Skyline-based dissimilarity of images
Journal of Intelligent Information Systems(2019)
摘要
Large image collections are being used in many modern applications. In this paper, we aim at capturing the intrinsic dissimilarities of image descriptors in large image collections, i.e., to detect dissimilar (or else diverse) images without defining an explicit similarity or distance measure. Towards this goal, we adopt skyline processing techniques for large image databases, based on their high-dimensional descriptor vectors. The novelty of the proposed methodology lies in the use of skyline techniques empowered by state-of-the-art hashing schemes to enable effective data partitioning and indexing in secondary memory, towards supporting large image databases. The proposed approach is evaluated experimentally by using three real-world image datasets. Performance evaluation results demonstrate that images lying on the skyline have significantly different characteristics, which depend on the type of the descriptor. Thus, these skyline items may be used as seeds to apply clustering in large image databases. In addition, we observe that skyline processing using hash-based indexing structures is significantly faster than index-free skyline computation and also more efficient than skyline computation with hierarchical indexing structures. Based on our results, the proposed approach is both efficient (regarding runtime) and effective (with respect to image diversity) and therefore can be used as a base for more complex data mining tasks such as clustering.
更多查看译文
关键词
Image databases, Image descriptors, Skyline algorithms, Hashing techniques
AI 理解论文
溯源树
样例
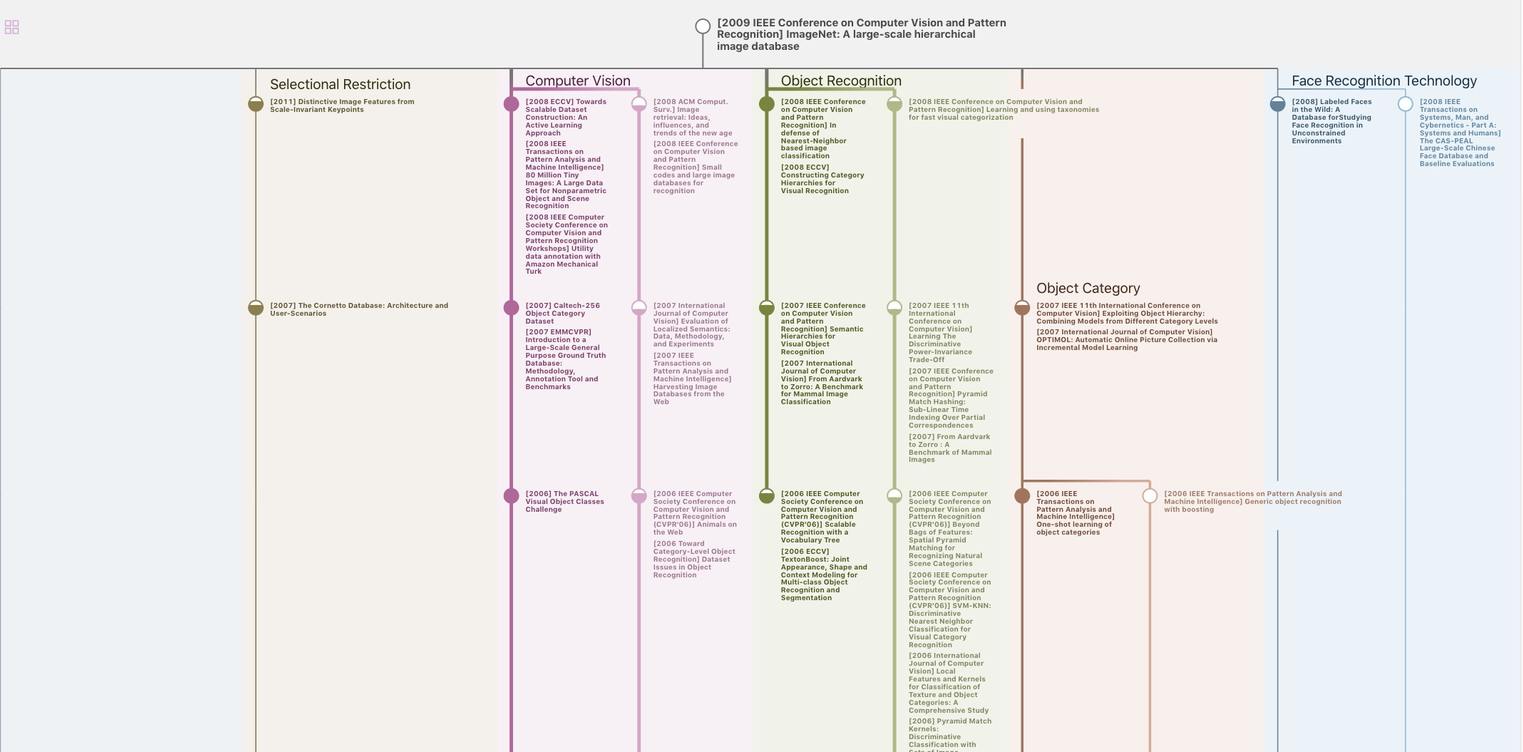
生成溯源树,研究论文发展脉络
Chat Paper
正在生成论文摘要