Novel DDoS Feature Representation Model Combining Deep Belief Network and Canonical Correlation Analysis
CMC-COMPUTERS MATERIALS & CONTINUA(2019)
摘要
Distributed denial of service (DDoS) attacks launch more and more frequently and are more destructive. Feature representation as an important part of DDoS defense technology directly affects the efficiency of defense. Most DDoS feature extraction methods cannot fully utilize the information of the original data, resulting in the extracted features losing useful features. In this paper, a DDoS feature representation method based on deep belief network (DBN) is proposed. We quantify the original data by the size of the network flows, the distribution of IP addresses and ports, and the diversity of packet sizes of different protocols and train the DBN in an unsupervised manner by these quantified values. Two feedforward neural networks (FFNN) are initialized by the trained deep belief network, and one of the feedforward neural networks continues to be trained in a supervised manner. The canonical correlation analysis (CCA) method is used to fuse the features extracted by two feedforward neural networks per layer. Experiments show that compared with other methods, the proposed method can extract better features.
更多查看译文
关键词
Deep belief network,DDoS feature representation,canonical correlation analysis
AI 理解论文
溯源树
样例
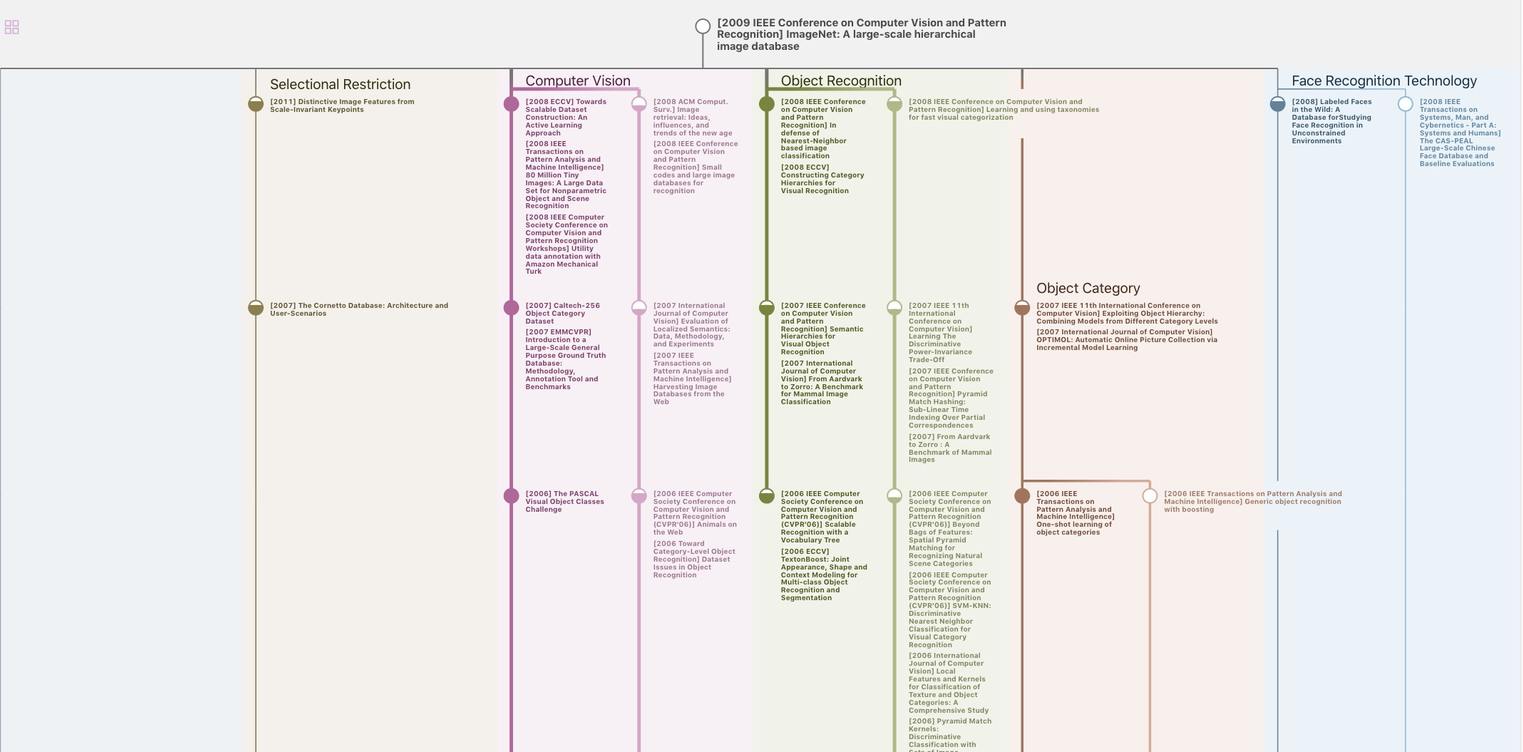
生成溯源树,研究论文发展脉络
Chat Paper
正在生成论文摘要