Hyperplane Division in Fuzzy C-Means: Clustering Big Data
IEEE Transactions on Fuzzy Systems(2020)
摘要
Big data with a large number of observations (samples) have posed genuine challenges for fuzzy clustering algorithms and fuzzy C-means (FCM), in particular. In this article, we propose an original algorithm referred to as a hyperplane division method to split the entire data set into disjoint subsets. By disjoint subsets, we mean that the data subspaces (parts of the entire data space), each of which is supported or spanned by the data points in the corresponding subset, do not overlap each other. The disjoint subsets turned out to be beneficial to the improvement of the quality of the clusters formed by the clustering algorithms. Moreover, considering that either a large number (say, thousands) or a small number (say, a few) of clusters may be pursued in the clustering task, we propose corresponding strategies (based on the hyperplane division method) to make clustering processes feasible, efficient, and effective. By validating the proposed strategies on both synthetic and publicly available data, we show their superiority (in terms of both efficiency and effectiveness) manifested in a visible way over the method of clustering the entire data and over some representative big data clustering methods.
更多查看译文
关键词
Big data clustering,clustering requirements,fuzzy C-means (FCM),hyperplane division,many clusters
AI 理解论文
溯源树
样例
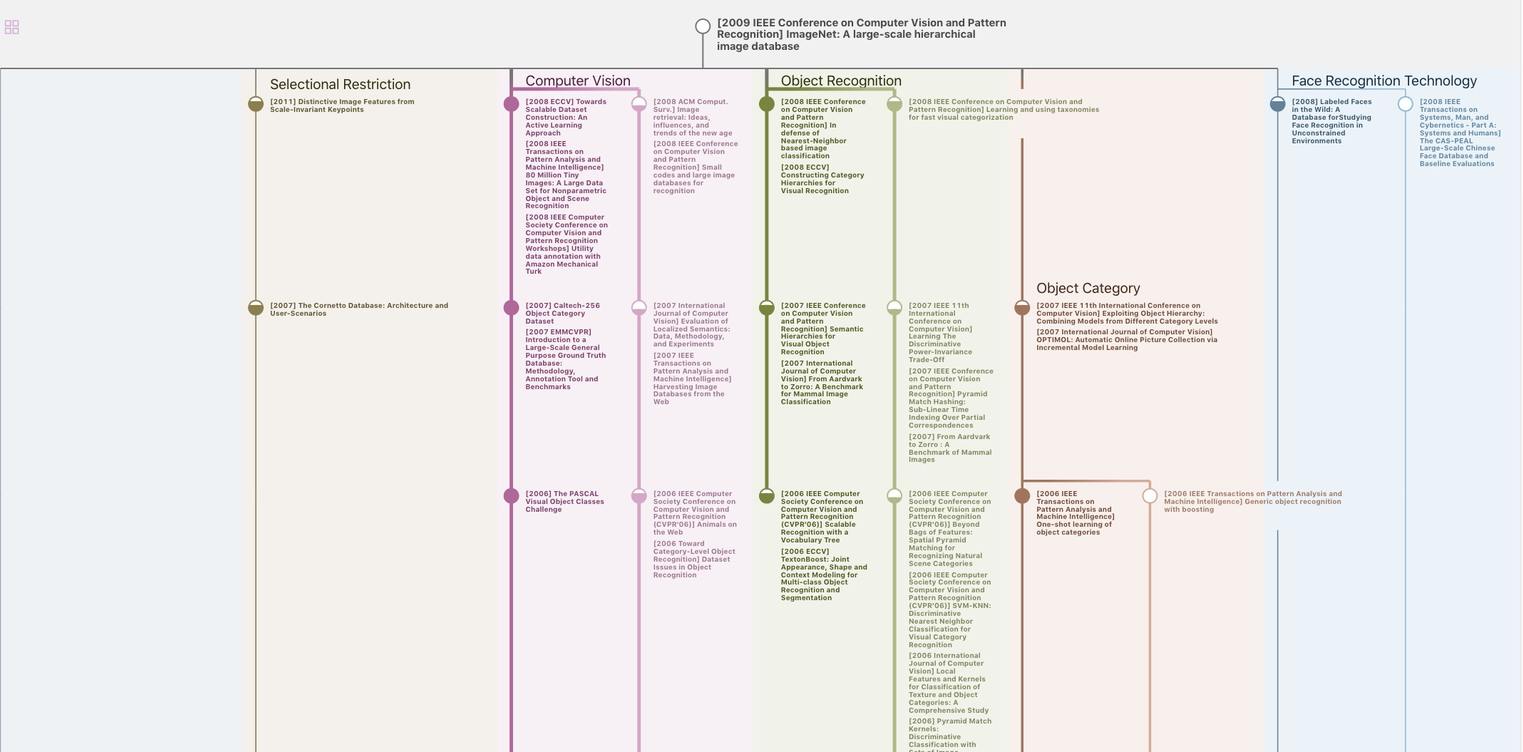
生成溯源树,研究论文发展脉络
Chat Paper
正在生成论文摘要