ARS-Net: Adaptively Rectified Supervision Network for Automated 3D Ultrasound Image Segmentation
Lecture Notes in Computer Science(2019)
摘要
3D Ultrasound (3DUS) has been widely used in clinical diagnosis. However, volume segmentation of 3DUS is very challenging due to relatively poor image quality and usually small datasets. We propose an efficient and robust method (ARS-Net) for single organ segmentation in 3DUS. Our contributions are twofold. (i) We propose a 2.5D framework based on a 2D segmentation network with 2.5D input which can provide more contextual and spatial information. The proposed framework also overcomes the limitation of 3D networks, such as small datasets, lack of pre-trained models and high memory cost. (ii) To further enhance the performance in low signal-to-noise ratio (SNR) regions, we incorporate a new mechanism of adaptively rectified supervision (ARS) into the proposed 2.5D framework at training stage. Specifically, both pixel-wise reweighted dice loss and image-wise shape regularization loss are applied to improve the sensitivity and the specificity of segmentation. The experiment results on two representative and challenging datasets of 3DUS show that the proposed ARS-Net outperforms state-of-the-art methods with higher accuracy but lower complexity. The proposed novel network is robust to small datasets and can provide an accurate and fast volume segmentation tool for 3DUS.
更多查看译文
关键词
3D Ultrasound,2.5D segmentation,Adaptively rectified supervision,Pixel-wise reweighted dice,Image-wise shape regularization
AI 理解论文
溯源树
样例
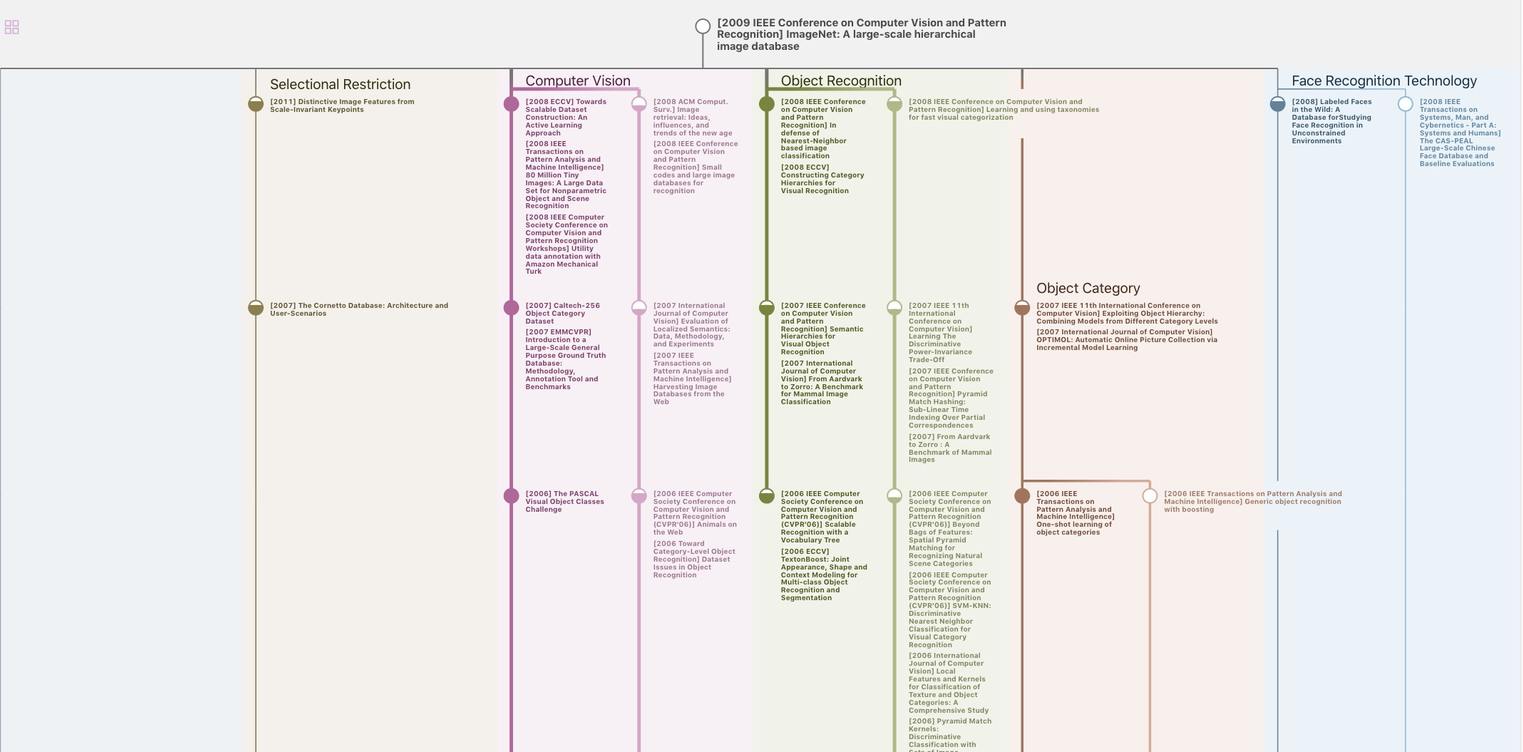
生成溯源树,研究论文发展脉络
Chat Paper
正在生成论文摘要