A novel learning adaptive hysteresis inverse compensator for pneumatic artificial muscles
SMART MATERIALS AND STRUCTURES(2020)
摘要
Because of friction and elastic deformation, a pneumatic artificial muscle (PAM) has strong asymmetric hysteresis which negatively affects the driving performance. The hysteresis is generally described by a phenomenological model. However, the phenomenological hysteresis model always only depicts the hysteresis under certain conditions, so the compensator based on it has poor universality for different external conditions. In this article, we proposed a guided reinforcement learning (GRL) algorithm to online adjust the output of a Kriging-median inverse hysteresis compensator (KMIC), so that improve the compensation performance of the compensator for PAMs under different size, external load, and input signal frequency conditions. We name the novel compensator as a guided reinforcement learning Kriging- median inverse compensator (GRL-KMIC). Both simulation and experiment platform are set up to verify the effectiveness of the proposed compensator. The results show that the GRL algorithm improves the universality of KMIC obviously, so GRL-KMIC always keeps better compensation control performance than KMIC for different external conditions.
更多查看译文
关键词
Pneumatic artificial muscle (PAM),asymmetric hysteresis,phenomenological hysteresis model,universality,guided reinforcement learning Kriging-median inverse compensator (GRL-KMIC)
AI 理解论文
溯源树
样例
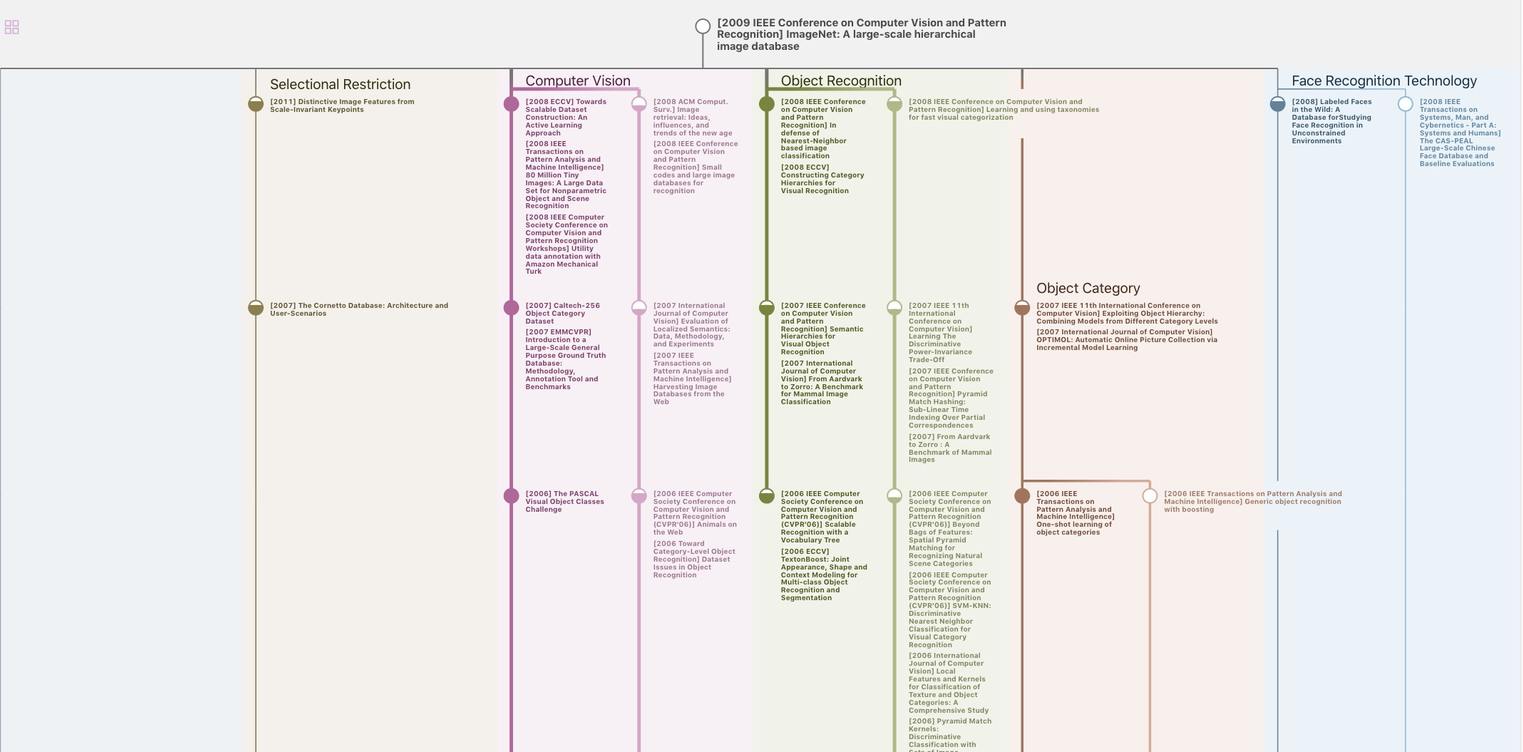
生成溯源树,研究论文发展脉络
Chat Paper
正在生成论文摘要