Establish a predictive model for high-risk de novo metastatic prostate cancer patients by machine learning.
Journal of Global Oncology(2019)
摘要
13 Background: For de novo metastatic prostate cancer (mPC)) patients, their prognosis may be really different. Some of these patients response very well to hormone therapy with durable survival, but others may be not. For those poor prognosis patients, if we could predict them as high risk patients when diagnosed, and provide aggressive upfront chemotherapy or novel hormonal therapy, they might get better treatment outcomes. Methods: We used data of prostate cancer patients from 2000 to 2016 in Chang Gung Research Database. There are 799 de novo mPC patients with castration. We predicted the possibility for these patients progressed to metastatic castration-resistant prostate cancer (mCRPC) in 1 year and find the high risk group patients. Then we figured out the best features for prediction from the best classifier with Recursive Feature Elimination. Results: The de nove mPC patients who pregressed to mCRPC in 1 year, whose mOS is 21.9 months is worse than who progressed to mCRPC beyond 1 year significantly, whose mOS is 80.7 months. (adjusted hazard ratio[aHR]: 6.43, P<0.001). The overall performance of machine learning by XGBoost is the best in all predictive models for high risk patients. (AUC=0.7000, Accuracy=0.7143). We excluded the features with missing data over 50%, then put all other features in the model. (AUC=0.7042, Accuracy=0.7239). But we got the best performance with only 11 features, including age, time from diagnosis to castration, nadir PSA, hemoglobin, eosinophil/white blood cell ratio, alkaline phosphatase, alanine transaminase, blood urea nitrogen, creatinine, prothrombin time, and secondary primary cancer, by Recursive Feature Elimination. (AUC=0.7131, Accuracy=0.7267). Conclusions: We found the predictive model has better predictive accuracy and shorter manuscript time with less features selected by Recursive Feature Elimination.We can predict high risk group in de novo mPC patients and make better clinical decision for treatment with this XGBoost model.
更多查看译文
AI 理解论文
溯源树
样例
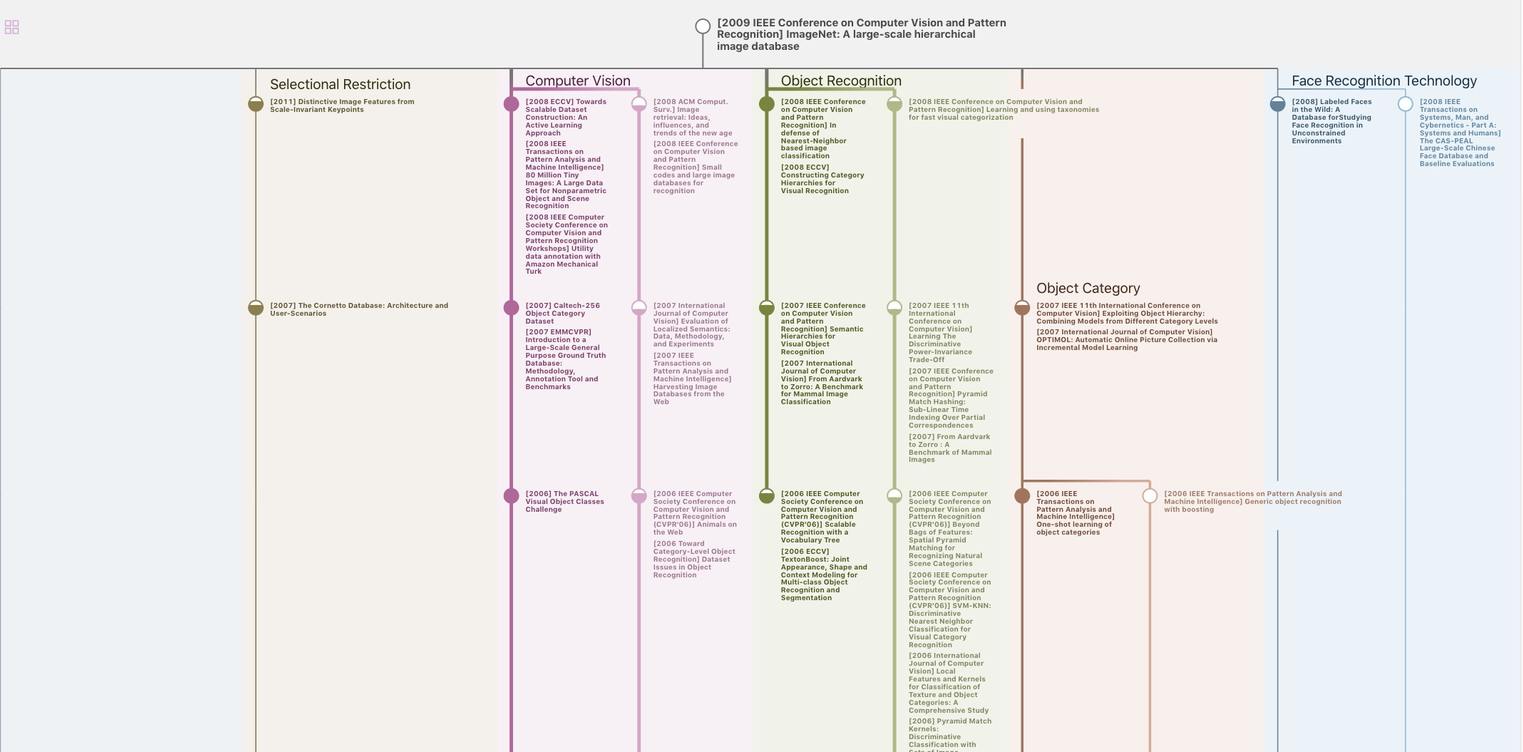
生成溯源树,研究论文发展脉络
Chat Paper
正在生成论文摘要