RNN-Based Room Scale Hand Motion Tracking
MobiCom '19: The 25th Annual International Conference on Mobile Computing and Networking Los Cabos Mexico October, 2019(2019)
摘要
Smart speakers allow users to interact with home appliances using voice commands and are becoming increasingly popular. While voice-based interface is intuitive, it is insufficient in many scenarios, such as in noisy or quiet environments, for users with language barriers, or in applications that require continuous motion tracking. Motion-based control is attractive and complementary to existing voice-based control. However, accurate and reliable room-scale motion tracking poses a significant challenge due to low SNR, interference, and varying mobility. To this end, we develop a novel recurrent neural network (RNN) based system that uses speakers and microphones to realize accurate room-scale tracking. Our system jointly estimates the propagation distance and angle-of-arrival (AoA) of signals reflected by the hand, based on AoA-distance profiles generated by 2D MUSIC. We design a series of techniques to significantly enhance the profile quality under low SNR. We feed the profiles in a recent history to our RNN to estimate the distance and AoA. In this way, we can exploit the temporal structure among consecutive profiles to remove the impact of noise, interference and mobility. Using extensive evaluation, we show our system achieves 1.2--3.7~cm error within 4.5~m range, supports tracking multiple users, and is robust against ambient sound. To our knowledge, this is the first acoustic device-free room-scale tracking system.
更多查看译文
关键词
Acoustic motion tracking,recurrent neural network,MUSIC
AI 理解论文
溯源树
样例
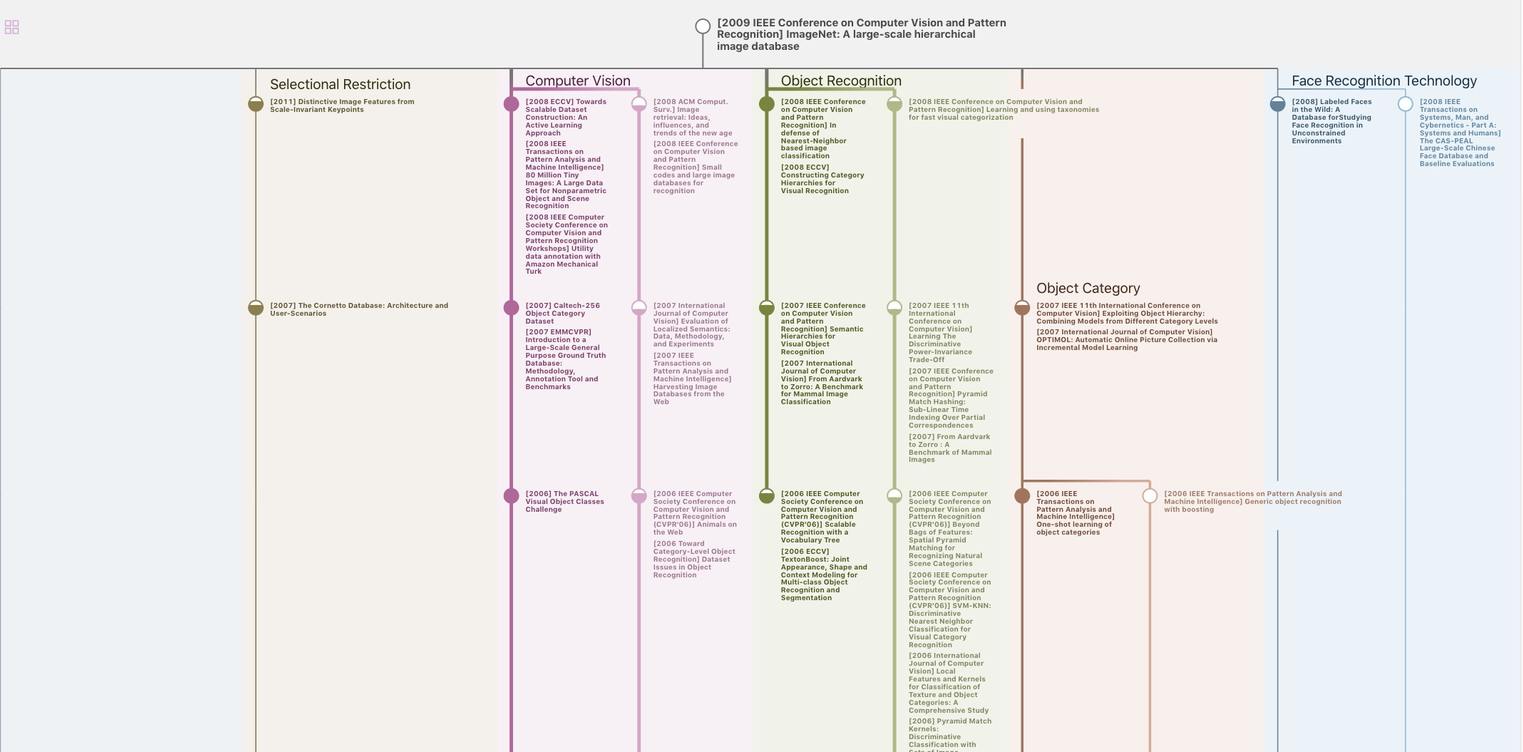
生成溯源树,研究论文发展脉络
Chat Paper
正在生成论文摘要