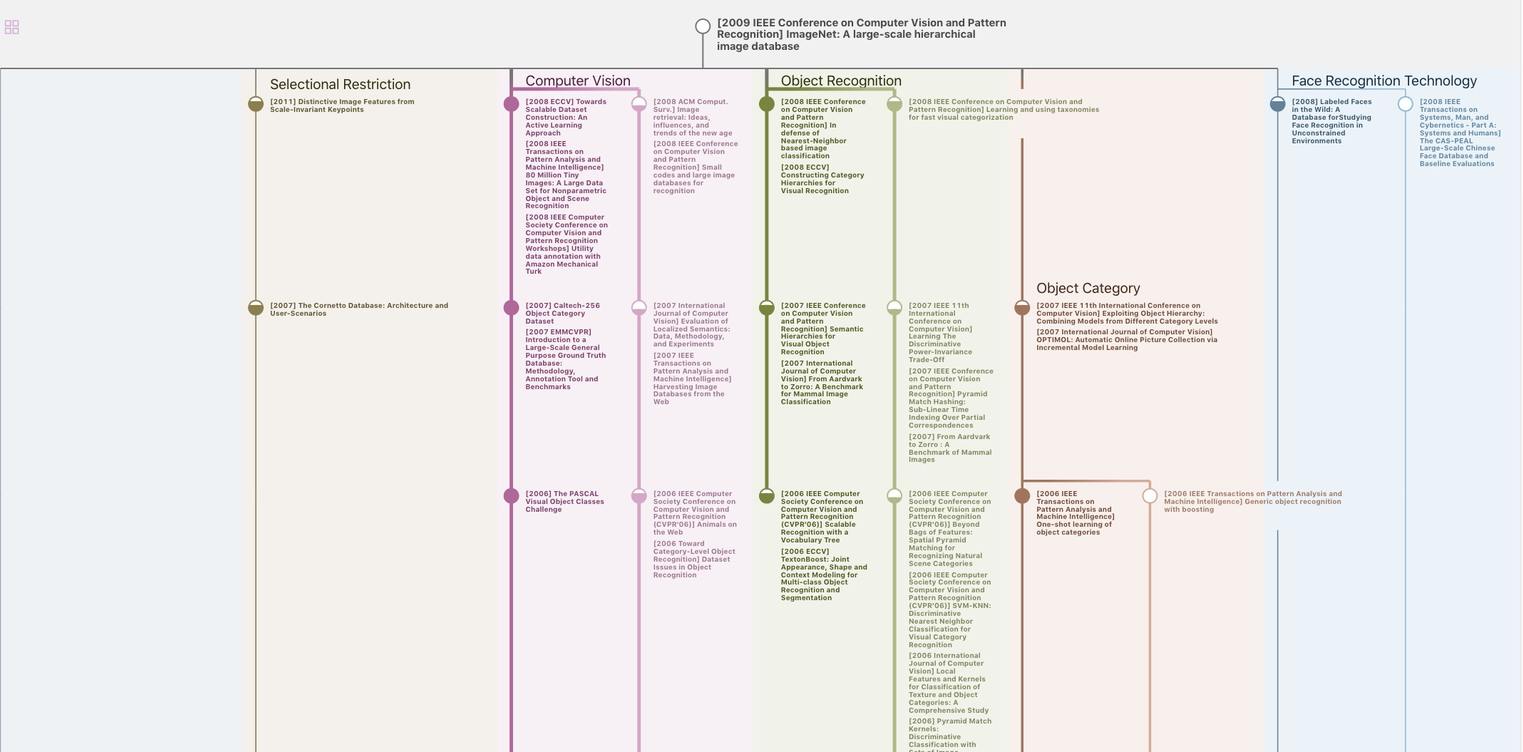
生成溯源树,研究论文发展脉络
Chat Paper
正在生成论文摘要