Jointly Detecting And Extracting Social Events From Twitter Using Gated Bilstm-Crf
IEEE ACCESS(2019)
摘要
In this article, we focus on the problem of social event extraction from Twitter, in which event detection, i.e., to identify which messages truly mention events of interest, is an indispensable step due to the fact that most Twitter messages, viz. tweets, are not related to any real-world event. Existing approaches to this problem often use pipelined architectures relying on some hand-crafted features derived using off-the-shelf natural language processing (NLP) tools, which may cause error propagation from the upstream component (event detection) to the downstream one (element extraction) and fail to leverage the interdependencies between them. To overcome these limitations, we propose a deep neural network based framework to Jointly Detect and Extract Events from Twitter (JDEET), which learns, as well as conducts, detection and extraction simultaneously by defining a joint loss function, a bidirectional long short-term memory (LSTM) based common representation layer, and a control gate. A conditional random field (CRF) layer is further employed to capture the strong dependencies among output labels. Experimental results show that the proposed approach outperforms the state-of-the-art ones considerably on a real-world dataset from Twitter.
更多查看译文
关键词
Event extraction, social events, Twitter, joint models, a control gate, deep neural networks
AI 理解论文
溯源树
样例
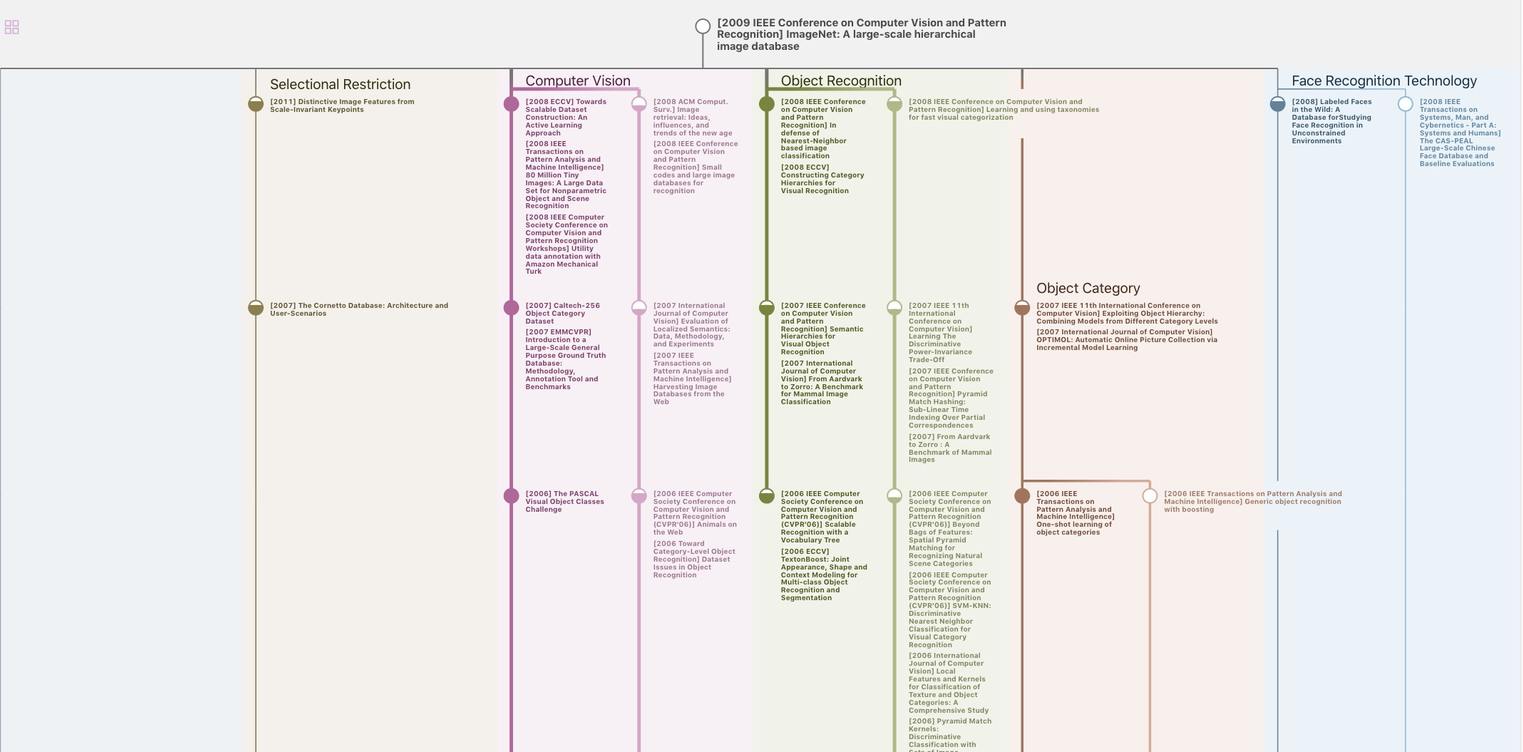
生成溯源树,研究论文发展脉络
Chat Paper
正在生成论文摘要